How to Measure Data Quality Effectively ?
Discover the essential strategies and tools for assessing data quality with precision.

Businesses rely heavily on data to make informed decisions. However, the effectiveness of these decisions is contingent upon the quality of the data available. This article delves into how organizations can measure data quality effectively through various clarity and methodologies.
Understanding the Importance of Data Quality
Data quality is a critical aspect that directly influences business outcomes. High-quality data can lead to superior business insights, while poor data quality can result in misguided decisions and lost opportunities.
In an era where organizations are saturated with large volumes of data, the emphasis on ensuring the integrity, reliability, and usability of this data cannot be overstated. Properly measured and enhanced data quality fosters a culture of data-driven decision-making. Furthermore, as businesses increasingly rely on advanced technologies such as artificial intelligence and machine learning, the need for clean and accurate data becomes even more pronounced. These technologies depend on high-quality data to function effectively, making data quality not just a best practice but a fundamental requirement for success in the digital age.
The Role of Data in Decision Making
Data plays an instrumental role across various sectors, driving decisions ranging from strategic planning to operational improvements. It aids organizations in identifying market trends, understanding customer behaviors, and optimizing processes.
With reliable data, organizations can leverage insights for competitive advantages, enhance customer experiences, and improve operational efficiencies. Thus, understanding its role solidifies the necessity for high data quality standards. Additionally, the integration of real-time data analytics allows businesses to respond swiftly to changing market conditions, ensuring they remain agile and competitive. This capability not only enhances decision-making processes but also empowers teams to innovate and adapt, fostering a proactive rather than reactive business environment.
Consequences of Poor Data Quality
The ramifications of poor data quality are significant and multifaceted. Organizations may experience financial losses due to incorrect information that leads to incorrect predictions and misguided strategies.
Moreover, operational inefficiencies, such as redundancies and increased processing times, may arise from unreliable data. There is also the potential for reputational damage if stakeholders lose trust in an organization’s operations or analytics due to flawed data. In addition to these immediate consequences, the long-term effects can be even more detrimental. For instance, a company that consistently relies on poor data may find itself at a strategic disadvantage, unable to make informed decisions that align with market realities. This can lead to a cycle of missed opportunities and stagnation, ultimately hindering growth and innovation in an increasingly competitive landscape.
Key Elements of Data Quality
To effectively measure data quality, it’s essential to focus on several key elements that embody what high-quality data should possess. Understanding these elements serves as the foundation for any data quality framework. By establishing a robust framework, organizations can not only enhance their data management practices but also foster a culture of data-driven decision-making that permeates all levels of the organization.
Accuracy
Accuracy measures whether the data is close to the true value or correct representation of the information it is supposed to convey. It involves validating data against trusted sources and ensuring that it reflects real-world conditions as closely as possible.
An accurate dataset prevents misinterpretations, thereby supporting sound decision-making processes. Organizations should implement regular checks and balances to maintain data accuracy over time. Additionally, employing automated data validation tools can significantly reduce human error, ensuring that data remains accurate as it is collected, processed, and analyzed. This proactive approach not only enhances data integrity but also builds trust among stakeholders who rely on the data for critical business decisions.
Consistency
Consistency refers to the uniformity of data across multiple datasets and platforms. For data to be considered consistent, it should not conflict with itself when accessed from various sources or at different times.
Inconsistent data can disrupt business processes and create confusion among stakeholders. Regular synchronization protocols and adherence to standardized formats can mitigate inconsistency issues. Furthermore, organizations can benefit from establishing a centralized data repository that serves as a single source of truth. This repository can help ensure that all departments are working with the same data, reducing discrepancies and fostering collaboration across teams.
Completeness
Completeness concerns whether all required data is present. This involves ensuring that no critical information is missing, which can skew results or lead to incomplete analyses.
Organizations can quantify completeness by identifying required data fields and performing audits to assess whether any data is missing or incomplete. It is crucial to address gaps promptly to maintain operational effectiveness. Additionally, implementing data entry validation rules can help capture complete information at the point of data collection, minimizing the risk of incomplete datasets from the outset. Regular training sessions for staff on the importance of data completeness can also enhance awareness and accountability in data management practices.
Relevance
The relevance of data indicates how well it meets the needs of the organization’s objectives. Data that is outdated or not aligned with current business goals can hinder decision-making.
To ensure data relevance, organizations should periodically review the datasets. This may involve discarding outdated information and including updated datasets that reflect current market conditions and operational needs. Moreover, engaging stakeholders in the review process can provide valuable insights into which data is most pertinent to their needs, ensuring that the organization remains agile and responsive to change. By fostering an environment where data relevance is continuously assessed, organizations can better align their strategies with evolving market dynamics and customer expectations.
Establishing Data Quality Metrics
Once the key elements of data quality are understood, the next step involves establishing measurable metrics that align with organizational goals. Metrics provide a tangible way to assess and improve data quality. By implementing a robust framework for data quality metrics, organizations can not only monitor their data but also foster a culture of accountability and continuous improvement.
Defining Your Data Quality Goals
Defining data quality goals is fundamental to measuring data quality effectively. These goals should be transparent, specific, measurable, and actionable, ensuring that everyone in the organization understands the purpose and importance of maintaining data quality. Involving stakeholders from various departments during this process can lead to a more comprehensive understanding of data needs and challenges, ultimately resulting in more relevant and impactful goals.
Goals might include achieving a certain percentage of accuracy across datasets, maintaining a consistency threshold, or ensuring data completeness for key business processes. Additionally, organizations may consider setting benchmarks based on industry standards or historical performance to provide context for their goals. Clear goals pave the way for targeted initiatives and improvements, enabling teams to prioritize their efforts and allocate resources effectively.
Identifying Suitable Metrics
After setting goals, organizations need to identify the right metrics to track progress. These metrics can vary widely and may include accuracy rates, timeliness of data updates, or user satisfaction scores based on data usability. It is essential to involve data stewards and analysts in this process, as their insights can help identify which metrics are most relevant and actionable for the organization.
It's crucial to tailor metrics to specific objectives and ensure they provide insights relevant to the organization’s needs. For instance, if a goal is to enhance customer experience, metrics such as response times to customer inquiries or the accuracy of customer data may be prioritized. Regular reviews of chosen metrics can help adapt to the evolving landscape of data and its implications on business decisions. Furthermore, leveraging advanced analytics and visualization tools can enhance the understanding of these metrics, making it easier for teams to interpret data quality trends and take proactive measures when issues arise.
Implementing Data Quality Measurement Tools
With established metrics in place, the next step involves implementing tools that aid in measuring and enhancing data quality. These tools provide automated solutions to track, evaluate, and improve data at scale.
Overview of Data Quality Tools
Data quality tools range from simple spreadsheet applications to sophisticated software solutions designed for advanced data governance. These tools typically feature functionalities such as data profiling, validation, cleansing, and monitoring.
Choosing the right tool depends on various factors, including the volume of data, the complexity of operations, and specific organizational needs. Understanding the strengths and limitations of available tools is key to effective implementation.
Selecting the Right Tool for Your Needs
When selecting a data quality measurement tool, organizations must consider their unique requirements. Factors such as ease of use, scalability, integration capabilities, and cost-effectiveness should guide the decision-making process.
Additionally, organizations may benefit from trials or demonstrations to ensure that the selected tool meets their expectations and effectively addresses their data quality challenges. Investing in the right tools can yield significant returns, enhancing overall data quality management efforts.
Regular Monitoring and Improvement of Data Quality
Data quality isn't a one-time effort; it demands ongoing monitoring and consistent improvement. Regularly assessing data quality ensures that organizations can adapt to changes and continuously enhance their data practices.
Setting Up a Data Quality Dashboard
A data quality dashboard is an invaluable asset that provides a real-time view of data quality metrics. It enables organizations to visualize key metrics and trends, facilitating quicker decision-making.
By establishing a centralized dashboard, stakeholders can gain insights at a glance, monitoring adherence to quality standards and identifying areas for improvement swiftly. It serves as an interactive tool for promoting awareness and accountability surrounding data quality initiatives.
Continuous Improvement of Data Quality
Continuous improvement is integral to maintaining high data quality. Organizations should cultivate a culture of ongoing assessment and refinement of their data management practices.
This may involve regular training for staff members, periodic reviews of data processes, and fostering open communication about data-related issues within teams. By adopting an attitude of continual improvement, organizations can adapt to data challenges as they come and sustain high-quality data standards.
Ready to elevate your data quality and empower your decision-making with cutting-edge technology? Look no further than CastorDoc, the comprehensive governance platform that integrates advanced cataloging, lineage capabilities, and a user-friendly AI assistant. CastorDoc is designed to enable self-service analytics, making it easier for both data teams and business users to manage, understand, and utilize data effectively. With CastorDoc, you can ensure data quality, maintain compliance, and leverage natural language interactions for seamless data discovery and utilization. Try CastorDoc today and unlock the full potential of your data to drive informed decisions across your enterprise.
You might also like
Get in Touch to Learn More
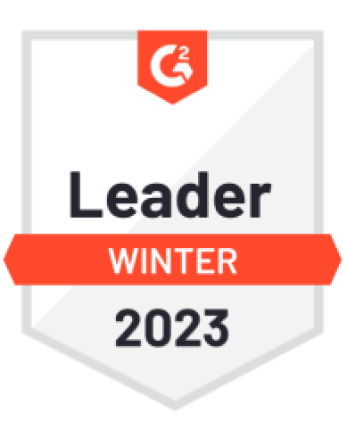
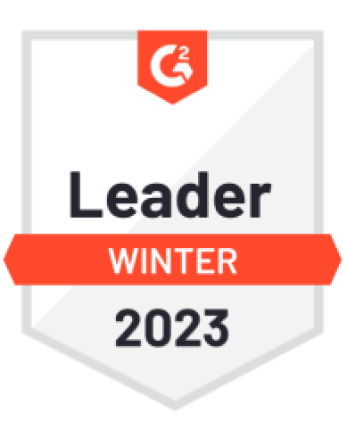

“[I like] The easy to use interface and the speed of finding the relevant assets that you're looking for in your database. I also really enjoy the score given to each table, [which] lets you prioritize the results of your queries by how often certain data is used.” - Michal P., Head of Data