Semantic Layer Management: Essential Practices for Long-Term Success
Unlock the secrets to sustainable data strategy with our guide on semantic layer management.

Understanding Semantic Layer Management
Semantic layer management refers to the processes and practices involved in overseeing the semantic layer of data architecture within an organization. This layer serves as an intermediary between complex data sources and end-users, enabling them to interact with data in a more meaningful and accessible manner. By organizing data in a way that reflects business concepts and relationships, the semantic layer acts as a bridge that translates data into understandable terms for informed decision-making.
As companies increasingly rely on data-driven insights, the management of the semantic layer becomes crucial for maximizing the value derived from data assets. It streamlines the interaction between users, analysts, and underlying data, transforming complex structures into user-friendly representations that make efficient data consumption possible.
The Basics of Semantic Layer Management
The foundation of semantic layer management lies in understanding how data can be categorized and interpreted. It encompasses a framework that standardizes data definitions, relationships, and hierarchies. This ensures uniformity across the organization, allowing users and applications to interact seamlessly with data without needing to understand its underlying complexity.
Additionally, establishing governed vocabularies and ontologies within the semantic layer plays a pivotal role. These vocabularies ensure that terms are consistently used across the organization, minimizing confusion and misinterpretation. By creating a shared language around data, organizations can foster collaboration among teams, ensuring that everyone is on the same page when it comes to data interpretation and usage.
The Role of Semantic Layer in Data Management
The semantic layer plays an integral role in modern data management strategies by offering a unified view of disparate data sources. By abstracting the complexities of raw data, it allows organizations to present data in ways that align with business requirements. This alignment facilitates better data retrieval and analysis, ultimately leading to more accurate insights.
Moreover, the semantic layer lays the groundwork for enhanced data interoperability. Different systems and applications can communicate and reconcile data effectively. This is essential in creating a cohesive data ecosystem where information flows freely between departments and platforms. For instance, marketing teams can access customer data from sales, while finance can analyze operational data, all through a common semantic framework that ensures consistency and clarity. This interconnectedness not only boosts efficiency but also empowers teams to leverage data collaboratively, driving innovation and strategic initiatives across the organization.
Key Components of Semantic Layer Management
A comprehensive approach to semantic layer management includes several key components that ensure its successful implementation and long-term sustainability. Each component plays a distinct role in enhancing the overall effectiveness of the semantic layer.
Data Abstraction in Semantic Layer
Data abstraction is critical in semantic layer management, as it strips away extraneous details and presents data in a more digestible format. This not only helps users quickly grasp complex concepts but also supports better analytics outcomes. By abstracting data, organizations can enable users to conduct analyses without needing to understand the intricacies of the data’s source or structure.
Implementing effective data abstraction strategies may involve the use of views, models, and analytical tools that encapsulate the data in user-friendly formats. By doing so, organizations empower their users to explore data with clarity and ease. Furthermore, the abstraction process can be tailored to different user roles, allowing for customized experiences that cater to both technical and non-technical users. This adaptability ensures that everyone, from data scientists to business analysts, can derive insights from the data without feeling overwhelmed by its complexity.
Metadata Management and Its Importance
Metadata management is another cornerstone of effective semantic layer management. It encompasses the processes involved in collecting, organizing, and maintaining information about data resources. Proper metadata allows users to discover, use, and interpret data more efficiently.
Effective metadata management enhances the usability and accessibility of data, fostering a culture of data literacy within the organization. It allows for context to be imbued within the data, ensuring that users not only have access to the raw data but also understand its significance, provenance, and limitations. Additionally, robust metadata practices can facilitate compliance with data governance policies, as they provide a clear lineage of data sources and transformations. This transparency is crucial in industries where regulatory requirements demand strict adherence to data handling protocols, thereby reducing the risk of non-compliance and enhancing trust in the data-driven decision-making process.
Essential Practices for Effective Semantic Layer Management
To maximize the strategic value of semantic layer management, organizations must adopt essential practices that create a robust framework for data governance, quality, security, and collaboration.
Establishing a Robust Data Governance Framework
Data governance is a fundamental practice that underpins successful semantic layer management. It involves defining roles, responsibilities, and policies for data management across the organization. A solid governance framework ensures accountability and promotes consistent practices in data handling.
In implementing data governance, it's vital to engage stakeholders from various departments to create a comprehensive and inclusive strategy. This collaboration fosters alignment on data definitions and promotes a shared understanding of data use across the organization. Additionally, establishing a data stewardship program can empower individuals to take ownership of specific datasets, ensuring that data remains accurate and relevant over time. Regular training sessions and workshops can further enhance the understanding of governance policies, enabling employees to recognize their role in maintaining data integrity.
Ensuring Data Quality and Consistency
Data quality is indispensable to effective semantic layer management; poor-quality data can lead to misguided insights and decisions. Organizations should implement processes for regular data validation, cleansing, and enrichment to maintain data integrity.
Establishing automated data quality checks ensures that anomalies are swiftly identified and addressed. By fostering a culture that prioritizes data quality, organizations can enhance the reliability of the insights derived from their semantic layer. Moreover, leveraging advanced analytics and machine learning techniques can help in predicting potential data quality issues before they escalate. This proactive approach not only saves time and resources but also enhances the overall trustworthiness of the data, allowing teams to make informed decisions based on accurate and timely information.
Implementing Effective Data Security Measures
With the increasing volume and sensitivity of data, robust security measures are paramount in semantic layer management. Organizations must develop policies for data protection, access control, and encryption to safeguard their data assets.
Regular audits and compliance assessments are essential in identifying vulnerabilities and ensuring adherence to regulations. By prioritizing data security, organizations can build trust with users and stakeholders, enabling safe and responsible data use. Additionally, employing multi-factor authentication and role-based access controls can further enhance security measures, ensuring that only authorized personnel can access sensitive information. Continuous monitoring of data access patterns can also help in detecting any unusual activities, allowing organizations to respond swiftly to potential threats and mitigate risks effectively.
The Impact of Semantic Layer Management on Business Intelligence
The implementation of effective semantic layer management practices has far-reaching implications for business intelligence initiatives. By optimizing the flow of data within the organization, companies can harness valuable insights to drive strategic decisions and operational efficiency.
Enhancing Data Accessibility and Usability
Semantic layer management significantly enhances data accessibility and usability for non-technical users. By translating complex data structures into user-friendly language, organizations empower employees across all levels to engage with data confidently.
This democratization of data access fosters a culture of data-driven decision-making where insights are derived collaboratively, leading to more informed and aligned actions across departments. Furthermore, as employees become more adept at navigating and interpreting data, they can contribute innovative ideas and solutions that might otherwise go unnoticed. This not only boosts morale but also cultivates a sense of ownership over the data, encouraging a proactive approach to problem-solving.
Facilitating Better Decision Making
Quality insights are imperative for effective decision-making processes. By managing the semantic layer diligently, organizations can ensure that decision-makers have access to accurate, relevant, and timely data. This responsiveness can be a crucial competitive advantage in today’s fast-paced business landscape.
With streamlined data access and clear representation, leaders become better equipped to identify opportunities, mitigate risks, and craft strategies that resonate with organizational goals. Additionally, the integration of advanced analytics tools within the semantic layer can further enhance decision-making capabilities. Predictive analytics, for example, allows organizations to not only react to current trends but also anticipate future developments, enabling them to stay ahead of the curve. This proactive stance is essential in a world where market dynamics can shift rapidly, and the ability to pivot based on data-driven insights can make all the difference in achieving long-term success.
Overcoming Challenges in Semantic Layer Management
Implementing and managing a semantic layer can present challenges that require strategic solutions. Organizations must recognize these challenges and tackle them systematically to ensure the sustainability of their semantic layer efforts.
Dealing with Data Complexity
Data complexity is a common challenge within organizations that manage vast and varied data sets. As data continues to grow in variety and volume, simplifying this complexity is crucial for maintaining an efficient semantic layer.
Adopting technologies and frameworks that simplify data integration while maintaining the integrity of underlying structures is essential. Employing ETL (Extract, Transform, Load) processes can facilitate clearer data pipelines that alleviate complexities for users.
Addressing Data Integration Issues
Data integration remains a pressing challenge in semantic layer management. Disparate data sources can lead to inconsistencies and confusion, undermining the effectiveness of the semantic layer.
To mitigate integration issues, organizations should invest in tools and platforms that specialize in data federation and integration. By ensuring that data is correctly mapped and aligned, organizations can promote a coherent data environment that enhances the effectiveness of their semantic layer.
Ready to elevate your semantic layer management and harness the full power of self-service analytics? Look no further than CastorDoc. With advanced governance, cataloging, and lineage capabilities, CastorDoc is the comprehensive solution your business needs to ensure data quality, compliance, and effective data utilization. Experience the ease of managing your data lifecycle with CastorDoc's AI assistant, and empower your team to make data-driven decisions with confidence. Try CastorDoc today and revolutionize the way you manage and leverage your organization's data for long-term success.
You might also like
Get in Touch to Learn More
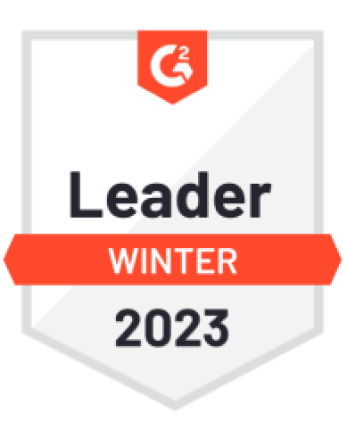
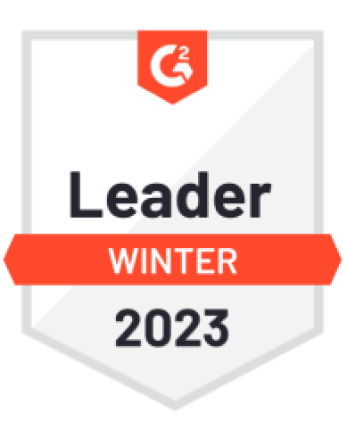

“[I like] The easy to use interface and the speed of finding the relevant assets that you're looking for in your database. I also really enjoy the score given to each table, [which] lets you prioritize the results of your queries by how often certain data is used.” - Michal P., Head of Data