Semantic Layer Meaning: What It Is and Why It Matters
Discover the significance of the semantic layer in data analytics.
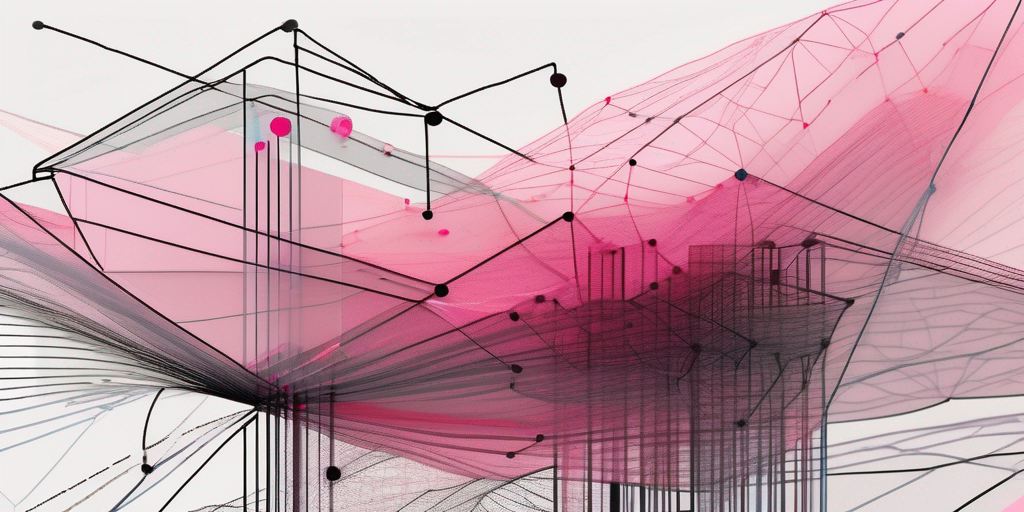
Understanding the Concept of Semantic Layer
The semantic layer serves as an intermediary between complex data structures and end-users seeking to understand and utilize that data effectively. It simplifies the process of data analysis, providing a consistent framework that abstracts the underlying complexities of the data sources. By doing so, it allows users to interact with data in a more intuitive manner, promoting a better understanding of the information at hand.
Definition of Semantic Layer
A semantic layer can be defined as a business representation of data that enhances the accessibility and usability of complex data sets. It employs business-friendly terminology, allowing users to query data without needing to understand the technicalities of the underlying data schema. This abstraction transforms technical metadata into user-centric concepts.
For instance, instead of requiring users to comprehend complex database architectures or programming languages, the semantic layer enables them to work with identifiable terms relevant to their roles. This makes analysis more approachable, fostering a more data-driven culture within organizations. Moreover, the semantic layer can also facilitate cross-departmental collaboration by providing a common vocabulary that different teams can understand, reducing miscommunication and enhancing the overall efficiency of data-driven projects.
The Role of Semantic Layer in Data Management
The semantic layer plays a crucial role in data management by acting as a bridge between raw data and business analytics. It allows organizations to create a unified view of their data while ensuring data integrity and consistency across various sources. By providing a coherent framework, businesses can efficiently manage their data assets.
Additionally, it streamlines the process of data preparation, significantly reducing the time and resources required for analysis. With a well-implemented semantic layer, users can access accurate and relevant data faster, enabling timely decision-making that is critical in today’s competitive environment. Furthermore, the semantic layer can enhance data governance by embedding rules and standards that ensure compliance and security, allowing organizations to trust the data they are working with. This layer not only promotes efficiency but also empowers users to derive insights that can lead to innovative strategies and improved operational performance.
The Importance of Semantic Layer in Business Intelligence
The implementation of a semantic layer is vital for organizations aiming to enhance their business intelligence capabilities. It significantly improves the way data is accessed and utilized, laying the groundwork for effective decision-making processes across all levels of the organization.
Enhancing Data Accessibility
One of the primary functions of a semantic layer is improving data accessibility. By translating intricate data schemas into business-friendly terms, users from various departments can analyze information without needing a comprehensive understanding of the underlying databases. This democratization of data fosters an environment where data-driven decision-making becomes the norm.
Moreover, the semantic layer can provide a consolidated view of disparate data sources, allowing users to access all relevant information from a single point. This approach eliminates data silos and encourages cross-functional collaboration, ultimately driving performance and innovation within the organization. As a result, teams can work more cohesively, sharing insights and strategies that leverage the full spectrum of available data, which can lead to more innovative solutions and enhanced competitive advantages.
Facilitating Better Decision Making
The semantic layer empowers decision-makers by enabling them to generate insights quickly and efficiently. By presenting data in a meaningful context, it allows users to focus on analysis rather than getting bogged down in technical details. This setting enhances critical thinking and supports more informed decision-making.
Furthermore, by standardizing data definitions and calculations, the semantic layer ensures consistency in reporting and analytics, which is essential for making strategic decisions. When every stakeholder relies on the same definitions and metrics, organizations can operate with a common understanding, significantly boosting collaboration and effectiveness. This consistency not only reduces the risk of errors but also builds trust in the data being used, as stakeholders can be confident that they are all interpreting the same information in the same way. Additionally, the semantic layer can facilitate advanced analytics capabilities, such as predictive modeling and machine learning, by providing a robust framework for data integration and analysis, ultimately leading to more proactive and strategic business initiatives.
Components of a Semantic Layer
The effectiveness of a semantic layer hinges on several key components that work together to create a cohesive data framework. Understanding these components can help organizations design more effective semantic layers tailored to their specific needs.
Metadata
Metadata lies at the core of the semantic layer. It encompasses data about the data, providing context and meaning to the raw information stored within databases. Metadata helps users understand the origin, structure, and timing of the data, facilitating more insightful analyses.
Moreover, it ensures that users can easily find the relevant data points they need. By employing well-structured metadata, organizations can create a rich, user-friendly repository of information that fosters efficient data retrieval and analysis. This structured approach not only enhances the user experience but also supports data governance initiatives by ensuring that data lineage is traceable and understandable. As organizations scale and their data environments become more complex, having a robust metadata framework becomes increasingly vital for maintaining clarity and coherence in data management.
Business Rules
Business rules define how data should be interpreted and used within the organization. These rules might include specific calculations, data transformations, or filtering criteria that dictate how data is aggregated and analyzed. Implementing business rules within the semantic layer ensures that the data users interact with adheres to organizational standards and practices.
This adherence helps maintain consistency in reporting and performance metrics, enabling organizations to establish trust in their data and the insights derived from it. When users understand how and why data is processed in a particular way, they are more likely to make informed decisions based on that data. Additionally, the establishment of clear business rules can streamline workflows and reduce the likelihood of errors, as users are guided by predefined protocols that reflect best practices and operational objectives. This not only enhances data quality but also empowers teams to leverage data more effectively in their strategic initiatives.
Security Parameters
Security is paramount in managing sensitive business data. The semantic layer should incorporate robust security parameters to control access to data based on user roles and permissions. By implementing fine-grained security measures, organizations can protect their data while still promoting accessibility where appropriate.
Security parameters ensure compliance with regulatory standards and protect against data breaches. They allow organizations to safely share data across departments while maintaining confidentiality and data integrity. Furthermore, implementing advanced security measures such as encryption and multi-factor authentication can bolster the overall security posture of the semantic layer. As cyber threats evolve, organizations must remain vigilant and proactive in updating their security frameworks to safeguard their data assets. This not only protects sensitive information but also builds a culture of security awareness among users, fostering a collective responsibility towards data protection across the organization.
Building an Effective Semantic Layer
Creating a successful semantic layer is not a one-size-fits-all process. It involves careful consideration of various factors to ensure alignment with organizational goals and data strategies.
Key Considerations
When building a semantic layer, organizations should prioritize user needs. Identifying the types of data and insights most relevant to end-users is crucial. This process may involve conducting user interviews or surveys to understand how different departments utilize data.
Additionally, organizations need to ensure that the semantic layer is scalable and adaptable to evolving data environments. As requirements change, the semantic layer should be able to accommodate new data sources, metrics, and business rules without compromising performance or usability.
Common Challenges and Solutions
Despite its advantages, implementing a semantic layer can present challenges. Common roadblocks include resistance to change, technological limitations, and data quality issues. To overcome these challenges, organizations can invest in training initiatives to bolster user adoption or utilize data governance practices to enhance data quality and consistency.
Additionally, leveraging tools and technologies specifically designed for building semantic layers can streamline the process and mitigate some technical hurdles. Proper planning and resource allocation can dramatically improve the success rate of implementing an effective semantic layer.
The Future of Semantic Layers
The landscape of data management and analysis is continuously evolving, and the semantic layer is set to play a pivotal role in shaping its future. As organizations increasingly recognize the importance of data-driven insights, the necessity for intuitive and accessible data frameworks becomes apparent.
Emerging Trends
Emerging trends in technology, such as artificial intelligence and machine learning, are likely to influence the development of semantic layers. These technologies can automate data categorization and enhance the semantic layer's ability to suggest insights based on user behavior and preferences, offering a more personalized analytical experience.
Incorporating these advancements can make semantic layers not just a tool for data retrieval but also an intelligent assistant that helps guide users through their data analysis journey.
Potential Impact on Data Analysis and Reporting
The evolution of semantic layers promises to transform data analysis and reporting. By enhancing the user experience and integrating more powerful analytical capabilities, organizations can expect faster and more accurate insights. This shift has the potential to empower all business users, further embedding data into the decision-making processes at all levels.
As organizations harness the power of a well-designed semantic layer, they will be better positioned to navigate the complexities of data analytics and ultimately achieve a competitive advantage in their respective industries.
Ready to elevate your data management and analytics capabilities? CastorDoc is here to transform your business intelligence efforts. With its advanced governance, cataloging, and lineage features, combined with a user-friendly AI assistant, CastorDoc is the ultimate tool for enabling self-service analytics. Say goodbye to the complexities of data analysis and embrace a future where data is accessible, understandable, and fully integrated into your decision-making process. Try CastorDoc today and experience the power of a semantic layer that's enhanced by AI, designed for both data professionals and business users alike. Unlock the full potential of your data and gain a competitive edge with CastorDoc.
You might also like
Get in Touch to Learn More
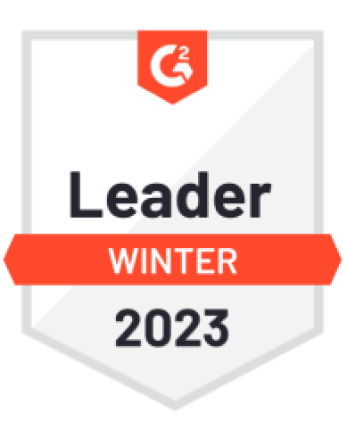
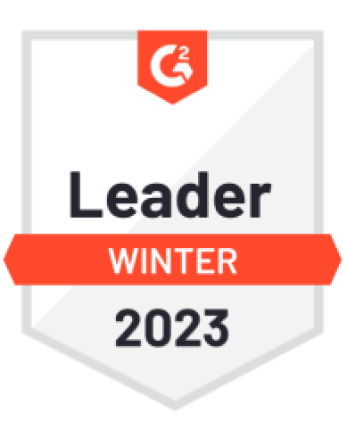

“[I like] The easy to use interface and the speed of finding the relevant assets that you're looking for in your database. I also really enjoy the score given to each table, [which] lets you prioritize the results of your queries by how often certain data is used.” - Michal P., Head of Data