A Comprehensive Guide to Spatial Data: Types, Examples, and Use Cases
Explore the world of spatial data with this guide, covering various types, real-life examples, and practical use cases.
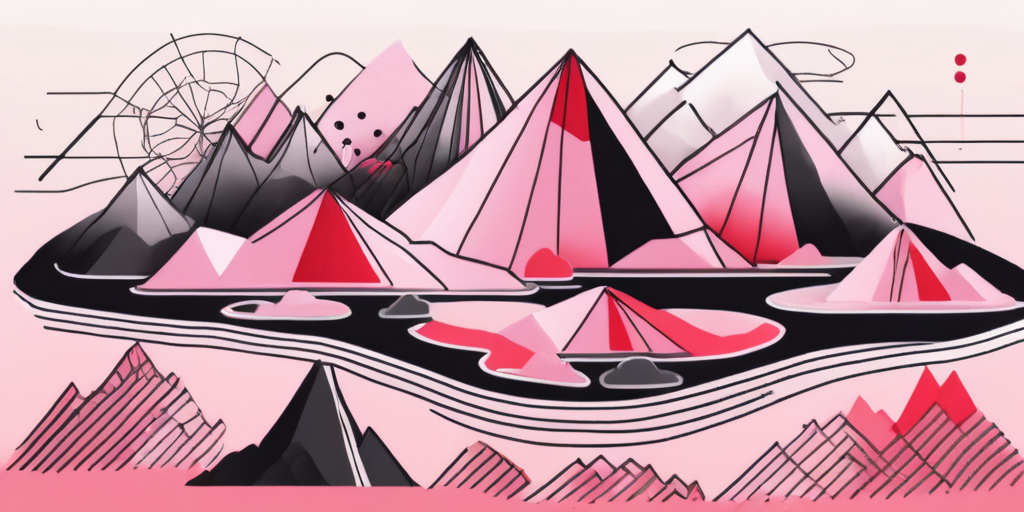
Spatial data plays a crucial role in various industries and sectors today. From urban planning to environmental management, from science to business, the applications of spatial data are vast and diverse. In this comprehensive guide, we will explore the different types of spatial data, provide examples of their use cases, and delve into the various tools and techniques used for spatial data analysis. Whether you are a seasoned GIS professional or new to the world of spatial data, this guide will provide you with a solid understanding of the subject.
Demystifying Spatial Data: Examples and Explanations
Spatial data refers to information that is tied to a particular location. It can include coordinates, addresses, boundaries, and attributes associated with specific objects or phenomena. Let's start by exploring some real-life examples of how spatial data is applied in urban planning.
Applying Spatial Data in Urban Planning
In urban planning, spatial data is vital for making informed decisions and creating sustainable development strategies. By analyzing spatial data, city planners can identify areas of high population density, assess infrastructure needs, and plan efficient transportation networks. Spatial data helps in visualizing and understanding the spatial relationships between different elements of a city, such as parks, buildings, and roads. This information is crucial for designing livable and functional urban spaces.
For example, when planning the construction of a new residential neighborhood, urban planners can use spatial data to determine the optimal location for amenities such as schools, parks, and healthcare facilities. By considering factors like population density, access to public transportation, and proximity to existing infrastructure, planners can optimize the layout of the neighborhood to meet the needs of its future residents.
In addition to urban planning, spatial data has a wide range of applications in other sectors as well. Let's explore the different types of spatial data and how they are used in various contexts.
Navigating the Landscape of Spatial Data Types
There are several types of spatial data, each serving different purposes and requiring distinct analysis techniques. The main types of spatial data are vector, raster, attribute, and temporal data.
A Closer Look at Vector, Raster, Attribute, and Temporal Data
Vector data represents spatial objects as points, lines, or polygons. For example, vector data can be used to represent roads, buildings, or land parcels. Vector data is particularly useful for precise measurements and creating accurate maps. It is often used in GIS (Geographic Information System) applications.
Raster data, on the other hand, represents spatial information as a grid of cells. Each cell in the grid contains a value that represents a specific attribute. Raster data is commonly used to represent satellite imagery, elevation data, and other continuous phenomena. It enables the analysis of trends and patterns over large areas.
Attribute data provides additional information about spatial objects. It can include data such as population demographics, land use classifications, or environmental factors. Attribute data enhances the analysis of spatial data by bringing in context and enabling the creation of thematic maps.
Temporal data captures how spatial phenomena change over time. It is particularly important in monitoring phenomena such as climate change, population growth, or traffic patterns. By analyzing temporal data, trends and patterns can be identified, contributing to better decision-making processes.
Now that we have a better understanding of the different types of spatial data, let's explore their journey from collection to application.
From Collection to Application: The Journey of Spatial Data
The effective use of spatial data involves several stages, including data collection, storage, analysis, and visualization. Let's take a closer look at each stage and its role in the journey of spatial data.
Understanding the Components and Functionality of Spatial Data
Data collection is the foundation of any spatial analysis. It involves gathering data from various sources, such as surveys, satellite imagery, GPS devices, or existing databases. Once the data is collected, it needs to be stored in a format that allows for efficient retrieval and analysis.
Spatial data analysis involves exploring, manipulating, and interpreting the data to uncover patterns, relationships, and insights. This can involve tasks such as spatial queries, geoprocessing, statistical analysis, or predictive modeling. The analysis stage is where the true value of spatial data is realized.
Data visualization is an important aspect of spatial data analysis, as it allows for the effective communication of findings and insights. Visualizations can take the form of maps, charts, or interactive dashboards. By visualizing spatial data, complex information can be presented in a digestible and actionable manner.
The journey of spatial data is not complete without understanding its impact on decision-making processes.
The Crucial Role of Spatial Data in Decision-Making Today
Across different sectors, spatial data plays a crucial role in informed decision-making. Let's explore the impact of spatial data in a variety of fields such as science, policy, business, and health.
Impact of Spatial Data in Various Sectors: Science, Policy, Business, Health, and More
In the field of science, spatial data is used for ecological modeling, climate change analysis, and biodiversity conservation. Scientists can analyze spatial data to understand patterns of species migration, assess the impact of human activities on the environment, and make predictions about future changes.
In the policy realm, spatial data helps policymakers understand the spatial distribution of populations, plan for infrastructure development, and manage natural resources effectively. Spatial data analysis informs policy decisions related to urban development, transportation planning, and disaster management.
Businesses can leverage spatial data for market analysis, site selection, and supply chain optimization. Spatial data analysis can provide insights into customer behavior, identify potential markets, and improve logistical efficiency. Location-based services, such as ride-hailing apps and online navigation tools, rely on spatial data to provide accurate and real-time information to users.
In the healthcare sector, spatial data is used for disease mapping, resource allocation, and emergency response planning. By analyzing spatial data, health professionals can identify areas with high disease prevalence, allocate medical resources to areas in need, and plan for efficient emergency response strategies.
Now that we have explored the impact of spatial data in various sectors, let's dive into some real-world examples of its applications.
Real-World Applications of Spatial Data
The versatility of spatial data is evident in its wide range of applications. From urban planning to environmental management, spatial data finds use in multiple domains. Let's explore some real-world examples of how spatial data is harnessed.
Harnessing Spatial Data for Urban Planning, Environmental Management, and More
In urban planning, spatial data is used to design sustainable cities, analyze transportation networks, and optimize resource allocation. By understanding the spatial relationships between different elements of a city, planners can create functional and livable urban spaces that meet the needs of their residents.
In environmental management, spatial data is crucial for monitoring ecosystems, mapping habitats, and identifying areas of conservation importance. By using spatial data, scientists and policymakers can make informed decisions about habitat restoration, land-use planning, and protected area management.
Other applications of spatial data include disaster management, precision agriculture, and transportation planning. In disaster management, spatial data is used to model and predict the impact of natural disasters, plan evacuation routes, and coordinate emergency response efforts. In precision agriculture, spatial data is employed to optimize crop yields, monitor soil health, and reduce the use of agrochemicals. In transportation planning, spatial data is vital for designing efficient transportation networks, predicting traffic patterns, and optimizing the location of transportation hubs.
Unveiling the versatile nature of spatial data, let's now explore its role in GIS, graphics, statistics, and virtual reality.
Unveiling the Versatility of Spatial Data: From GIS to Virtual Reality
Spatial data plays a fundamental role in Geographic Information Systems (GIS), graphics, statistics, and virtual reality. Let's delve into how spatial data is leveraged in each of these domains.
Leveraging Spatial Data for GIS, Graphics, Statistics, and Virtual Reality
In GIS, spatial data serves as the backbone for creating maps, conducting spatial analysis, and supporting decision-making processes. GIS platforms provide the tools and functionalities to store, manipulate, and visualize spatial data. From creating custom maps to analyzing spatial patterns, GIS enables professionals to explore and derive insights from spatial data.
In graphics, spatial data is used to create visually appealing and accurate representations of the real world. Whether it's a 3D model of a city skyline or a satellite imagery visualization, spatial data forms the foundation for creating realistic and immersive graphics.
In statistics, spatial data analysis techniques, such as spatial interpolation and cluster analysis, are used to identify spatial patterns and relationships. Through spatial statistics, researchers can uncover hidden trends and make informed decisions based on the spatial distribution of data.
In virtual reality (VR), spatial data is used to create immersive and interactive experiences. By leveraging spatial data, VR applications can simulate realistic environments, allowing users to explore different locations and interact with virtual objects.
Finally, let's explore some essential tools that facilitate spatial data analysis.
Essential Tools for Spatial Data Analysis
Effective analysis of spatial data requires the use of specialized software and tools. Let's explore some essential tools used in spatial data analysis, including GIS software, statistical tools, and data visualization platforms.
Exploring GIS Software, Statistical Tools, and Data Visualization Platforms
GIS software provides the necessary tools for analyzing, manipulating, and visualizing spatial data. Popular GIS software options include ArcGIS, QGIS, and GRASS GIS. These software packages offer a wide range of functionalities, from spatial query capabilities to advanced geoprocessing tools.
Statistical tools such as R and Python's libraries (e.g., pandas, GeoPandas) are commonly used for spatial data analysis. These tools enable statistical modeling, spatial regression analysis, and spatial cluster detection. They provide a flexible and powerful environment for analyzing complex spatial data.
Data visualization platforms like Tableau and Power BI allow users to create interactive and visually compelling representations of spatial data. These tools make it easy to create maps, charts, and dashboards, enabling effective communication of spatial insights to a wider audience.
As we conclude our comprehensive guide to spatial data, we hope you have gained a solid understanding of the different types of spatial data, their applications, and the tools used for spatial data analysis. Spatial data is a powerful tool that has the potential to revolutionize decision-making processes across various sectors. By harnessing the power of spatial data, we can better understand and shape the world around us.
As you've seen, spatial data is integral to shaping the decisions that drive progress in various sectors. If you're looking to elevate your organization's ability to manage and analyze spatial data, CastorDoc is your go-to solution. With its advanced governance, cataloging, and lineage capabilities, coupled with a user-friendly AI assistant, CastorDoc is designed to empower your team with self-service analytics. Whether you're a data professional seeking to streamline governance processes or a business user aiming to harness data for strategic insights, CastorDoc's intuitive platform and AI copilot will transform the way you interact with data. Ready to revolutionize your spatial data analysis and decision-making? Try CastorDoc today and unlock the full potential of your data.
You might also like
Get in Touch to Learn More
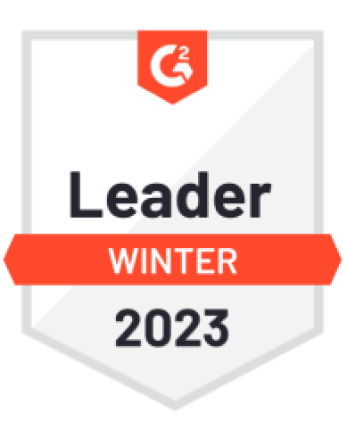
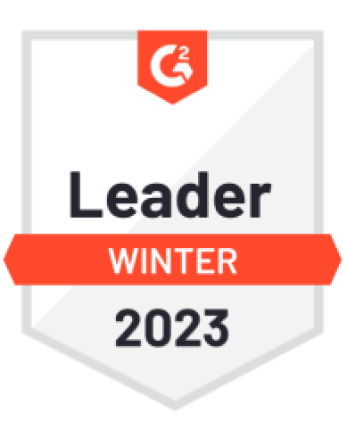

“[I like] The easy to use interface and the speed of finding the relevant assets that you're looking for in your database. I also really enjoy the score given to each table, [which] lets you prioritize the results of your queries by how often certain data is used.” - Michal P., Head of Data