The Differences Between Data Lineage and Data Provenance
Discover the distinctions between data lineage and data provenance in our comprehensive article.

Data lineage and data provenance are two important concepts in the field of data management. While they are closely related, there are key differences between these two terms. It is crucial to understand these differences in order to effectively manage and analyze data in various industries and applications.
In this article, we will explore the definitions of data lineage and data provenance, delve into their roles in data management, highlight their key features, discuss their practical applications, and address the challenges in implementing them.
Understanding Key Concepts: Data Lineage and Data Provenance
Before we delve into the differences between data lineage and data provenance, let's define these terms.
When it comes to managing data effectively, understanding the concepts of data lineage and data provenance is crucial. These two terms play a significant role in ensuring data quality, reliability, and traceability within organizations.
Defining Data Lineage
Data lineage refers to the ability to trace and understand the origins, transformations, and movements of data throughout its lifecycle. It provides a comprehensive view of how data is created, modified, and exchanged within an organization or system.
By capturing metadata and tracking data transformations, data lineage allows organizations to answer critical questions about the source, quality, and reliability of data. It enables data scientists, analysts, and other stakeholders to gain insights into the data and make informed decisions based on its lineage.
Understanding data lineage is essential for ensuring data governance and compliance. It helps organizations maintain data integrity, identify potential issues or errors in data processes, and improve overall data management practices.
Defining Data Provenance
Data provenance, on the other hand, focuses on capturing the history and lineage of data in a more detailed and granular manner. It aims to provide a complete audit trail of data, including information about its creators, processes, and inputs.
Data provenance encompasses not only the movement of data but also the contextual information surrounding it. It captures the why and how behind data transformations, enabling users to understand the reasons for specific changes made to the data.
Having a clear understanding of data provenance is crucial for ensuring data transparency and accountability. It allows organizations to track data back to its source, verify its authenticity, and ensure compliance with data regulations and policies.
The Importance of Data Lineage and Data Provenance
Role of Data Lineage in Data Management
Data lineage plays a crucial role in ensuring data quality, compliance, and trustworthiness. By tracing the origin of data, organizations can identify any potential issues or errors that may have occurred during data collection, processing, or integration.
For example, imagine a large retail company that collects customer data from various sources, such as online purchases, in-store transactions, and loyalty programs. With data lineage, the company can track the journey of this data, from the moment it is captured to its final destination in the data warehouse. This allows them to identify any discrepancies or inconsistencies that may have occurred along the way, ensuring that the data is accurate and reliable.
Furthermore, data lineage facilitates data governance and regulatory compliance efforts. It provides transparency and accountability, allowing organizations to demonstrate data lineage to auditors and regulatory bodies. This is particularly important in industries that are heavily regulated, such as finance and healthcare. By having a clear understanding of where the data comes from and how it has been processed, organizations can ensure that they are meeting all the necessary compliance requirements.
Additionally, data lineage enhances the efficiency of data analysis and troubleshooting. It enables data scientists and analysts to easily track the flow of data and identify the impact of data changes on downstream processes or reports. This can help them quickly identify and resolve any issues that may arise, saving time and resources.
Role of Data Provenance in Data Management
Data provenance offers a more comprehensive view of data by capturing detailed information about its lineage. This enables organizations to have a deeper understanding of the data's context and reliability.
For instance, let's consider a research institution conducting experiments to study the effects of a new drug. With data provenance, the institution can not only track the origin of the data but also capture additional information such as the equipment used, the methodology followed, and the personnel involved in the experiment. This level of detail provides a complete picture of the data's provenance, allowing researchers to confidently analyze and interpret the results.
With data provenance, organizations can verify the authenticity and integrity of data. This is particularly important in domains such as scientific research and legal compliance, where the accuracy and traceability of data are paramount. By having a clear record of the data's provenance, organizations can ensure that the data has not been tampered with or manipulated, maintaining its integrity and reliability.
Furthermore, data provenance supports data integration and data fusion efforts by providing insights into the compatibility and suitability of different datasets. It helps data professionals make informed decisions about data integration strategies and identify potential issues with data compatibility. For example, in a merger or acquisition scenario, where two organizations need to combine their datasets, data provenance can help identify any inconsistencies or conflicts between the datasets, enabling data professionals to address them effectively.
As organizations continue to deal with increasingly complex and diverse data sources, data lineage and data provenance play a vital role in ensuring data quality, compliance, and trustworthiness. By understanding the importance of these concepts and implementing robust data management practices, organizations can harness the full potential of their data and make informed decisions based on accurate and reliable information.
Distinguishing Between Data Lineage and Data Provenance
Key Features of Data Lineage
One key feature of data lineage is its focus on tracking the journey of data from its source to its destination. It identifies the datasets involved, the operations performed on the data, and the transformations applied at each stage.
Data lineage also emphasizes capturing metadata, such as data types, data formats, and data quality metrics. This information helps users understand the characteristics of the data and assess its fitness for specific purposes.
Key Features of Data Provenance
Data provenance, on the other hand, places a stronger emphasis on capturing the contextual information surrounding the data. It aims to answer questions about the motivations and intentions of data creators, as well as the reasons behind specific data transformations.
Data provenance captures additional information, such as timestamps, user identities, and process descriptions. This enables users to understand the who, what, when, and why of data changes, providing a richer context for data analysis and decision-making.
Practical Applications of Data Lineage and Data Provenance
Data Lineage in Business Intelligence
In the realm of business intelligence, data lineage is crucial for ensuring data accuracy and reliability. It enables organizations to trace the sources of data used in reports and analytics, ensuring that decision-makers have access to trustworthy insights.
Moreover, data lineage helps identify any data quality issues that may impact business intelligence initiatives. By understanding the journey of data, organizations can pinpoint the root causes of inconsistencies, errors, or anomalies in their reports and take corrective actions.
Data Provenance in Research and Development
In research and development fields, data provenance plays a pivotal role in ensuring scientific reproducibility and data integrity. It allows researchers to track and validate the steps taken during experiments, ensuring that results can be accurately replicated.
Data provenance also helps identify potential biases, errors, or outliers in datasets. By understanding the origin and history of data, researchers can make informed decisions about data inclusion or exclusion, ensuring that their conclusions are based on sound and reliable information.
Challenges in Implementing Data Lineage and Data Provenance
Common Obstacles in Data Lineage Implementation
Implementing data lineage can be challenging due to various factors. One common obstacle is the complexity and diversity of data sources and technologies within an organization. Data lineage requires integration with different systems, databases, and data formats, which can pose technical difficulties and require significant coordination and collaboration.
Another challenge is the lack of standardized metadata models and data lineage frameworks. Organizations often struggle with defining consistent metadata vocabularies and establishing clear data lineage practices that can be applied consistently across different domains and applications.
Common Obstacles in Data Provenance Implementation
Data provenance implementation faces similar challenges as data lineage. However, the more detailed nature of data provenance adds additional complexities. Capturing and managing contextual information, such as user intentions and process descriptions, requires careful design and governance to ensure accuracy and completeness.
Furthermore, privacy and security concerns may arise when dealing with sensitive data. Organizations need to establish appropriate access controls and anonymization techniques to protect the confidentiality of data while still capturing meaningful provenance information.
Conclusion
In summary, data lineage and data provenance are crucial concepts in data management. While data lineage focuses on the traceability of data, data provenance provides a more detailed understanding of its context and history.
Both concepts play pivotal roles in ensuring data quality, compliance, and trustworthiness. They support decision-making and data analysis by providing insights into the origin, transformations, and movements of data.
In the rapidly evolving data landscape, understanding and implementing data lineage and data provenance can help organizations navigate the complexities of data management and unlock the true value of their data.
You might also like
Get in Touch to Learn More
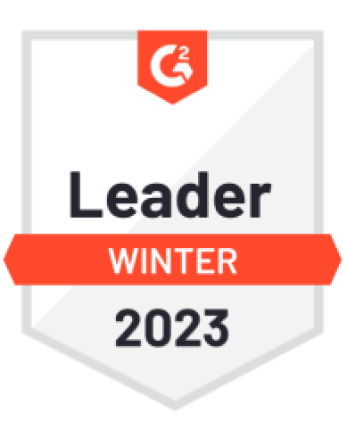
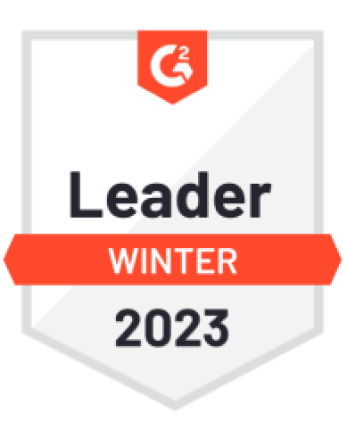

“[I like] The easy to use interface and the speed of finding the relevant assets that you're looking for in your database. I also really enjoy the score given to each table, [which] lets you prioritize the results of your queries by how often certain data is used.” - Michal P., Head of Data