The Evolution of Data Quality: From the Archives to the New Age
Explore the fascinating journey of data quality from its humble beginnings in the archives to its pivotal role in the modern age.

Data plays a crucial role in shaping decision-making and driving business success. However, the concept of data quality is not a new one. It has evolved over time, from its humble beginnings in the archives to the sophisticated techniques and tools we have today. Understanding this evolution is key to harnessing the power of data and ensuring its accuracy, reliability, and relevance.
Understanding Data Quality: A Historical Perspective
Data quality is not a novel concept but has its roots in the archives. In the early days, data quality was primarily concerned with the accuracy and completeness of records stored in physical formats. Archivists meticulously maintained and organized documents, ensuring they were legible, well-preserved, and accessible to future generations.
As technology advanced, so did the need for a more systematic approach to managing data quality. The progression of data quality standards has played a crucial role in shaping our understanding and practices in this field.
The Birth of Data Quality in the Archives
Before the digital revolution, data quality management mainly revolved around ensuring the integrity of physical records. Archivists employed rigorous processes to organize, classify, and preserve documents, ensuring their long-term usability. The emphasis was on accuracy, consistency, and reliability – principles that remain relevant even in the digital era.
Archivists not only focused on the content of the documents but also on the context in which they were created, providing valuable insights into historical events and societal norms. The meticulous care taken in preserving these records laid the foundation for modern data quality practices, emphasizing the importance of maintaining data integrity and ensuring its usability over time.
The Progression of Data Quality Standards
As data management shifted from physical to digital formats, industry-wide standards for data quality emerged. Organizations recognized the importance of consistent and reliable data and developed frameworks to govern and assess its quality. Standards such as ISO 8000 and the Data Management Body of Knowledge (DMBoK) have revolutionized the way we perceive and measure data quality.
With the advent of big data and the increasing reliance on data-driven decision-making, the need for robust data quality standards has become more pressing than ever. Organizations now not only focus on the accuracy and completeness of data but also on aspects such as timeliness, relevance, and usability. The evolution of data quality standards continues to shape the way we collect, manage, and leverage data in a rapidly changing digital landscape.
The Role of Technology in Advancing Data Quality
With the advent of digital transformation, technology has played a pivotal role in driving advancements in data quality. The widespread adoption of digital systems and the abundance of data sources have necessitated innovative approaches to ensure the accuracy and reliability of information.
As organizations continue to embrace technological solutions for data management, the role of technology in advancing data quality becomes increasingly prominent. Beyond just ensuring accuracy, technology now enables organizations to delve deeper into their data, uncovering valuable insights and trends that were previously hidden. This shift towards more sophisticated data analysis not only improves data quality but also enhances decision-making processes across various industries.
The Impact of Digital Transformation on Data Quality
Digital transformation has brought about both opportunities and challenges for data quality. On one hand, it has facilitated the collection and storage of vast amounts of data, enabling organizations to make data-driven decisions. On the other hand, the sheer volume and variety of data sources have made it increasingly challenging to maintain data quality standards. Data verification, cleansing, and enrichment techniques have become essential in this era of digital abundance.
Moreover, the evolution of data quality management practices in response to digital transformation has led to the development of advanced tools and methodologies. Organizations are now leveraging cutting-edge technologies such as blockchain and data encryption to not only ensure data quality but also enhance data security and privacy. This holistic approach to data management underscores the critical role of technology in safeguarding the integrity of information in the digital age.
The Influence of Artificial Intelligence and Machine Learning
Artificial intelligence (AI) and machine learning (ML) have emerged as powerful tools in enhancing data quality. By automating data validation and cleansing processes, AI and ML algorithms can identify patterns, anomalies, and inconsistencies in large datasets more efficiently than human efforts alone. This not only speeds up data quality assessment but also reduces the risk of human error.
Furthermore, the integration of AI and ML in data quality initiatives has paved the way for predictive analytics and proactive data management strategies. These technologies can now anticipate potential data quality issues before they occur, allowing organizations to take preemptive measures and maintain high standards of data integrity. The continuous evolution of AI and ML capabilities holds great promise for the future of data quality, enabling organizations to stay ahead of the curve in an increasingly data-driven world.
The Current State of Data Quality
The evolution of data quality has led to the development of modern tools and techniques for managing and improving data quality. Today, organizations have access to a wide range of technologies that enable them to assess, monitor, and enhance the quality of their data assets.
In addition to the technological advancements, the human element also plays a crucial role in maintaining data quality. Data stewards and data quality analysts are key individuals responsible for overseeing data quality initiatives within organizations. They work closely with business users, data engineers, and data scientists to ensure that data is accurate, consistent, and reliable for decision-making purposes.
Modern Data Quality Tools and Techniques
Modern data quality tools encompass a broad range of functionalities, including data profiling, cleansing, standardization, and enrichment. These tools automate the processes of identifying and rectifying data issues, reducing the time and effort required for manual data validation. The use of metadata, data catalogs, and data lineage tracking has become essential in ensuring data quality transparency and traceability.
Data quality tools are continuously evolving to keep pace with the increasing volume and complexity of data. Machine learning algorithms and artificial intelligence are being integrated into data quality platforms to enable predictive data quality capabilities. These advanced tools can proactively identify potential data quality issues before they impact business operations, allowing organizations to take preemptive actions.
The Importance of Data Governance in Ensuring Quality
Data governance plays a pivotal role in ensuring data quality across an organization. Well-defined data governance frameworks establish accountability, ownership, and standards for data management. They define roles and responsibilities, establish data quality metrics, and provide mechanisms for identifying and resolving data quality issues. By embedding data governance practices into organizational culture, businesses can maintain high data quality standards and drive successful data-driven initiatives.
Furthermore, data governance is not just a one-time initiative but an ongoing process that requires continuous monitoring and improvement. Regular data quality audits, compliance checks, and stakeholder feedback mechanisms are essential components of a robust data governance program. By fostering a data-driven culture and promoting data literacy among employees, organizations can ensure that data governance principles are ingrained in day-to-day operations.
The Future of Data Quality
Data quality continues to evolve as new technologies and trends emerge. The future of data quality management holds immense potential for organizations willing to embrace innovation and stay ahead of the curve.
Emerging Trends in Data Quality Management
Emerging trends such as data profiling, data cataloging, and master data management are revolutionizing the field of data quality management. These practices enable organizations to gain deeper insights into their data, identify data quality issues proactively, and ensure consistent data quality across various systems.
The Role of Big Data and IoT in Shaping Data Quality
With the proliferation of big data and the Internet of Things (IoT), the volume and velocity of data are increasing exponentially. Ensuring data quality in this era of data abundance is paramount, as organizations heavily rely on accurate insights for strategic decision-making. Advanced analytics techniques, combined with robust data quality practices, can help organizations extract meaningful information from vast datasets, thereby improving business outcomes.
Overcoming Challenges in Data Quality Management
While advancements in data quality management have provided organizations with powerful tools and techniques, challenges still exist. Overcoming these challenges is crucial to maintaining high-quality data and leveraging its full potential.
Strategies for Ensuring Consistent Data Quality
Ensuring consistent data quality requires a holistic approach that takes into account data governance, data quality assessment, and ongoing data stewardship. Organizations should establish robust data quality processes, define metrics and thresholds for quality, and actively involve data stewards in monitoring and resolving data quality issues.
The Role of Data Literacy in Enhancing Data Quality
Data literacy is increasingly important in the era of data-driven decision-making. Organizations must invest in training and educating their workforce on data literacy, ensuring that employees understand the importance of data quality and are equipped with the skills to assess, interpret, and utilize data effectively. By fostering a data-literate culture, businesses can enhance data quality and drive successful data-driven initiatives.
The evolution of data quality from its origins in the archives to the sophisticated practices we have today has transformed the way organizations utilize data. With the right tools, techniques, and strategies in place, businesses can harness the power of high-quality data to gain a competitive edge in the new age of data-driven decision-making.
You might also like
Get in Touch to Learn More
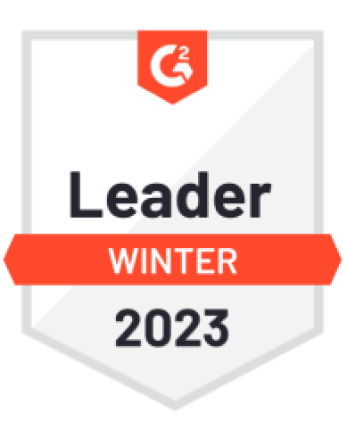
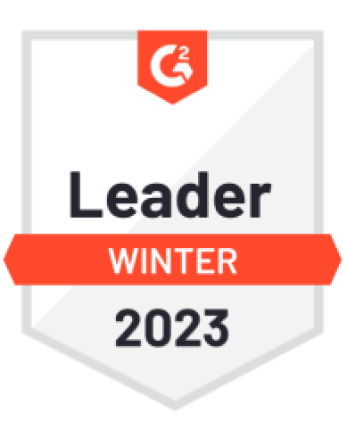

“[I like] The easy to use interface and the speed of finding the relevant assets that you're looking for in your database. I also really enjoy the score given to each table, [which] lets you prioritize the results of your queries by how often certain data is used.” - Michal P., Head of Data