The Ultimate Guide to Data Catalog for AI
Unlock the power of AI with our comprehensive guide to data cataloging.
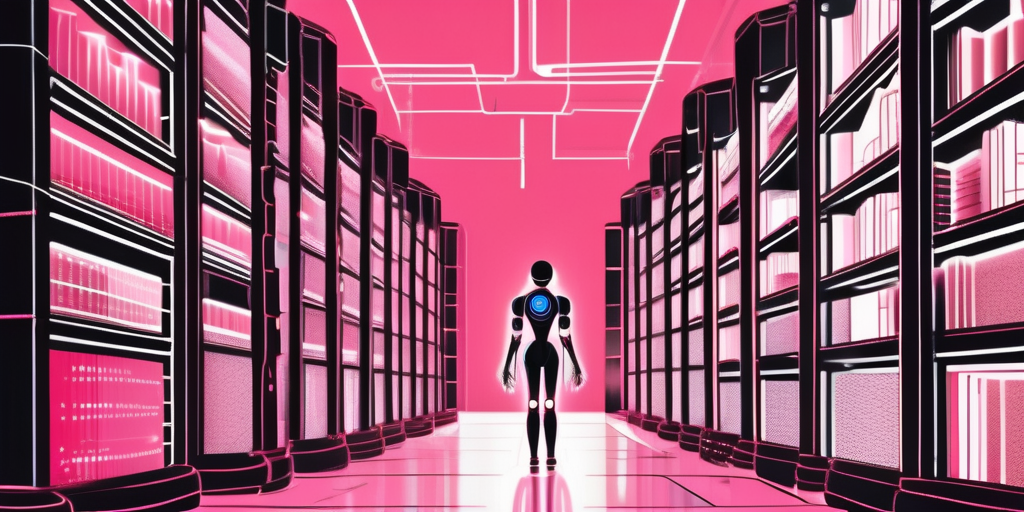
In today's data-driven world, managing and analyzing vast amounts of information is crucial for businesses to gain a competitive edge. The rise of artificial intelligence (AI) has further emphasized the need for organizations to have a comprehensive understanding of their data assets. This is where a data catalog comes into play, providing a centralized repository that enables efficient data discovery, organization, and governance.
Understanding the Basics of Data Catalog
A data catalog can be defined as a tool or system that allows organizations to manage and catalog their data assets. It serves as a digital library, containing detailed metadata about the available datasets. Metadata includes information such as the data source, structure, lineage, and other relevant attributes that help users discover, understand, and access the data they need for AI projects.
In the context of AI, data catalog plays a critical role in streamlining the data discovery process, ensuring data quality, and supporting data governance initiatives. It acts as a central hub where data scientists, analysts, and other stakeholders can easily search, explore, and access the data required for training machine learning models and other AI applications.
What is a Data Catalog?
A data catalog is a centralized repository that stores metadata about an organization's data assets. It provides a user-friendly interface for users to search, discover, and understand the available datasets. This includes information about data sources, data quality, data lineage, and other relevant attributes.
Importance of Data Catalog in AI
In the realm of AI, data serves as the lifeblood of algorithms and models. Without a solid understanding of available data assets, organizations may struggle to derive meaningful insights or make accurate predictions. That's where the importance of a data catalog comes into play.
By integrating a data catalog into their AI infrastructure, organizations can ensure that data scientists have easy access to the datasets they need for training and validation. It minimizes the time spent on searching for suitable data sources, reducing the overall time-to-insight for AI projects. Moreover, a data catalog promotes data reuse, preventing duplication of efforts and maximizing data ROI.
Key Components of a Data Catalog
A comprehensive data catalog consists of several key components that work together to provide an efficient and effective data management solution for AI projects. These components include:
- Data Discovery: A data catalog should have robust search capabilities, enabling users to discover relevant datasets based on various criteria, such as keywords, data domains, or data types. This feature plays a vital role in reducing the time and effort spent on finding suitable data sources.
- Data Profiling: Data profiling involves analyzing and summarizing the characteristics of datasets. A data catalog should offer automated data profiling capabilities, providing users with insights into the quality, completeness, and relevance of the data. This helps data scientists make informed decisions about data suitability for their AI projects.
- Data Lineage: Tracking the origin, transformations, and relationships between datasets is crucial for ensuring data reliability and trustworthiness. A data catalog should capture and visualize data lineage, allowing users to understand the data's journey and maintain transparency throughout the AI workflow.
- Data Governance: Data governance ensures compliance with regulatory requirements and internal policies. A data catalog should support data governance initiatives by integrating data classification, access controls, and data usage policies. This helps organizations maintain data privacy, security, and compliance.
Implementing a robust data catalog not only enhances the efficiency of AI projects but also fosters collaboration and knowledge sharing among data stakeholders. It provides a centralized platform where data scientists, analysts, and business users can collaborate, share insights, and contribute to the organization's data-driven initiatives.
Furthermore, a data catalog enables organizations to track the usage and performance of their data assets. By monitoring data access patterns and user feedback, organizations can gain valuable insights into the popularity and effectiveness of different datasets. This information can then be used to optimize data management strategies and allocate resources effectively.
Steps to Implement a Data Catalog for AI
Implementing a data catalog for AI requires careful planning, selecting the right tools, and establishing proper implementation and maintenance processes. Here are the key steps to follow:
Planning and Preparation
Prior to implementing a data catalog, organizations need to conduct a thorough assessment of their data landscape. This involves identifying existing data sources, understanding data dependencies, and defining data governance policies. A clear roadmap and implementation plan should be established to ensure the successful deployment of the data catalog.
During the planning phase, it is essential to involve key stakeholders from various departments to gather insights into their data needs and challenges. Understanding the different data requirements across the organization will help in designing a data catalog that caters to diverse needs. Additionally, conducting data quality assessments and data profiling exercises can provide valuable insights into the state of the data and help in setting data quality benchmarks for the catalog.
Choosing the Right Data Catalog Tools
The market offers a variety of data catalog tools, each with its own strengths and features. It is crucial to evaluate different options and select a tool that aligns with the organization's specific requirements. The chosen tool should provide efficient data discovery, data profiling, data lineage tracking, and data governance capabilities.
When selecting a data catalog tool, organizations should consider factors such as scalability, ease of integration with existing systems, user-friendliness, and vendor support. It is also important to assess the tool's compatibility with different data formats and sources to ensure seamless data ingestion and management. Pilot testing the selected tool with a subset of data can help in evaluating its performance and suitability for the organization's needs.
Implementation and Maintenance
Once a data catalog tool is selected, it is crucial to ensure smooth implementation and ongoing maintenance. This involves defining data catalog schemas, integrating with existing data management systems, configuring access controls, and establishing data quality monitoring processes. Continuous monitoring and periodic updates are essential to keep the data catalog up-to-date and relevant.
During the implementation phase, organizations should focus on providing adequate training to users on how to effectively utilize the data catalog for their data discovery and governance needs. Regular audits and reviews of the data catalog usage can help in identifying areas for improvement and optimizing the catalog for better performance. Collaboration between data stewards, data engineers, and data scientists is key to ensuring the data catalog remains a valuable asset for AI initiatives within the organization.
Benefits of Using a Data Catalog for AI
Implementing a data catalog for AI offers numerous benefits that help organizations unleash the full potential of their data assets. Some of the key advantages include:
Improved Data Quality
A data catalog enables organizations to profile and assess the quality of their data assets. By identifying data anomalies, inconsistencies, or incompleteness, organizations can take necessary measures to rectify or improve data quality. This results in more accurate AI models and reliable insights.
Enhanced Data Governance
With a data catalog in place, organizations can establish and enforce data governance policies effectively. They can define access controls, monitor data usage, and ensure compliance with regulatory requirements. This helps organizations maintain data privacy, security, and compliance, which is crucial in the age of AI.
Increased Efficiency in AI Projects
By providing a user-friendly interface for data discovery and access, a data catalog saves valuable time for data scientists and other stakeholders involved in AI projects. It allows them to quickly find and utilize relevant datasets, avoiding duplication of efforts and promoting collaboration. This boosts the overall efficiency of AI initiatives.
Facilitates Collaboration and Knowledge Sharing
Furthermore, a robust data catalog fosters collaboration among teams by providing a centralized platform for sharing data assets, insights, and best practices. Data scientists, analysts, and business users can easily discover and leverage each other's work, leading to enhanced innovation and problem-solving capabilities. This collaborative environment encourages cross-functional communication and learning, ultimately driving better outcomes for AI projects.
Scalability and Future-Proofing
Another significant advantage of utilizing a data catalog for AI is the scalability it offers. As organizations accumulate more data and expand their AI initiatives, a data catalog can adapt to growing needs by efficiently organizing and cataloging new datasets. This scalability ensures that the organization's data infrastructure remains agile and capable of supporting future AI advancements. By future-proofing data management processes, organizations can stay ahead in the rapidly evolving landscape of artificial intelligence.
Overcoming Challenges in Data Catalog Implementation
While implementing a data catalog for AI brings numerous benefits, organizations often face certain challenges that need to be addressed. These challenges include:
Data Privacy and Security Concerns
With the increasing amount of sensitive data being used for AI projects, organizations must prioritize data privacy and security. Implementing appropriate access controls, anonymization techniques, and ensuring compliance with data protection regulations is essential for managing privacy and security risks.
Data Integration Issues
Data catalogs need to integrate seamlessly with existing data management systems and tools. However, organizations often face challenges in data integration due to the heterogeneity of data sources, varying data formats, and complex integration requirements. Robust data integration strategies and tools are essential to overcome these challenges.
Managing Data Catalog Scalability
As the volume and complexity of data grow, organizations need to ensure that their data catalog can scale to accommodate new datasets, data sources, and user demands. Scalability challenges include storage capacity, search performance, and efficient data indexing. Regular monitoring and performance optimization are essential for maintaining a scalable data catalog.
In conclusion, a data catalog is a fundamental component of any AI-driven organization. It provides the necessary infrastructure to discover, manage, and govern data assets effectively. By following the steps outlined in this guide, organizations can successfully implement a data catalog and harness the true power of their data for AI projects. With improved data quality, enhanced data governance, and increased efficiency, organizations are well-equipped to drive successful AI initiatives and stay ahead in today's competitive landscape.
Ready to elevate your AI initiatives with a data catalog that does more than just organize your assets? Discover the transformative power of CastorDoc, where advanced governance meets user-friendly AI assistance. CastorDoc's robust data catalog is designed to streamline your data governance lifecycle, enhance regulatory compliance, and improve data quality with ease. Embrace the future of data management and empower your team with the ability to interact naturally with your data, fostering a culture of informed decision-making. Don't miss the opportunity to revolutionize your data strategy. Try CastorDoc today and unlock the full potential of your data for AI.
You might also like
Get in Touch to Learn More
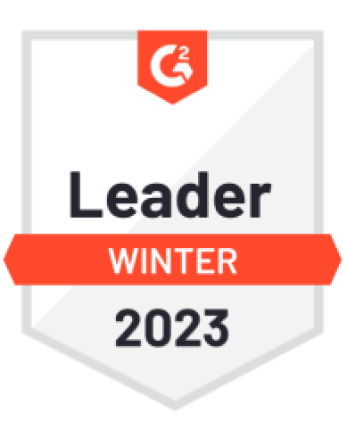
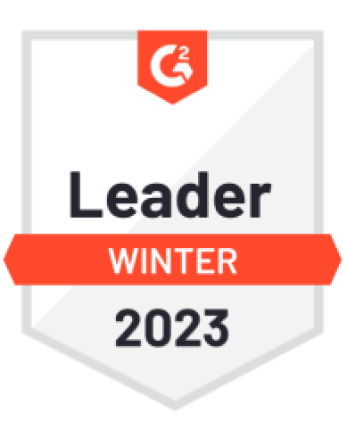

“[I like] The easy to use interface and the speed of finding the relevant assets that you're looking for in your database. I also really enjoy the score given to each table, [which] lets you prioritize the results of your queries by how often certain data is used.” - Michal P., Head of Data