Understanding the Difference Between Observability and Monitoring
Discover the nuances between observability and monitoring in this article.
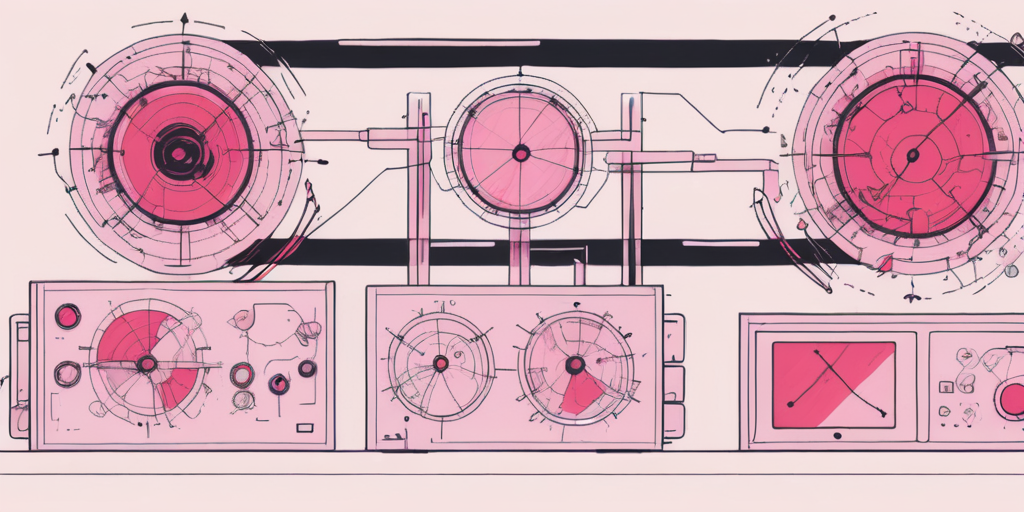
The terms observability and monitoring are often used interchangeably, but they actually refer to distinct concepts in the world of data management. Understanding the difference between them is crucial for organizations looking to optimize their data analysis and enhance decision-making processes. In this article, we will demystify the concepts of observability and monitoring, tracing their paths and exploring their significance in data management. We will also delve into the advantages of implementing observability practices and examine how observability and monitoring can work together to strengthen applications and ensure data integrity. So let's dive in and unlock the mysteries behind observability and monitoring.
Demystifying Data Monitoring
The Importance of Data Monitoring in Today's Tech Landscape
Data monitoring plays a pivotal role in today's rapidly evolving tech landscape. It involves the systematic collection and analysis of data to ensure the performance, availability, and reliability of systems, applications, and networks. With the exponential growth of data and the increasing complexity of technological infrastructures, effective data monitoring has become paramount for organizations.
By closely monitoring data, businesses can proactively identify and address potential issues, such as performance bottlenecks, security vulnerabilities, and operational risks. This allows them to maintain optimal performance, prevent downtime, and ultimately deliver a seamless user experience.
But what exactly does effective data monitoring entail? Let's dive deeper into the tools and techniques that organizations use to achieve this.
Tools and Techniques for Effective Data Monitoring
To achieve effective data monitoring, organizations leverage a variety of tools and techniques. These include real-time monitoring systems, log analyzers, performance metrics dashboards, and anomaly detection algorithms. These tools provide valuable insights into the health and performance of systems, enabling organizations to quickly identify and resolve issues before they escalate.
Real-time monitoring systems continuously collect and analyze data from various sources, providing organizations with up-to-the-minute information about the state of their systems. Log analyzers, on the other hand, help organizations make sense of the vast amount of log data generated by their systems, identifying patterns and anomalies that may indicate potential problems.
Performance metrics dashboards offer a visual representation of key performance indicators, allowing organizations to monitor the health of their systems at a glance. These dashboards provide real-time updates on metrics such as CPU usage, memory utilization, network latency, and response times, enabling organizations to quickly spot any abnormalities and take appropriate action.
Additionally, organizations employ proactive monitoring strategies, such as threshold-based monitoring and trend analysis, to detect patterns and identify deviations from normal behavior. By setting thresholds and establishing baseline performance metrics, organizations can define acceptable ranges of operation and trigger alerts when anomalies occur, enabling prompt action and minimizing potential impact.
By combining these tools and techniques, organizations can ensure that their data monitoring efforts are comprehensive and effective, enabling them to stay ahead of potential issues and maintain the highest level of performance and reliability.
Tracing the Path of Observability in Data Management
The Significance of Observability in Data Analysis
Observability is a concept that has gained significant traction in the field of data analysis. It refers to the ability to measure, understand, and debug complex systems by observing their internal states, behaviors, and interactions. Observability goes beyond traditional monitoring approaches by focusing on gaining insights into the inner workings of systems rather than solely relying on external observations.
With the exponential growth of data and the increasing complexity of modern systems, organizations require deeper visibility into system behavior to uncover inefficiencies, identify bottlenecks, and optimize performance. Observability equips organizations with the necessary tools and techniques to analyze data at scale, enabling them to make informed decisions and drive business growth.
Enhancing Data Insights Through Observability
Observability enhances data insights by providing organizations with a holistic view of system behavior and performance. By instrumenting systems with continuous monitoring and capturing granular metrics, organizations gain access to rich data streams that can be analyzed to derive valuable insights. These insights, in turn, help organizations identify optimization opportunities, enhance system performance, and drive informed decision-making.
Additionally, observability enables organizations to identify and understand the impact of external factors and interactions on system behavior. By capturing contextual data, such as user interactions, external service dependencies, and environmental conditions, organizations can gain a comprehensive understanding of system behavior and assess its impact on business outcomes.
Decoding Data Observability
Unpacking the Core Concepts of Data Observability
Data observability revolves around three core concepts: telemetry, analytics, and actionable insights. Telemetry refers to the process of collecting and aggregating data from various sources, such as logs, metrics, events, and traces. Analytics involves the analysis of telemetry data to derive meaningful insights and identify patterns or anomalies. Finally, actionable insights empower organizations to take proactive measures and optimize system performance based on the derived insights.
Organizations can achieve data observability by adopting best practices and utilizing specialized tools and techniques. These include distributed tracing frameworks, log aggregation platforms, anomaly detection algorithms, and machine learning models. By embracing data observability practices, organizations can unlock deeper insights into their systems and achieve superior performance and reliability.
Advantages of Implementing Data Observability Practices
Implementing data observability practices offers numerous advantages for organizations. Firstly, it enables them to gain a deep understanding of system behavior, identifying potential bottlenecks and improving overall performance. Secondly, data observability provides organizations with operational and business insights, empowering them to make data-driven decisions and fuel innovation. Lastly, data observability enhances collaboration and problem-solving within organizations by enabling stakeholders to share a common understanding of system behavior and work together to resolve issues promptly.
Navigating the Complexities of Observability and Monitoring
Understanding the Interplay Between Observability and Monitoring
While observability and monitoring are distinct concepts, they are not mutually exclusive. In fact, they complement each other and can work synergistically to provide organizations with comprehensive insights into system behavior and performance.
Monitoring focuses on collecting data from external sources, such as system logs, metrics, and events, to track system health and performance. Observability, on the other hand, delves deeper by capturing internal system states and behaviors, allowing organizations to gain a holistic understanding of system dynamics.
By combining the power of monitoring and observability, organizations can leverage a comprehensive set of data to optimize system performance, diagnose issues effectively, and ensure the delivery of high-quality services to end-users.
Leveraging Both for Optimal Data Management
Leveraging both observability and monitoring practices is crucial for optimal data management. Organizations can start by implementing robust monitoring solutions to capture essential data points and track system performance. This includes real-time monitoring, log analysis, and performance metrics tracking.
Once a strong monitoring foundation is established, organizations can augment their capabilities by embracing observability practices. This involves instrumenting systems to capture comprehensive telemetry data, leveraging distributed tracing frameworks, and analyzing data to gain actionable insights.
By combining the strengths of both observability and monitoring, organizations can drive continuous improvement, enhance decision-making, and ensure the effective management of their data infrastructure.
Strengthening Applications with Data Observability and Monitoring
Best Practices for Integrating Observability and Monitoring in App Development
Integrating observability and monitoring practices into application development is essential for building robust and reliable software. Organizations can start by adopting a proactive approach to monitoring, employing real-time monitoring systems, and leveraging cloud-based solutions for scalability and flexibility.
Furthermore, data observability can be integrated into the application development process by leveraging distributed tracing frameworks, setting up performance dashboards, and implementing log aggregation platforms to capture granular system data.
By integrating observability and monitoring practices into application development, organizations can ensure that their applications are built with resilience and performance in mind, guaranteeing a seamless user experience.
Ensuring Data Integrity Through Robust Observability Measures
Data integrity is paramount for organizations, and robust observability measures play a vital role in ensuring the accuracy and reliability of data. By implementing comprehensive telemetry practices, including log analysis, metrics tracking, and distributed tracing, organizations can ensure the integrity of their data.
In addition, implementing anomaly detection algorithms and applying machine learning techniques can further enhance data integrity by identifying abnormal system behavior and potential data inconsistencies.
Ensuring data integrity through robust observability measures instills trust in the data-driven decision-making process, enabling organizations to rely on accurate data to drive business growth.
The Essential Guide to Modern Data Catalogs
How Data Catalogs Streamline Information Management
Data catalogs have emerged as powerful tools in the world of information management. They streamline the process of discovering, organizing, and accessing data assets within an organization. By providing a centralized repository of metadata and data lineage information, data catalogs empower organizations to efficiently manage their data assets.
With the growing volume and complexity of data, organizations need effective solutions to locate and understand their data assets. Data catalogs provide a comprehensive view of data, enabling organizations to find relevant data sets, understand their structure and dependencies, and accelerate the data discovery process.
In conclusion, understanding the difference between observability and monitoring is crucial for organizations seeking to optimize their data management practices. Both concepts have unique roles to play in unlocking valuable insights, enhancing system performance, and driving data-driven decision-making. By embracing observability and monitoring, organizations can navigate the complexities of data management effectively and strengthen their applications while ensuring data integrity. Additionally, the implementation of data catalogs streamlines information management and enables organizations to efficiently locate and harness the power of their data assets. Embracing these concepts and tools will empower organizations to extract actionable insights from their data and gain a competitive edge in today's data-driven world.
Ready to elevate your organization's data management to the next level? CastorDoc is here to transform the way you approach observability, monitoring, and data governance. With our advanced cataloging, lineage capabilities, and user-friendly AI assistant, CastorDoc stands as a powerful ally in enabling self-service analytics. Take control of your data governance lifecycle, ensure compliance, and empower your business users to harness the full potential of your data assets. Try CastorDoc today and join the revolution in data-driven decision-making.
You might also like
Get in Touch to Learn More
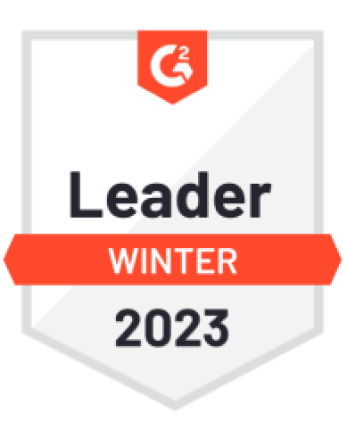
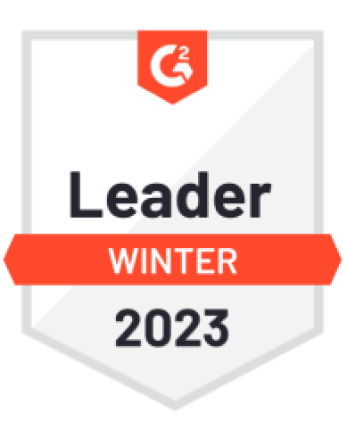

“[I like] The easy to use interface and the speed of finding the relevant assets that you're looking for in your database. I also really enjoy the score given to each table, [which] lets you prioritize the results of your queries by how often certain data is used.” - Michal P., Head of Data