What is Bad Data and How to Manage It in 2024
Discover the ins and outs of bad data and learn effective strategies to manage it in 2024.
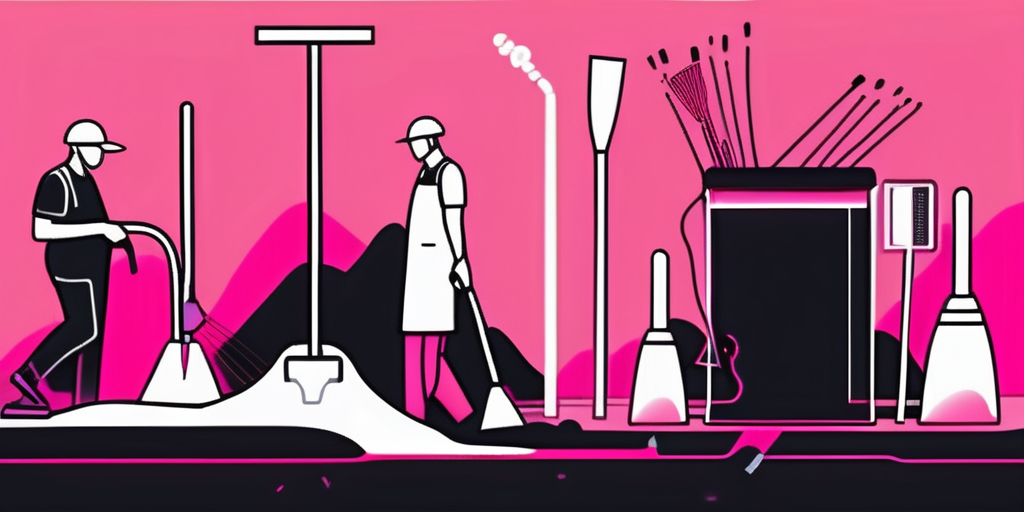
In the world of data management, bad data has become a hindrance to businesses seeking to extract valuable insights and make informed decisions. As we enter 2024, the need to understand and effectively manage bad data has become even more crucial. This article delves into the concept of bad data, its sources, and its impact on businesses. We'll also explore the evolving landscape of data management, strategies for identifying and managing bad data, as well as the challenges that organizations face in this area.
Understanding the Concept of Bad Data
Before delving into the complexities of bad data management, it is essential to understand what bad data truly entails. Bad data refers to any information that is inaccurate, inconsistent, incomplete, or unreliable. It can be generated internally or sourced from external data providers, leading to a multitude of potential issues.
Defining Bad Data
Bad data can manifest in different ways, including missing or incorrect values, duplicates, outdated records, or inconsistent formats. It can occur at various stages of the data lifecycle, from collection to storage and analysis. All of these factors contribute to the deteriorating quality of data, making it challenging to derive meaningful insights.
Common Sources of Bad Data
There are several common sources of bad data that organizations must be aware of:
- Inaccurate Data Entry: Human error during data entry can lead to typos, misspellings, or entering incorrect values.
- Outdated Data: As time passes, data can become outdated or irrelevant, especially in fast-paced industries.
- Integration Issues: When combining data from different sources or systems, inconsistencies and conflicts can arise, leading to bad data.
- Data Migration: During the process of migrating data from one system to another, errors can be introduced, impacting data quality.
- External Data Sources: Third-party data providers may supply incomplete or incorrect information, further contributing to bad data.
The Impact of Bad Data on Businesses
The impact of bad data on businesses is far-reaching and can affect different areas of operations:
- Decision Making: Inaccurate or incomplete data can lead to poor decision making by executives and hinder strategic planning.
- Customer Experience: Bad data can result in incorrect customer information, leading to poor customer service and decreased satisfaction levels.
- Operational Efficiency: Inaccurate data can cause delays, errors, and inefficiencies in business operations, leading to wasted resources.
- Regulatory Compliance: Data quality issues can lead to non-compliance with industry regulations, resulting in legal and financial consequences.
Furthermore, bad data can also have a significant impact on marketing efforts. For instance, if a company's marketing team relies on inaccurate data to target their audience, they may end up wasting resources on ineffective campaigns. This can not only lead to a loss of revenue but also damage the company's reputation.
In addition, bad data can hinder the ability to identify trends and patterns, making it difficult to gain valuable insights into customer behavior or market trends. This lack of understanding can put a company at a disadvantage when it comes to making informed business decisions or staying ahead of the competition.
Moreover, bad data can have serious implications for data-driven industries such as healthcare or finance. Inaccurate or unreliable data in these sectors can lead to incorrect diagnoses, financial losses, or even compromised patient safety.
The Evolution of Data Management
Data management practices have undergone a significant transformation in recent years, driven by advancements in technology and changing business needs.
In the past, data management was often approached in an ad-hoc manner, with organizations relying on manual processes and outdated systems. However, as the volume and complexity of data have increased, organizations have recognized the need for a more comprehensive and proactive approach.
The Shift in Data Management Practices
Organizations are now moving away from traditional, ad-hoc approaches to data management, towards more comprehensive and proactive strategies. This shift involves:
- Data Governance: Implementing robust data governance frameworks to ensure data quality, privacy, and compliance. This includes defining clear roles and responsibilities, establishing data standards, and implementing processes for data stewardship.
- Data Integration: Establishing streamlined processes for integrating and harmonizing data from various sources. This involves leveraging technologies such as ETL (Extract, Transform, Load) tools and data integration platforms to ensure data consistency and accuracy.
- Master Data Management: Employing MDM solutions to consolidate and maintain a single, authoritative view of data. This involves creating a centralized repository for master data, implementing data validation rules, and establishing data governance policies.
- Data Quality Management: Implementing processes and tools for ensuring data accuracy, consistency, and completeness. This includes data profiling, data cleansing, and data quality monitoring to identify and resolve data quality issues.
By adopting these practices, organizations can improve the reliability and usability of their data, enabling better decision-making and driving business growth.
Future Trends in Data Management
The future of data management is poised for exciting developments:
- Increased Automation: Artificial intelligence and machine learning algorithms will play a vital role in automating data management tasks and improving data quality. These technologies can analyze large volumes of data, identify patterns, and make data-driven recommendations, reducing the need for manual intervention.
- Data Privacy and Security: As data breaches become more prevalent, organizations will focus on enhancing data privacy and security measures. This includes implementing robust encryption techniques, adopting multi-factor authentication, and establishing strict access controls to protect sensitive data.
- Real-time Data Analytics: The need for real-time data insights will continue to grow, driving the adoption of advanced analytics tools and platforms. Organizations will leverage technologies such as in-memory computing and real-time data streaming to analyze data as it is generated, enabling faster and more accurate decision-making.
As technology continues to evolve, data management practices will continue to adapt and improve. Organizations that embrace these advancements will be better positioned to harness the power of data and gain a competitive edge in today's data-driven world.
Identifying Bad Data in Your System
Recognizing the presence of bad data within your system is the first step towards effective management. Here are some signs to watch out for:
Signs of Bad Data
Common indicators of bad data include:
- Consistently incorrect or inconsistent values appearing in reports or analytics.
- Unexpected data patterns or outliers that deviate from the expected distributions.
- Frequent data quality issues identified during data validation processes.
- Low data accuracy scores or metrics falling below acceptable thresholds.
Tools for Detecting Bad Data
A range of tools and technologies are available to help organizations identify and flag bad data:
- Data Quality Assessment Tools: These tools assess data quality based on predefined rules and provide reports highlighting potential issues.
- Data Profiling Tools: Data profiling tools analyze the content, structure, and relationships within datasets, identifying anomalies and inconsistencies.
- Data Cleansing Tools: Data cleansing tools automate the process of correcting or removing bad data, ensuring data integrity.
Strategies for Managing Bad Data in 2024
To effectively manage bad data and mitigate its impact, organizations must adopt comprehensive strategies. The following approaches can be instrumental:
Implementing Data Quality Measures
Formulating and implementing data quality measures involves establishing data quality rules, conducting regular audits, and monitoring data quality metrics. This ensures that data adheres to defined standards and meets the organization's requirements.
Leveraging Artificial Intelligence for Data Management
Artificial intelligence (AI) technologies, such as machine learning algorithms, can automate data management tasks, improving efficiency and accuracy. AI can be utilized for data cleansing, data validation, and anomaly detection, reducing reliance on manual intervention.
The Role of Data Governance in Controlling Bad Data
Data governance frameworks play a critical role in enforcing data quality standards and establishing processes for identifying and rectifying bad data. By implementing robust data governance practices, organizations can cultivate a culture of data responsibility and accountability.
Overcoming Challenges in Bad Data Management
While organizations strive to manage bad data effectively, they encounter several challenges along the way:
Dealing with Large Volumes of Data
As data volumes continue to grow exponentially, organizations must grapple with the sheer magnitude of data they need to manage. Scalable infrastructure, advanced analytics tools, and effective data processing techniques are essential to overcome this challenge.
Ensuring Data Privacy and Security
In the era of stringent data protection regulations and increasing cyber threats, organizations must prioritize data privacy and security. Implementing robust data security protocols, encryption methods, and access controls helps safeguard data from unauthorized access and misuse.
Addressing the Skills Gap in Data Management
With the rapidly evolving landscape of data management, organizations face a shortage of skilled professionals who can effectively navigate complex data environments. Investing in training and development programs can bridge this skills gap and equip employees with the knowledge and expertise required for effective bad data management.
In conclusion, bad data poses significant challenges to organizations seeking to derive meaningful insights and make informed decisions. By understanding the concept of bad data, adopting evolving data management practices, implementing strategies for identifying and managing bad data, and addressing associated challenges, organizations can pave the way towards effective data-driven decision making in 2024 and beyond.
You might also like
Get in Touch to Learn More
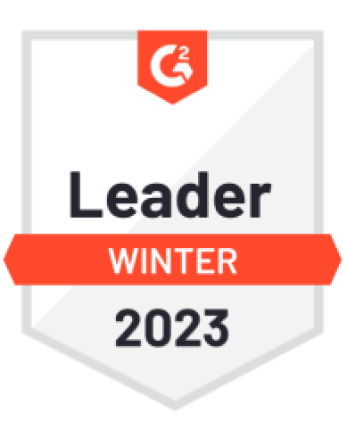
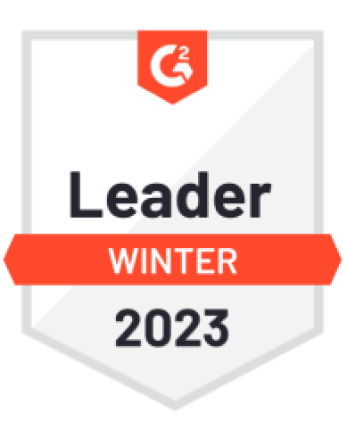

“[I like] The easy to use interface and the speed of finding the relevant assets that you're looking for in your database. I also really enjoy the score given to each table, [which] lets you prioritize the results of your queries by how often certain data is used.” - Michal P., Head of Data