What is Data Lake Metadata Management? Benefits, Examples, Tools, & Key Factors to Consider
Unlock the power of data lake metadata management with this article.
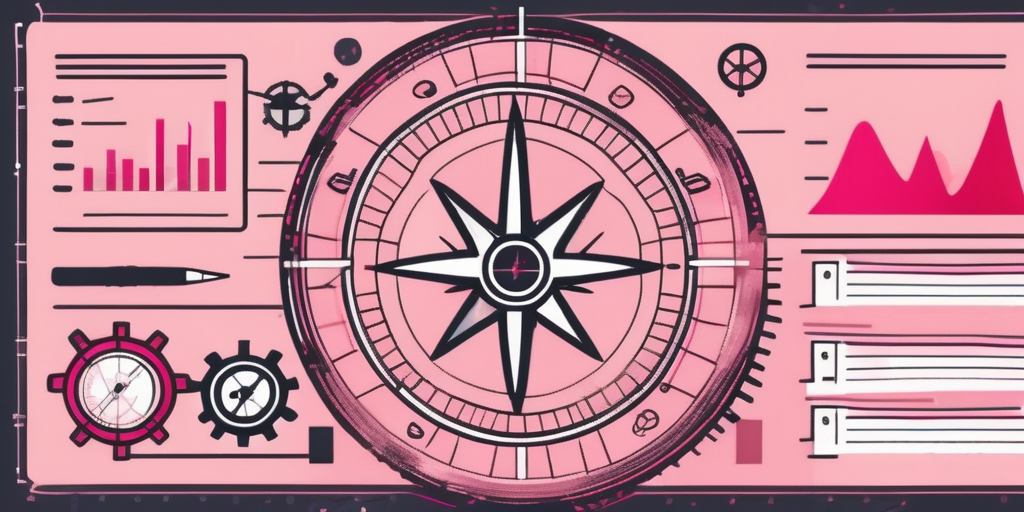
Data Lake Metadata Management is a crucial aspect of managing and organizing data within a data lake environment. In this article, we will explore the definition and importance of Data Lake Metadata Management, its advantages, various tools available for managing metadata, and key factors to consider when implementing this practice. Additionally, we will discuss future trends in Data Lake Metadata Management.
Understanding Data Lake Metadata Management
Definition of Data Lake Metadata Management
Data Lake Metadata Management refers to the practice of capturing, organizing, and maintaining metadata within a data lake. Metadata is descriptive information that provides context and understanding about the data contained within the data lake. It includes details such as data source, data quality, data lineage, data ownership, and other relevant information.
Effective Metadata Management ensures that data is well-documented, easily discoverable, and properly governed, enabling organizations to make informed decisions based on the data available in their data lakes.
Implementing a robust metadata management strategy involves the use of metadata repositories, data catalogs, and metadata tagging mechanisms. Metadata repositories store metadata in a structured format, making it accessible for data governance and analysis purposes. Data catalogs provide a centralized inventory of metadata assets, allowing users to search, browse, and understand the available data resources. Metadata tagging involves assigning metadata tags to data assets, making it easier to classify and retrieve information based on specific attributes.
The Role of Metadata in a Data Lake
In a data lake, metadata plays a crucial role in enabling organizations to effectively store, manage, and analyze vast amounts of data. It provides insights into the structure, format, and contextual information of the data. Metadata acts as a guide for data discovery, ensuring that users can locate and understand relevant data assets.
Metadata also facilitates data lineage, allowing users to track the origin and transformation of data within the data lake. By providing visibility into data quality, metadata helps in ensuring data consistency and reliability for analysis and decision-making.
Furthermore, metadata management supports data governance initiatives by establishing policies and procedures for metadata creation, maintenance, and usage. It helps organizations comply with regulatory requirements, enhance data security, and improve data quality. Metadata management also aids in data integration efforts by mapping data relationships and dependencies across different data sources, enabling seamless data flow and interoperability.
The Advantages of Data Lake Metadata Management
Improving Data Quality and Consistency
Metadata Management plays a crucial role in improving data quality and consistency within a data lake. By documenting data definitions, data types, and data validation rules, organizations can enforce consistent data standards. This reduces errors, enhances data accuracy, and ensures that users can trust the data available in the data lake for their analysis and decision-making processes.
Enhancing Data Security and Compliance
Data Lake Metadata Management enables organizations to maintain better control over data security and compliance. Metadata can include information about data access permissions, data sensitivity, and data governance policies. With this information, organizations can enforce access controls, monitor data usage, and ensure compliance with privacy regulations and security standards.
Facilitating Data Discovery and Analysis
Metadata plays a vital role in facilitating data discovery and analysis within a data lake. By capturing and indexing metadata, organizations can provide users with comprehensive search capabilities, enabling them to find relevant data assets quickly and efficiently. This enhances productivity and enables users to make more informed decisions based on the available data.
Furthermore, metadata provides context to the data, allowing users to understand the various data sources, transformations, and relationships involved. This enhances the accuracy and effectiveness of data analysis, enabling organizations to uncover valuable insights and gain a competitive advantage.
Moreover, metadata management also enables organizations to track the lineage of data within the data lake. By capturing information about the origin, transformation, and usage of data, organizations can establish a clear lineage trail. This lineage information is valuable for compliance purposes, as it allows organizations to demonstrate data provenance and ensure regulatory compliance.
Additionally, metadata management can help organizations optimize data storage and retrieval within the data lake. By analyzing metadata, organizations can identify data redundancy and eliminate unnecessary duplication. This not only reduces storage costs but also improves data retrieval performance, as users can access the most relevant and up-to-date data without sifting through redundant copies.
Tools for Data Lake Metadata Management
Overview of Metadata Management Tools
Several tools are available to assist organizations in managing metadata within their data lakes. These tools play a crucial role in ensuring that metadata is effectively captured, stored, visualized, and governed within the data lake environment. By utilizing these tools, organizations can gain valuable insights into their data assets, improve data quality, and enhance data governance practices.
Informatica Axon is a popular Metadata Management tool known for its robust capabilities in data governance and metadata management. It offers features such as data lineage tracking, metadata visualization, and business glossary management. Collibra is another widely used tool that focuses on data governance and data cataloging. It enables organizations to establish data governance policies, maintain data quality, and ensure regulatory compliance within their data lake infrastructure.
Selecting the Right Tool for Your Needs
When selecting a Metadata Management tool for your organization's data lake, it is essential to conduct a thorough evaluation of the available options to determine the best fit for your specific requirements. Consider factors such as scalability, performance, ease of use, and integration capabilities with your existing data platforms. Additionally, assess the tool's support for industry standards such as Apache Atlas for metadata management in Hadoop environments.
Furthermore, it is crucial to involve key stakeholders from various departments in the selection process to gather diverse perspectives and ensure that the chosen tool aligns with the organization's overall goals and objectives. By engaging IT professionals, data governance experts, and business users in the decision-making process, you can identify the tool that best meets your organization's metadata management needs and sets the foundation for effective data governance practices.
Key Factors to Consider in Data Lake Metadata Management
Data Governance Policies
Data governance policies play a critical role in Data Lake Metadata Management. Organizations must establish clear guidelines and governance processes to ensure consistent metadata capture, maintenance, and usage. Such policies should cover metadata standards, ownership, access controls, and data lifecycle management. It is crucial to involve all relevant stakeholders in defining these policies to ensure compliance, transparency, and data integrity.
Furthermore, data governance policies should also address data quality and security aspects within the data lake environment. Implementing robust data quality controls ensures that the metadata remains accurate, reliable, and up to date. Simultaneously, incorporating stringent security measures safeguards sensitive information from unauthorized access or breaches, thereby maintaining the overall integrity of the data lake ecosystem.
Scalability and Performance
As the volume and variety of data within a data lake continue to grow, scalability becomes a crucial consideration in Metadata Management. The selected Metadata Management tool should be capable of handling large-scale metadata ingestion, search, and retrieval efficiently. It should also be able to scale seamlessly as the data lake expands, without compromising performance.
In addition to scalability, performance optimization is key in ensuring that metadata operations do not hinder the overall data processing speed and analytics capabilities of the data lake. Efficient indexing, caching mechanisms, and distributed processing techniques can significantly enhance the performance of Metadata Management operations, enabling faster access to critical metadata information for various data lake users and applications.
Integration with Existing Systems
Integration with existing data platforms, data governance frameworks, and data management systems is another factor to consider in Data Lake Metadata Management. The chosen Metadata Management tool should be compatible with the technology stack in use within the organization. Seamless integration enables smooth data flow, reduces duplication of efforts, and enhances overall efficiency.
Moreover, interoperability with other metadata repositories and tools across the organization's IT landscape is essential for facilitating data sharing, collaboration, and cross-functional analytics initiatives. By ensuring seamless integration with existing systems, Data Lake Metadata Management can streamline metadata workflows, promote data reuse, and foster a more cohesive data ecosystem within the organization.
Future Trends in Data Lake Metadata Management
The Rise of AI in Metadata Management
The future of Data Lake Metadata Management is likely to be influenced significantly by artificial intelligence (AI) technologies. AI-powered metadata management promises advanced capabilities such as automated metadata discovery, data tagging, and metadata lineage inference. These advancements will streamline metadata management processes and reduce manual effort, enabling organizations to leverage their data lakes more effectively.
The Impact of Cloud-Based Solutions
Cloud-based solutions are expected to play a significant role in the future of Data Lake Metadata Management. Cloud platforms offer scalability, flexibility, and cost-effectiveness in managing metadata across large-scale data lakes. Additionally, cloud-based solutions provide seamless integration with various data platforms and enable cross-organizational collaboration. The adoption of cloud-based Metadata Management solutions is likely to increase as organizations strive to leverage the benefits of cloud technology in their data lake environments.
In conclusion, Data Lake Metadata Management is a critical practice for managing and organizing data within a data lake. It offers numerous benefits, including improved data quality, enhanced data security, and facilitated data discovery and analysis. Various Metadata Management tools are available to assist organizations in managing metadata effectively. When implementing Data Lake Metadata Management, organizations must consider key factors such as data governance policies, scalability, and integration with existing systems. Looking ahead, AI-powered metadata management and cloud-based solutions are expected to shape the future of Data Lake Metadata Management, revolutionizing the way organizations manage and leverage their data assets.You might also like
Get in Touch to Learn More
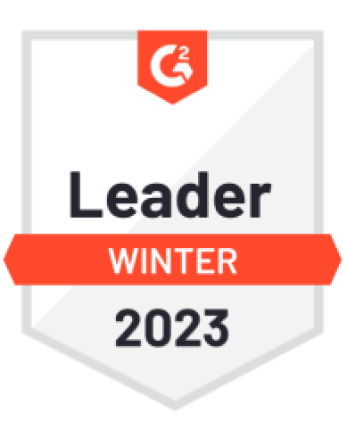
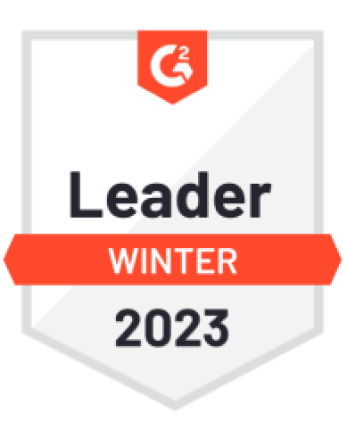

“[I like] The easy to use interface and the speed of finding the relevant assets that you're looking for in your database. I also really enjoy the score given to each table, [which] lets you prioritize the results of your queries by how often certain data is used.” - Michal P., Head of Data