What is Data Quality? Dimensions & Their Measurement
Explore the essential concept of data quality, uncovering its key dimensions and measurement techniques.
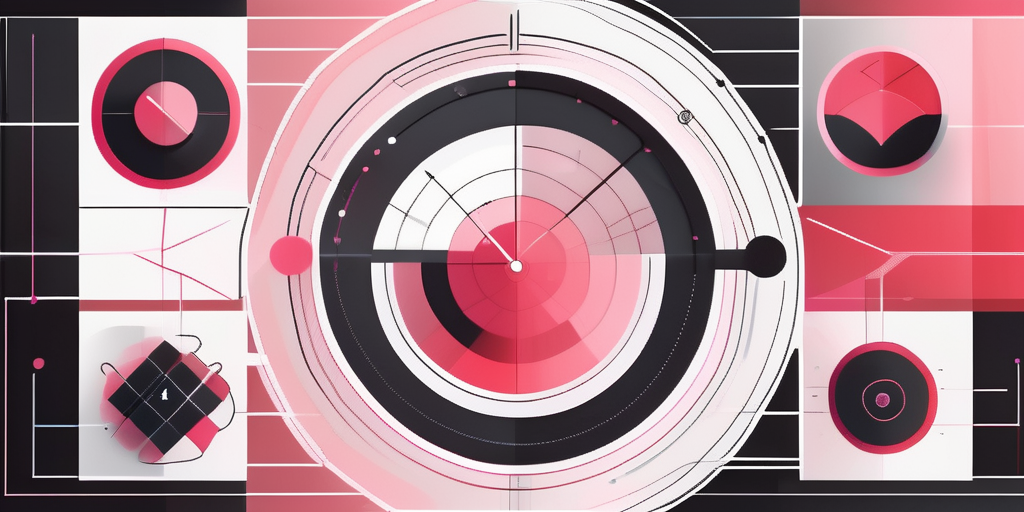
Data quality is a critical consideration in the management and utilization of data across various fields, including business, healthcare, and research. As organizations increasingly rely on data-driven decision-making, understanding the dimensions of data quality becomes essential. This article delves into the foundations of data quality, its dimensions, the importance of data governance, and methods for measurement.
Understanding the Concept of Data Quality
Data quality refers to the overall utility of a dataset’s information for a specific purpose. High-quality data is both accurate and consistent, which significantly enhances its reliability for decision-making processes. Organizations must strive to maintain data quality to ensure that they can trust their analytical insights and operational activities.
The Importance of High-Quality Data
The significance of high-quality data cannot be overstated. Data that is accurate, consistent, and complete leads to reliable insights, which ultimately promote better decision-making. Conversely, poor data quality can result in flawed analyses, misinformed strategies, and wasted resources.
For instance, in healthcare, patient records must be accurate to ensure proper treatment. In business, faulty sales data can lead to ineffective marketing strategies, ultimately harming revenue. Therefore, maintaining high standards of data quality is essential for all sectors.
Moreover, the rise of big data analytics has amplified the need for high-quality data. As organizations increasingly rely on vast amounts of data to drive their strategies, the implications of poor data quality become even more pronounced. Inaccurate data can lead to misguided business decisions, affecting everything from product development to customer satisfaction. Consequently, investing in data quality management systems has become a priority for many organizations, as they seek to harness the full potential of their data assets.
Key Characteristics of Data Quality
Several key characteristics define data quality, including accuracy, consistency, completeness, relevance, and timeliness. These characteristics help organizations assess and enhance the quality of their data. When evaluating data quality, organizations should consider the purpose of the data as different applications may prioritize certain attributes over others.
To achieve high-quality standards, organizations often establish specific metrics and benchmarks. Monitoring these characteristics helps identify deficiencies and facilitates targeted improvements. For example, an organization might implement regular data audits to ensure that its datasets remain accurate and up-to-date. Additionally, the use of automated data cleansing tools can help streamline the process of maintaining data quality, allowing teams to focus on more strategic initiatives rather than getting bogged down in manual data correction tasks.
Furthermore, fostering a data-driven culture within an organization is crucial for sustaining high data quality. When employees at all levels understand the importance of data quality and are trained in best practices for data entry and management, the likelihood of errors decreases significantly. This cultural shift not only improves the quality of data collected but also empowers employees to take ownership of the data they work with, leading to a more informed and agile organization overall.
Exploring the Dimensions of Data Quality
The dimensions of data quality provide a framework for assessing and ensuring the integrity of data. Understanding these dimensions allows organizations to systematically evaluate and enhance their data quality processes. In an era where data drives decision-making, the importance of maintaining high-quality data cannot be overstated. Organizations that prioritize data quality are better positioned to leverage insights, optimize operations, and achieve strategic objectives.
Accuracy: The Heart of Data Quality
Accuracy refers to the degree to which data correctly describes the real-world scenario it represents. High accuracy means that there are minimal errors in the data, which is crucial for making sound decisions. For example, accurate customer data ensures that marketing campaigns reach the intended audience. Moreover, inaccuracies can lead to financial losses, reputational damage, and misguided strategies that may take years to rectify.
Organizations can enhance data accuracy through validation rules, data cleansing, and continuous monitoring. Employing automated systems to check for inaccuracies can also help maintain high accuracy levels over time. Additionally, fostering a culture of accountability among employees who handle data can significantly reduce errors. Training staff on best practices for data entry and encouraging them to report discrepancies can create a more reliable data ecosystem.
Consistency: Ensuring Uniformity in Data
Consistency involves maintaining uniformity of data across different datasets. If the same data element appears in multiple places, it should have the same format and value. Inconsistent data can lead to confusion and erroneous interpretations. For instance, if customer names are recorded in different formats—some in full, others as initials—it can complicate customer relationship management and analytics.
To achieve consistency, organizations often implement data integration practices, standardization metrics, and strict data entry guidelines. Regular audits can also identify and rectify inconsistencies, ensuring that all data adheres to established standards. Additionally, leveraging data governance frameworks can help enforce consistency by defining roles, responsibilities, and processes for data management across the organization.
Completeness: Filling in the Data Gaps
Completeness measures the extent to which all required data is present within a dataset. Data gaps can result from incomplete inputs or data not being collected at all. Such deficiencies can significantly undermine analyses, leading to skewed results. For example, missing sales data can hinder accurate forecasting and inventory management, ultimately affecting customer satisfaction and business performance.
Organizations must define what constitutes complete data based on their specific requirements. Implementing checks during data entry processes and performing regular audits can help identify missing data. Additionally, engagement with data providers to ensure thorough data collection can address these issues proactively. Utilizing data enrichment services can also fill in gaps by supplementing existing datasets with additional information, thereby enhancing overall completeness.
Relevance: The Value of Pertinent Data
Relevance assesses how well data meets the needs of the task at hand. Data must be applicable, timely, and aligned with the current objectives of the organization to have value. Irrelevant data can clutter analysis, divert focus, and obscure critical insights. As organizations evolve, the relevance of certain data points may diminish, necessitating a regular review of data assets.
Assessing relevance should involve scrutinizing the purpose of data collection and usage. Ensuring that data remains relevant requires ongoing communication between data users and stakeholders, as organizational goals evolve over time. Furthermore, employing analytics tools that can filter out irrelevant data can streamline decision-making processes, allowing teams to focus on the most impactful information. By fostering a dynamic approach to data relevance, organizations can ensure they remain agile and responsive to changing market conditions and internal objectives.
The Role of Data Governance in Maintaining Data Quality
Data governance is a vital framework that establishes policies and standards for managing data across an organization, thereby playing a key role in maintaining data quality. It encompasses procedures for data handling, stewardship, and compliance, ensuring that data is managed responsibly.
Defining Data Governance
Data governance establishes the overall strategy for managing data as a valuable asset. It includes aspects such as data ownership, data responsibility, and accountability. A robust data governance framework outlines clear roles and responsibilities for data management, ensuring that quality standards are consistently applied.
The implementation of data governance involves the formation of governance councils and committees aimed at overseeing data policies and practices. This structured approach fosters a culture of data quality across the organization.
The Connection Between Data Governance and Data Quality
The relationship between data governance and data quality is reciprocal; effective governance is fundamental to achieving high data quality. Conversely, quality data enhances the frameworks that govern its use. When data governance policies are established, they serve to enforce data quality through regulatory means.
Organizations with a strong governance framework can better identify data quality issues and take corrective measures swiftly. This dynamic interplay fosters an environment conducive to maintaining continuous improvement in data quality.
Measuring Data Quality: Tools and Techniques
Measuring data quality is crucial for understanding its efficacy and identifying areas that need improvement. Various tools and techniques exist to assess different dimensions of data quality, enabling organizations to maintain high standards effectively.
Data Quality Assessment: An Overview
Data quality assessment involves the systematic evaluation of data against established standards. This process typically includes defining specific quality metrics and employing analytical tools to measure actual data quality against these benchmarks.
Organizations may employ assessment frameworks such as the Data Quality Assessment Framework (DQAF), which allows for a holistic examination of data quality across multiple dimensions. Regular assessments empower organizations to remain vigilant and proactive about data quality issues.
Techniques for Measuring Accuracy and Consistency
Techniques such as data profiling, audits, and statistical sampling are commonly used to measure accuracy and consistency. Data profiling examines datasets to understand their structure, culture, and anomalies, while audits systematically verify data against rules and standards.
Additionally, utilizing database constraints and validation rules during data entry can prevent inaccuracies from occurring in the first place, thus enhancing both accuracy and consistency over time.
Tools for Evaluating Completeness and Relevance
Tools like ETL (Extract, Transform, Load) processes often play a critical role in evaluating completeness by ensuring that all relevant data from various sources is gathered and transformed appropriately. Moreover, reporting tools and dashboards can aid in analyzing data relevance by continuously tracking performance metrics against business objectives.
Furthermore, organizations can utilize advanced analytics and machine learning algorithms to assess the value of their data consistently, ensuring that it remains aligned with evolving goals and context.
In conclusion, achieving and maintaining data quality requires a comprehensive understanding of its dimensions and dedicated efforts in governance, measurement, and continuous improvement. As organizations recognize the pivotal role of data quality, they position themselves for success in their data-driven initiatives.
Understanding and maintaining data quality is fundamental to any data-driven organization, but it can be an intricate and challenging task. This is where CastorDoc steps in, integrating advanced governance, cataloging, and lineage capabilities with a user-friendly AI assistant, creating a powerful tool for businesses to enable self-service analytics. With CastorDoc, you can ensure that your data quality meets the high standards necessary for reliable decision-making and strategic success. Whether you're a data professional looking to streamline governance and maintain data quality or a business user seeking to harness the power of data analytics, CastorDoc is your partner in navigating the complex data landscape. Try CastorDoc today and revolutionize the way your organization manages and leverages its data.
You might also like
Get in Touch to Learn More
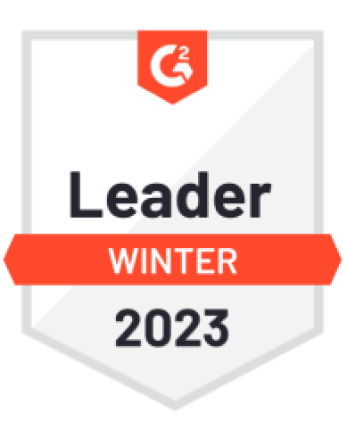
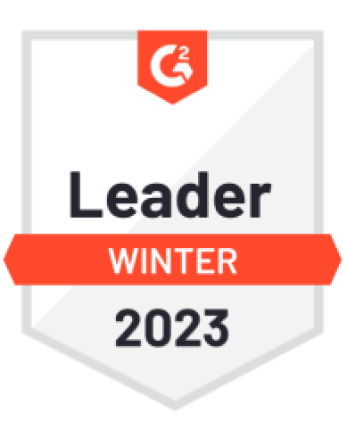

“[I like] The easy to use interface and the speed of finding the relevant assets that you're looking for in your database. I also really enjoy the score given to each table, [which] lets you prioritize the results of your queries by how often certain data is used.” - Michal P., Head of Data