What is Data Reliability? Examples, How to Measure & Ensure!
Discover the importance of data reliability and learn how to measure and ensure it in your organization.

Data reliability is a critical aspect of data quality that organizations and researchers must ensure. In an era where data is king, relying on inaccurate or inconsistent data can lead to incorrect analysis and flawed decision-making. Therefore, understanding and measuring data reliability are paramount to ensure the integrity and trustworthiness of the data used in various fields. This article aims to provide an in-depth understanding of data reliability, its components, measurement techniques, and strategies to enhance its reliability.
Understanding the Concept of Data Reliability
Data reliability refers to the degree of trust and confidence that data users can place in the accuracy, consistency, and stability of the data. It involves assessing the extent to which data accurately represents the real-world phenomena it aims to capture. By ensuring data reliability, users can have confidence that the data can be used consistently over time and across different contexts.
Defining Data Reliability
Data reliability can be defined as the extent to which data accurately represents the underlying reality it aims to describe. It goes beyond mere accuracy and encompasses consistency, stability, and absence of bias. Reliable data should provide consistent results when measured repeatedly, and it should withstand scrutiny in various contexts and time periods.
Importance of Data Reliability in Various Fields
Data reliability plays a crucial role in numerous fields, such as scientific research, business analytics, healthcare, and environmental studies, among others. In scientific research, reliable data is vital for drawing accurate conclusions and making informed decisions. Similarly, businesses heavily rely on reliable data to identify trends, forecast demand, and optimize operations. In healthcare, reliable data ensures accurate patient diagnoses and effective treatment plans. Additionally, environmental studies heavily rely on reliable data to monitor climate change, assess the impact of human activities, and develop sustainable solutions.
Let's delve deeper into the importance of data reliability in scientific research. In this field, data reliability is the cornerstone of any study. Researchers spend countless hours collecting and analyzing data to draw meaningful conclusions. Without reliable data, their efforts would be in vain. Imagine a scenario where a scientist is studying the effects of a new drug on a specific disease. If the data collected is not reliable, the conclusions drawn from the study could be inaccurate, leading to potentially harmful consequences for patients.
Similarly, in the business world, data reliability is essential for making informed decisions. Companies rely on data to identify market trends, understand customer behavior, and optimize their operations. Without reliable data, businesses would be operating blindly, making decisions based on inaccurate or inconsistent information. This could result in missed opportunities, poor customer satisfaction, and ultimately, financial losses.
In the healthcare industry, data reliability is crucial for accurate patient diagnoses and effective treatment plans. Doctors and healthcare professionals heavily rely on data to make critical decisions about patient care. From medical tests to patient histories, reliable data ensures that healthcare providers have the necessary information to provide the best possible care. Without data reliability, misdiagnoses and ineffective treatments could occur, jeopardizing patient health and well-being.
Lastly, in environmental studies, data reliability is paramount for monitoring climate change and assessing the impact of human activities. Scientists collect data on various environmental factors, such as temperature, air quality, and biodiversity, to understand the state of our planet. Reliable data is essential to accurately measure and analyze these factors, enabling scientists to develop effective strategies to mitigate the effects of climate change and protect our environment.
In conclusion, data reliability is of utmost importance in various fields. It ensures that the data accurately represents the real-world phenomena it aims to capture and can be used consistently over time and across different contexts. Whether in scientific research, business analytics, healthcare, or environmental studies, reliable data is the foundation for making informed decisions and driving meaningful change.
Components of Data Reliability
Data reliability consists of several interconnected components that contribute to the overall trustworthiness of the data. These components include consistency in data, stability of data over time, and accuracy in data representation.
Accuracy in Data Representation
Accuracy in data representation is crucial for ensuring that the data reflects the true values and characteristics of the phenomena being measured. It involves minimizing errors and biases in data collection, processing, and reporting. Accurate data representation is essential for making informed decisions and drawing reliable conclusions based on the data.
Consistency in Data
Consistency in data refers to the absence of variations or contradictions within the dataset. Consistent data should provide similar results when measured repeatedly under similar conditions. Inconsistencies may arise due to measurement errors, human errors during data entry, data collection biases, or data integration issues. Ensuring data consistency involves rigorous quality control measures throughout the data lifecycle, including data collection, storage, and analysis.
Stability of Data Over Time
The stability of data over time refers to its ability to maintain its reliability across different time periods. Reliable data should reflect consistent patterns and trends across multiple data collection points. Stability is particularly important when analyzing longitudinal data or comparing data from different time periods. To ensure data stability, organizations should establish standardized data collection methods and regularly update and validate data sources to account for changes in the environment or underlying phenomena.
Enhancing data reliability requires a comprehensive approach that addresses all these components to ensure that the data is accurate, consistent, and stable over time. Organizations must invest in robust data management practices and quality assurance processes to uphold the integrity and reliability of their data assets.
Measuring Data Reliability
Measuring data reliability is essential to assess the quality and trustworthiness of the data. Various tools and metrics can be employed to measure data reliability effectively.
Tools for Measuring Data Reliability
There are several tools available to measure data reliability, such as statistical methods, data quality assessment frameworks, and third-party data audit services. Statistical methods, including inter-rater reliability, test-retest reliability, and internal consistency measures, assess the agreement or consistency between different measurements or data sources. Data quality assessment frameworks, such as the Total Data Quality Management framework, help organizations evaluate multiple dimensions of data quality, including reliability. Third-party data audit services can provide independent assessments and recommendations to improve data reliability.
Common Metrics in Data Reliability
Common metrics used to measure data reliability include measures of accuracy, coherence, and completeness of the data. Accuracy measures assess the degree of error or deviation between the collected data and the true value it aims to represent. Coherence measures evaluate the logical consistency and absence of contradictions or outliers in the dataset. Completeness measures determine the extent to which the data includes all relevant variables and records required for analysis or decision-making.
Ensuring Data Reliability
Ensuring data reliability requires organizations and researchers to implement best practices in data collection, verification, and validation.
Best Practices for Data Collection
Implementing robust data collection practices is crucial to ensure data reliability. Organizations should define clear data collection protocols, including standardized data collection forms, explicit definitions of variables, and rigorous training for data collectors. Data collection processes should include appropriate validation mechanisms, such as cross-checking data with multiple sources or conducting pilot studies to identify and rectify potential errors or biases.
Data Verification and Validation Techniques
Data verification and validation are essential steps to ensure the accuracy and reliability of the collected data. Verification involves checking the integrity and consistency of the data during the data entry process. Validation, on the other hand, goes beyond verification and aims to establish the accuracy and reliability of the data by comparing it with external sources or conducting internal consistency checks. Employing techniques such as double data entry, independent data audits, or data triangulation can enhance data verification and validation processes.
Challenges in Maintaining Data Reliability
Maintaining data reliability poses various challenges that organizations and researchers must overcome.
Dealing with Incomplete Data
Incomplete data, where certain variables or records are missing, presents a challenge to data reliability. Missing data can introduce biases and impact the accuracy and representativeness of the analysis. To address this challenge, organizations should implement strategies such as imputation techniques, sensitivity analyses, or carefully consider the limitations and potential biases associated with incomplete data.
Overcoming Bias in Data Collection
Bias in data collection can significantly undermine data reliability. Biases can arise due to non-random sampling methods, selective reporting, or subjective data interpretation. To overcome bias, organizations should employ random sampling techniques, implement standardized data collection protocols, and carefully consider potential sources of bias during data analysis. Additionally, transparency in reporting methods and robust peer review processes can help identify and mitigate biases.
In conclusion, data reliability is of utmost importance in ensuring accurate analysis and informed decision-making in various fields. By understanding and measuring data reliability, organizations and researchers can enhance the trustworthiness and usability of the data they rely on. Consistency, stability, and accuracy are key components of data reliability, which can be measured using various tools and metrics. Implementing best practices in data collection, verification, and validation is crucial to enhance data reliability. Despite the challenges posed by incomplete data and biases, organizations can overcome these obstacles through careful consideration and adoption of appropriate techniques and methodologies. Ultimately, ensuring data reliability is paramount for organizations and researchers to maximize the value and impact of their data-driven endeavors.You might also like
Get in Touch to Learn More
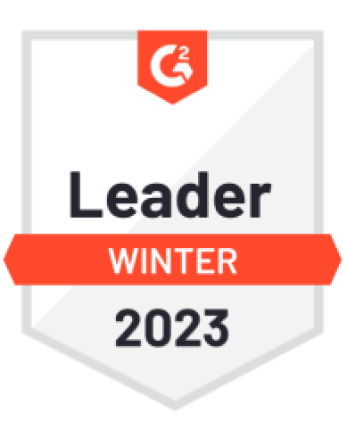
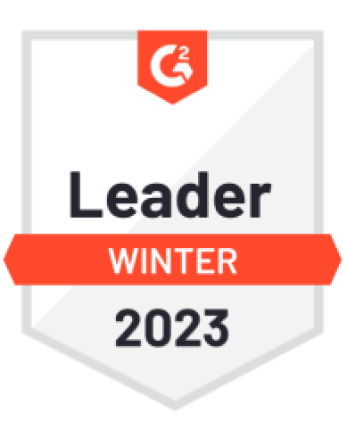

“[I like] The easy to use interface and the speed of finding the relevant assets that you're looking for in your database. I also really enjoy the score given to each table, [which] lets you prioritize the results of your queries by how often certain data is used.” - Michal P., Head of Data