Data Observability Tool Comparison: Datafold vs. Anomalo
Data observability has become a critical aspect of data management in today's digital landscape. With the increasing complexity and scale of data operations, organizations need reliable tools to ensure the quality and reliability of their data. In this article, we will compare two prominent data observability tools: Datafold and Anomalo. Understanding the importance of data observability and the key features offered by these tools is essential for making an informed decision about which one suits your needs best. Let's dive in and explore the capabilities and unique selling points of Datafold and Anomalo.
Understanding Data Observability
Data observability refers to the ability to gain insights into the health, trustworthiness, and overall quality of data. It involves systematically monitoring, measuring, and managing data quality throughout its lifecycle. This practice ensures that data pipelines, transformations, and analyses are reliable and accurate, ultimately improving the decision-making process.
With the increasing volume, variety, and velocity of data, traditional approaches to data management are no longer sufficient. Organizations require comprehensive data observability tools that can provide real-time visibility into the quality and reliability of their data assets.
The Importance of Data Observability
Data observability plays a vital role in ensuring data-driven decision-making and maintaining the integrity of data operations. By proactively monitoring data quality, organizations can identify and address issues before they impact critical business processes. This minimizes the risk of making incorrect decisions based on flawed or incomplete data.
Furthermore, data observability enables organizations to meet regulatory compliance requirements, ensure data privacy, and improve data governance practices. With robust observability tools, organizations can establish trust in their data assets and enhance collaboration among data teams.
Key Features of Data Observability Tools
Data observability tools offer a range of features to help organizations gain insights into their data assets. These features typically include:
- Data Profiling: Tools like Datafold and Anomalo provide comprehensive data profiling capabilities, allowing users to understand the structure, content, and quality of their data through statistical analysis and visualizations.
- Data Lineage: Understanding the origin and transformations applied to data is crucial for ensuring data integrity. Data observability tools offer data lineage capabilities, enabling users to trace data back to its source and track its transformations and dependencies.
- Data Quality Monitoring: Effective data observability requires continuous monitoring of data health. Tools like Datafold and Anomalo offer data quality monitoring features that detect anomalies, inconsistencies, and errors in real-time.
- Data Validation: Data observability tools facilitate data validation by allowing users to define and enforce data quality rules. These rules help identify and flag deviations from expected data patterns and ensure data accuracy.
These are just a few key features of data observability tools, and both Datafold and Anomalo offer additional functionalities to address the complex challenges associated with data quality management.
When it comes to data profiling, Datafold and Anomalo go beyond basic statistical analysis. They provide advanced visualizations that allow users to explore the distribution of data across different dimensions, identify outliers, and detect patterns that may not be immediately apparent. This level of granularity empowers data teams to gain a deep understanding of their data, enabling them to make informed decisions and take proactive measures to improve data quality.
In terms of data lineage, Datafold and Anomalo offer comprehensive tracking capabilities that go beyond simple source-to-destination mapping. They allow users to visualize the entire data flow, including intermediate transformations and dependencies. This detailed lineage information helps organizations identify potential bottlenecks, troubleshoot issues, and ensure the accuracy and reliability of their data.
An Introduction to Datafold
Datafold is a data observability platform designed to empower data teams with real-time insights into their data pipelines. With its intuitive interface and powerful features, Datafold enables organizations to improve data quality, accelerate development cycles, and enhance collaboration among data stakeholders.
At Datafold, we understand the critical role that data plays in driving business decisions and outcomes. That's why we have developed a comprehensive set of functionality to ensure data observability throughout the entire data lifecycle.
Overview of Datafold's Functionality
Datafold offers a wide range of functionality aimed at ensuring data observability:
- Metadata Management: Datafold allows users to manage and organize metadata, providing a comprehensive view of data assets, lineage, and validations. This ensures that data teams have a clear understanding of the data they are working with, reducing the risk of errors and improving overall data quality.
- Data Monitoring: The platform enables proactive data monitoring by detecting anomalies, data drift, and inconsistencies across various stages of the data pipeline. With real-time alerts and notifications, data teams can quickly identify and address any issues that may impact the accuracy and reliability of their data.
- Alerts and Notifications: Datafold provides customizable alerts and notifications, ensuring that potential data quality issues are promptly identified and addressed. This allows data teams to take immediate action to rectify any issues, minimizing the impact on downstream processes and analyses.
- Collaboration: Datafold fosters collaboration among data teams by providing a centralized platform for sharing insights, documenting issues, and tracking data quality improvements. With features like comments and annotations, data stakeholders can easily communicate and collaborate on data-related tasks, leading to more efficient and effective data operations.
By offering these robust functionalities, Datafold empowers data teams to gain full visibility into their data pipelines, enabling them to make informed decisions and drive business success.
Unique Selling Points of Datafold
Datafold offers several standout features that set it apart from other data observability tools:
- Automated Data Testing: Datafold automates the testing of data pipelines, enabling organizations to identify and fix data quality issues early in the development process. This not only saves time and effort but also ensures that data teams can deliver high-quality data consistently.
- Data Drift Detection: The platform helps detect changes and discrepancies in data over time, allowing users to understand the impact of these changes on downstream processes and analyses. By providing insights into data drift, Datafold empowers data teams to proactively address any issues and maintain data integrity.
- Data Comparison: Datafold provides an easy-to-use interface for comparing data sets, enabling users to identify differences and inconsistencies between different versions or sources of data. This feature is particularly valuable when working with complex data pipelines, as it allows data teams to pinpoint discrepancies and take corrective actions swiftly.
With these unique selling points, Datafold goes beyond traditional data observability tools, providing data teams with the tools they need to ensure data accuracy, reliability, and consistency.
An Introduction to Anomalo
Anomalo is another popular data observability tool that offers a comprehensive suite of features to improve data quality and ensure reliable data operations.
Overview of Anomalo's Functionality
Anomalo provides a range of functionality to support data observability:
- Data Quality Analysis: Anomalo offers powerful data profiling and analysis capabilities to identify data quality issues, anomalies, and outliers.
- Data Lineage Visualization: The platform provides a graphical representation of data lineage, allowing users to visualize data transformations and dependencies.
- Real-time Monitoring: Anomalo enables real-time monitoring of data quality metrics, ensuring that potential issues are identified promptly.
- Integrated Remediation: The platform integrates with existing data workflows and systems to facilitate the remediation of data quality issues.
Unique Selling Points of Anomalo
Anomalo distinguishes itself with the following features:
- Machine Learning Capabilities: The platform leverages machine learning algorithms to automatically detect data anomalies and improve the accuracy of data quality assessment.
- Root Cause Analysis: Anomalo provides advanced analytics capabilities to identify the root causes of data quality issues, helping users address the underlying problems effectively.
- Automation and Orchestration: Anomalo offers automated data validation and orchestration features, reducing manual effort and enabling efficient data quality management.
Comparing Datafold and Anomalo
Performance Comparison
When it comes to performance, both Datafold and Anomalo offer powerful capabilities for data observability. However, it's important to consider the unique requirements and constraints of your organization.
Datafold's automated data testing and data drift detection features make it well-suited for agile development environments, allowing data teams to identify and resolve issues quickly. On the other hand, Anomalo's machine learning capabilities provide advanced data anomaly detection, making it ideal for organizations dealing with high-volume and rapidly evolving data.
Ultimately, the performance of each tool depends on the specific use case and the individual needs of your organization.
User Interface and Ease of Use
Both Datafold and Anomalo prioritize user experience and offer intuitive interfaces. Datafold's clean and user-friendly interface makes it easy for data teams to navigate and access the necessary information. Anomalo also provides a visually appealing interface with interactive visualization capabilities that enhance data exploration and analysis.
While both tools strive to provide a seamless user experience, the choice between Datafold and Anomalo ultimately depends on the preferences and requirements of your organization.
Pricing Structure
It's important to consider the pricing structure when evaluating data observability tools. Datafold and Anomalo offer flexible pricing options tailored to the needs of different organizations.
Datafold offers a tiered pricing model based on the number of data sources and features required. Anomalo follows a similar approach, providing pricing plans based on the scope of usage and the desired level of functionality.
Organizations should carefully assess their specific requirements and budgetary constraints when comparing the pricing models of Datafold and Anomalo.
Pros and Cons of Datafold
Advantages of Using Datafold
Datafold offers numerous benefits:
- Improved Data Quality: Datafold's comprehensive monitoring and quality analysis features enable organizations to enhance the reliability and accuracy of their data.
- Efficient Data Testing: The platform's automated data testing capabilities significantly reduce manual effort, improving development efficiency and accelerating time to production.
- Collaboration and Transparency: Datafold's collaboration features foster transparency and collaboration among data teams, ensuring alignment and knowledge sharing.
Limitations of Datafold
While Datafold offers significant advantages, it's important to consider its limitations:
- Limited Ecosystem Integration: Datafold's integration options may be limited compared to other data observability tools. organizations with complex data ecosystems may need to evaluate compatibility before adoption.
- Learning Curve: Like any powerful tool, Datafold requires some learning to fully harness its capabilities. Organizations should allocate time for training and onboarding to ensure optimal usage.
In conclusion, both Datafold and Anomalo provide robust solutions for data observability, enabling organizations to ensure the quality and reliability of their data. By understanding the importance of data observability and evaluating the key features and unique selling points of these tools, organizations can make an informed decision based on their specific requirements and priorities. Whether you choose Datafold or Anomalo, investing in a data observability tool is a proactive step towards driving data-driven decision-making, improving data quality, and maximizing the value of your data assets.
As you consider the right data observability tool for your organization, remember that the journey doesn't end there. CastorDoc offers an integrated solution that combines advanced governance, cataloging, and lineage capabilities with a user-friendly AI assistant, elevating your data management to new heights. With CastorDoc, you can enable self-service analytics, ensuring that both your data teams and business users can leverage data effectively. Discover how CastorDoc can complement your data observability efforts and transform your data governance by checking out more tools comparisons here.
You might also like
Get in Touch to Learn More
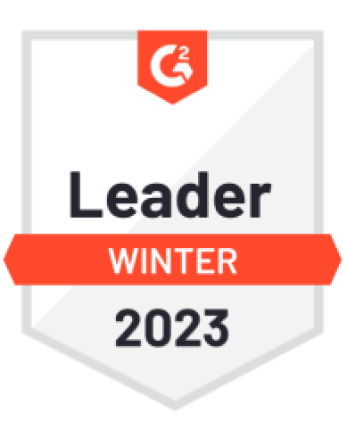
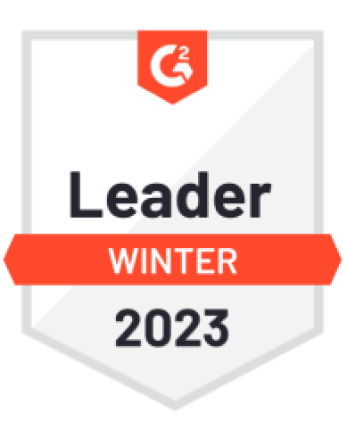

“[I like] The easy to use interface and the speed of finding the relevant assets that you're looking for in your database. I also really enjoy the score given to each table, [which] lets you prioritize the results of your queries by how often certain data is used.” - Michal P., Head of Data