Data Observability Tool Comparison: Monte Carlo vs. Marquez
In today's data-driven world, ensuring the quality and reliability of your data is crucial. This is where data observability comes into play. Data observability refers to the process of monitoring, understanding, and improving the quality of your data. In this article, we will compare two popular data observability tools: Monte Carlo and Marquez. By examining their features, functions, strengths, and weaknesses, we will help you make an informed decision about which tool is best suited for your data observability needs.
Understanding Data Observability
Data observability is all about gaining visibility into your data. It involves monitoring and analyzing your data pipelines to ensure that data is accurate, complete, and consistent. By implementing data observability practices, businesses can identify data quality issues, detect anomalies, and mitigate potential risks. This ultimately leads to better decision-making and improved business outcomes.
When diving deeper into the realm of data observability, it becomes evident that the process is not just about monitoring data but also about understanding the context in which the data operates. It involves tracking the flow of data from its source to its destination, ensuring that transformations and manipulations are accurately captured and documented. This level of insight is crucial for organizations looking to optimize their data processes and ensure data integrity.
The Importance of Data Observability
Data is the lifeblood of any organization, and making decisions based on inaccurate or inconsistent data can have serious consequences. Data observability helps ensure the reliability and trustworthiness of your data, enabling stakeholders to make informed decisions confidently. It also ensures compliance with regulations and establishes a foundation for data-driven initiatives such as machine learning and AI.
Furthermore, data observability plays a vital role in fostering a culture of data transparency within an organization. By promoting visibility and accountability around data quality and usage, businesses can foster collaboration among teams and drive innovation. This transparency also aids in building trust among stakeholders, both internal and external, enhancing the overall credibility of the organization.
Key Features of Data Observability Tools
Effective data observability tools offer a range of features to help businesses monitor and improve their data quality. These features typically include data lineage tracking, anomaly detection, data profiling, data cataloging, and data validation. By providing comprehensive visibility into your data ecosystem, these tools empower organizations to identify and resolve issues swiftly.
In addition to the core features, modern data observability tools are equipped with advanced capabilities such as predictive analytics and automated alerting. These functionalities enable proactive monitoring of data pipelines, allowing organizations to anticipate and address potential issues before they escalate. By leveraging these advanced features, businesses can enhance their data governance practices and ensure continuous data reliability.
Introduction to Monte Carlo
Monte Carlo is a leading data observability tool designed to provide end-to-end visibility into data pipelines. It helps organizations monitor data quality, detect anomalies, and ensure the accuracy and reliability of their data. With its user-friendly interface and powerful features, Monte Carlo simplifies the complex process of data observability.
One of the key strengths of Monte Carlo is its ability to scale with the growing needs of an organization. Whether you are a small startup or a large enterprise, Monte Carlo can adapt to your data monitoring requirements. This scalability ensures that as your data ecosystem expands, Monte Carlo can continue to provide comprehensive insights and monitoring capabilities.
Overview of Monte Carlo
Monte Carlo offers a holistic view of your data by tracking data lineage and providing a clear understanding of data transformations. It allows you to monitor data quality in real-time, helping you proactively identify and resolve issues. Monte Carlo integrates seamlessly with various data platforms, making it suitable for organizations with diverse data ecosystems.
Moreover, Monte Carlo's intuitive dashboard provides customizable visualizations that enable users to gain deep insights into their data health at a glance. By presenting complex data quality metrics in a user-friendly format, Monte Carlo empowers data teams to make informed decisions quickly and effectively.
Key Functions and Capabilities of Monte Carlo
Monte Carlo offers a range of functions and capabilities to enhance data observability. It provides automated data quality monitoring, enabling you to set up custom alerts and notifications for data anomalies. Monte Carlo also offers automated data catalogs and data profiling, simplifying the process of understanding your data and ensuring its accuracy.
Furthermore, Monte Carlo's machine learning algorithms continuously learn from your data patterns, allowing the tool to adapt and improve its anomaly detection capabilities over time. This dynamic approach to data monitoring ensures that Monte Carlo remains at the forefront of detecting and addressing data quality issues, providing organizations with a reliable solution for maintaining data integrity.
Introduction to Marquez
Marquez is another popular data observability tool that helps organizations gain visibility into their data pipelines. Designed for the modern data ecosystem, Marquez offers a scalable and reliable solution for monitoring and improving data quality. With its robust feature set, Marquez empowers businesses to confidently make data-driven decisions.
Many organizations rely on Marquez to streamline their data operations and ensure data integrity throughout their pipelines. By providing a clear and detailed view of data lineage, Marquez enables teams to quickly troubleshoot issues and optimize their processes for maximum efficiency. With Marquez, businesses can establish a solid foundation for their data infrastructure, fostering a culture of trust and transparency.
Overview of Marquez
Marquez provides a comprehensive data lineage tracking system that allows organizations to understand the origins of their data and track its transformations. By offering a centralized view of your data, Marquez helps identify data quality issues and ensures data reliability. Marquez integrates seamlessly with popular data platforms such as Apache Spark and Apache Hive.
Moreover, Marquez goes beyond traditional data lineage tools by offering advanced metadata management capabilities. Users can annotate their data assets with custom tags and descriptions, facilitating better organization and searchability. This metadata enrichment feature enhances collaboration among team members and promotes a deeper understanding of the data landscape.
Key Functions and Capabilities of Marquez
Marquez offers a variety of functions and capabilities to enhance data observability. It provides automated data lineage tracking, enabling you to trace the journey of your data from source to destination. Marquez also offers data discovery and data cataloging features, making it easy to understand and manage your data assets.
Additionally, Marquez includes built-in data quality monitoring tools that help organizations proactively identify anomalies and discrepancies in their datasets. By setting up alerts and notifications, users can stay informed about any issues that may arise, allowing for swift remediation actions. This proactive approach to data quality assurance sets Marquez apart as a comprehensive solution for data observability and governance.
Detailed Comparison: Monte Carlo vs. Marquez
User Interface and Usability
When comparing Monte Carlo and Marquez, user interface and usability are important factors to consider. Monte Carlo boasts an intuitive and user-friendly interface, allowing users to easily navigate and monitor their data pipelines. The interface is designed with a clean and organized layout, making it effortless for users to locate and access the necessary features.
Marquez, on the other hand, offers a sleek and modern interface that provides a seamless user experience. The interface is visually appealing, with a minimalist design that focuses on simplicity and ease of use. Users will find it effortless to navigate through the various functionalities and perform tasks efficiently.
Both tools prioritize user experience and have invested in creating interfaces that are not only visually appealing but also highly functional, ensuring that users can effortlessly interact with their data pipelines.
Data Processing and Management
Both Monte Carlo and Marquez excel in data processing and management capabilities. Monte Carlo offers automated data quality monitoring, alerting users to any anomalies or issues in real-time. This feature allows organizations to proactively identify and address data quality issues, ensuring the accuracy and reliability of their data.
Marquez, on the other hand, provides a comprehensive data lineage tracking system, allowing organizations to understand the journey of their data and identify any quality issues. With Marquez, users can easily trace the origin and transformation of their data, enabling them to make informed decisions and maintain data integrity.
Both tools prioritize data quality and provide robust mechanisms to ensure that organizations can effectively process and manage their data, guaranteeing its accuracy and reliability throughout the data pipeline.
Integration and Compatibility
When it comes to integration and compatibility, both tools provide seamless integration with popular data platforms. Monte Carlo integrates well with platforms such as Snowflake, Google BigQuery, and Amazon Redshift. This compatibility allows users to leverage their existing data infrastructure and seamlessly incorporate Monte Carlo into their workflows.
Marquez, on the other hand, integrates with Apache Spark, Apache Hive, and other popular tools in the data ecosystem. This broad compatibility ensures that organizations can easily integrate Marquez into their existing data workflows, without the need for significant changes or disruptions.
Both tools prioritize compatibility and have invested in creating integrations that enable users to seamlessly incorporate them into their data ecosystems, reducing the complexity and effort required for adoption.
Pricing and Value for Money
Monte Carlo and Marquez offer different pricing models, depending on your organization's specific needs. It is worth considering the features and benefits each tool provides to determine their value for money.
Monte Carlo offers a flexible pricing structure based on data volume. This model allows organizations to scale their usage and pay only for the data they process, ensuring cost-effectiveness and optimal resource allocation.
Marquez, on the other hand, follows a subscription-based model that provides unlimited data volume and users. This pricing model offers predictability and simplicity, allowing organizations to have full access to Marquez's capabilities without worrying about additional costs based on data volume.
Both tools provide value for money by offering pricing models that cater to different organizational needs, ensuring that organizations can choose the option that aligns with their budget and usage requirements.
Strengths and Weaknesses
Monte Carlo's Strengths and Weaknesses
Monte Carlo's strengths lie in its user-friendly interface, real-time data quality monitoring, and seamless integration with various data platforms. However, some users have reported that Monte Carlo lacks advanced analytics capabilities and requires additional configuration for complex data pipelines.
Marquez's Strengths and Weaknesses
Marquez's strengths lie in its comprehensive data lineage tracking, centralized data view, and compatibility with popular data platforms. However, some users have found the initial setup process to be complex, and additional customization may be required for specific use cases.
In conclusion, both Monte Carlo and Marquez are powerful data observability tools that can help organizations monitor, understand, and improve the quality of their data. While Monte Carlo offers a user-friendly interface and real-time monitoring capabilities, Marquez provides comprehensive data lineage tracking and compatibility with popular data platforms. Your choice between the two will ultimately depend on your specific requirements and preferences. With the information provided in this article, you can now make an informed decision and select the data observability tool that best suits your needs.
As you consider the right data observability tool for your organization, remember that the landscape is rich with innovative solutions like CastorDoc. CastorDoc distinguishes itself by integrating advanced governance, cataloging, and lineage capabilities with a user-friendly AI assistant, enabling self-service analytics that cater to both data professionals and business users. With its robust data catalog and conversational interface, CastorDoc offers a unique blend of control, compliance, and accessibility, revolutionizing data management and utilization. To explore how CastorDoc compares to other tools in the modern data stack and to understand how it can elevate your data governance and observability strategy, check out more tools comparisons here.
You might also like
Get in Touch to Learn More
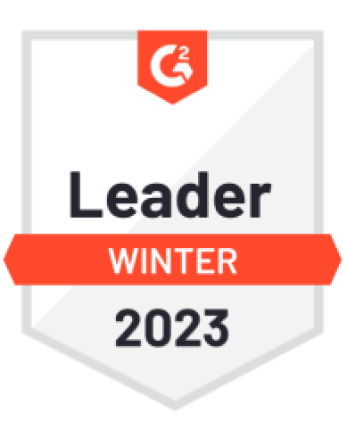
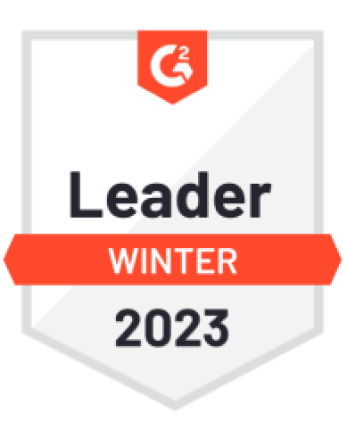

“[I like] The easy to use interface and the speed of finding the relevant assets that you're looking for in your database. I also really enjoy the score given to each table, [which] lets you prioritize the results of your queries by how often certain data is used.” - Michal P., Head of Data