Data Observability Tool Comparison: Monte Carlo vs. Metaplane
In today's data-driven world, organizations rely heavily on the accuracy and reliability of their data. Ensuring data observability has become a critical aspect of maintaining trust in data pipelines and making informed decisions. Two popular tools in this space are Monte Carlo and Metaplane. In this article, we will compare these two data observability tools to help you understand their key features, pros and cons, and make an informed choice for your organization.
Understanding Data Observability
Data observability refers to the ability to understand, measure, and monitor the quality and reliability of data in real-time. It involves continuous monitoring, reporting, and alerting on data issues to ensure data integrity. By ensuring data observability, organizations can identify and address inconsistencies, inaccuracies, and anomalies in their datasets, leading to improved data quality and trustworthiness.
But what does it mean to have reliable and accurate data? Let's dive deeper into the importance of data observability and how it impacts organizations.
The Importance of Data Observability
Reliable and accurate data is the foundation for making data-driven decisions. Without proper data observability mechanisms in place, organizations risk making flawed or biased decisions based on faulty or incomplete data. Data observability enables organizations to proactively detect and address data issues, preventing costly mistakes and increasing operational efficiency.
Imagine a scenario where a retail company relies on data to forecast customer demand and optimize inventory management. Without data observability, the company might unknowingly base their decisions on incomplete or inaccurate data, leading to overstocking or stockouts. This can result in financial losses, customer dissatisfaction, and missed business opportunities.
On the other hand, with robust data observability practices, the retail company can continuously monitor their data pipelines and systems, ensuring that the data used for decision-making is accurate and reliable. They can set up automated data quality checks to identify inconsistencies and inaccuracies, allowing them to take corrective actions promptly. By having real-time alerts and notifications for data issues, they can address problems before they impact operations, ensuring smooth and efficient business processes.
Key Features of Data Observability Tools
Data observability tools offer a wide range of features to help organizations ensure data accuracy and reliability. Let's explore some of the key features:
- Data Monitoring: Real-time monitoring of data pipelines and systems to detect anomalies and errors. This feature allows organizations to have a pulse on their data, ensuring that any issues are promptly identified and resolved.
- Data Quality Checks: Automated validation and verification of data to identify inconsistencies and inaccuracies. These checks help organizations maintain data integrity and ensure that the data they rely on is trustworthy.
- Alerting and Notifications: Instantaneous alerts and notifications for data issues, ensuring timely resolution. With this feature, organizations can stay proactive and address data issues before they escalate.
- Data Lineage and Metadata: Tracking and documenting the origin, transformation, and usage of data for improved traceability and transparency. This feature allows organizations to understand the journey of their data, making it easier to troubleshoot issues and ensure compliance.
- Root Cause Analysis: In-depth analysis of data issues to identify the root cause and prevent recurrence. By conducting thorough investigations, organizations can address the underlying problems, preventing similar issues from happening in the future.
These features empower organizations to have a comprehensive view of their data, ensuring its accuracy, reliability, and trustworthiness. With data observability tools in place, organizations can confidently leverage their data to make informed decisions, drive innovation, and achieve their business goals.
Introduction to Monte Carlo
Monte Carlo is a data observability tool designed to ensure the reliability and quality of data. It leverages advanced machine learning algorithms to detect and prevent data issues in real-time. Let's explore the overview, key features, and pros and cons of Monte Carlo.
Overview of Monte Carlo
Monte Carlo provides end-to-end data observability, enabling organizations to identify and address data issues proactively. With its intuitive interface, Monte Carlo allows users to monitor data pipelines, validate data quality, and receive real-time alerts for anomalies or inconsistencies.
But what exactly does data observability mean? In simple terms, it refers to the ability to gain visibility into the health and reliability of data. Just like observability is crucial in other domains, such as software development or infrastructure management, it is equally important in the world of data. Without proper observability, data issues can go unnoticed, leading to incorrect insights and decisions.
Monte Carlo takes data observability to the next level by automating the monitoring process. It continuously tracks data pipelines, ensuring that the data meets defined quality standards. This proactive approach helps organizations detect and address issues before they impact critical business processes.
Key Features of Monte Carlo
Some key features of Monte Carlo include:
- Automated Data Monitoring: Monte Carlo continuously monitors data pipelines, ensuring that data meets defined quality standards. It saves time and effort by eliminating the need for manual checks.
- Quality Metrics Tracking: Monte Carlo tracks key data quality metrics, providing insights into the reliability and integrity of the data. This allows organizations to measure and improve data quality over time.
- Anomaly Detection: Advanced machine learning algorithms detect anomalies and deviations from expected data patterns. This helps in identifying and resolving data issues before they cause significant problems.
- Collaboration and Workflow Integration: Monte Carlo seamlessly integrates with existing workflows and collaboration tools, making it easy to share insights and resolve data issues. It promotes cross-team collaboration and ensures a smooth data observability process.
These features collectively empower organizations to establish a robust data observability practice, ensuring the accuracy and trustworthiness of their data.
Pros and Cons of Monte Carlo
Like any tool, Monte Carlo has its pros and cons. Some advantages of using Monte Carlo include:
- Real-Time Monitoring: Monte Carlo provides real-time monitoring, ensuring timely detection and resolution of data issues. This helps organizations maintain data integrity and make informed decisions based on up-to-date information.
- Automated Anomaly Detection: With its advanced machine learning algorithms, Monte Carlo can automatically detect anomalies, reducing the manual effort required. This frees up valuable time for data teams to focus on more strategic tasks.
- User-Friendly Interface: Monte Carlo offers an intuitive interface, making it easy for users to navigate and interpret data observability insights. Its user-friendly design ensures that users can quickly understand and act upon the information presented.
On the flip side, some limitations of Monte Carlo include:
- Single Tool Dependency: Monte Carlo provides comprehensive data observability, but organizations relying on other observability tools may face challenges in integrating them. This can lead to a fragmented observability landscape and increased complexity in managing data quality.
- Limited Data Transformation Capabilities: Monte Carlo primarily focuses on data quality monitoring and may lack advanced data transformation capabilities. Organizations requiring extensive data transformation functionalities may need to supplement Monte Carlo with additional tools.
Despite these limitations, Monte Carlo remains a powerful solution for organizations seeking to ensure the reliability and quality of their data. Its advanced features and automation capabilities make it a valuable asset in the data observability toolbox.
Introduction to Metaplane
Metaplane is another popular data observability tool that helps organizations ensure the reliability and accuracy of their data. Let's explore an overview of Metaplane, its key features, as well as its pros and cons.
Overview of Metaplane
Metaplane provides a comprehensive data observability platform, enabling organizations to monitor, validate, and manage data quality across their entire data ecosystem. It offers intuitive dashboards, automated alerts, and collaborative workflows to ensure that data issues are promptly identified and resolved.
Key Features of Metaplane
Metaplane offers a wide range of features to support data observability, including:
- Data Quality Monitoring: Metaplane monitors data quality in real-time, performing continuous checks to ensure data accuracy and consistency.
- Data Profiling and Analysis: Metaplane performs comprehensive data profiling and analysis, providing insights into data quality, completeness, and transformation.
- Integrated Data Catalog: Metaplane maintains a centralized catalog of data assets, facilitating easy discovery, understanding, and governance of data.
- Collaboration and Workflow Automation: Metaplane enables collaboration between data teams, automating workflows and notifications to streamline data issue resolution.
Pros and Cons of Metaplane
Metaplane offers several benefits, such as:
- Comprehensive Data Observability: Metaplane provides end-to-end data observability, covering data quality, lineage, and governance.
- Powerful Data Profiling and Analysis: With its extensive data profiling capabilities, Metaplane enables organizations to gain deep insights into their datasets.
- Simplified Data Governance: Metaplane's integrated data catalog facilitates data discovery, understanding, and governance, ensuring compliance and accountability.
However, Metaplane also has some limitations, including:
- Steep Learning Curve: Due to its comprehensive feature set, getting started with Metaplane may require a steep learning curve for users.
- Higher Cost: The extensive functionality of Metaplane may come at a higher price point, making it less accessible for smaller organizations with limited budgets.
In-depth Comparison: Monte Carlo vs. Metaplane
Now that we have explored the key features, pros, and cons of both tools, let's dive into an in-depth comparison between Monte Carlo and Metaplane.
Comparison of Key Features
When it comes to data observability, both Monte Carlo and Metaplane offer robust features for monitoring, validating, and managing data quality. Here's a comparison of their key features:
- Data Monitoring: Both tools provide real-time data monitoring, alerting users of any anomalies or inconsistencies.
- Data Quality Checks: Monte Carlo and Metaplane automate data quality checks, ensuring data accuracy and reliability.
- Data Lineage and Metadata: Metaplane excels in metadata and data lineage management, while Monte Carlo focuses more on data quality monitoring.
- Collaboration and Workflow Integration: Both tools offer collaboration features, but Metaplane provides more extensive workflow automation capabilities.
Performance Analysis
In terms of performance, both Monte Carlo and Metaplane are powerful tools that can significantly enhance data observability. The ideal choice depends on the specific needs and requirements of your organization. Consider factors such as data complexity, integration capabilities, and budget constraints when making a decision.
Pricing Comparison
Pricing is a crucial factor when evaluating data observability tools. Both Monte Carlo and Metaplane offer flexible pricing models, including subscription-based plans and enterprise agreements. It is advisable to contact their sales teams for detailed pricing information tailored to your organization's needs.
In conclusion, both Monte Carlo and Metaplane are robust data observability tools that can help organizations ensure data accuracy and reliability. While Monte Carlo excels in real-time data monitoring and anomaly detection, Metaplane offers comprehensive data profiling, analysis, and governance capabilities. Make an informed decision by considering your organization's specific requirements and budget constraints. Remember, data observability is not a luxury but a necessity for organizations that value accurate and reliable data.
As you consider the benefits of data observability tools like Monte Carlo and Metaplane, don't overlook the transformative potential of CastorDoc. With its advanced governance, cataloging, and lineage capabilities, coupled with a user-friendly AI assistant, CastorDoc is an exceptional tool that enables self-service analytics and empowers your organization to harness the full power of your data. Whether you're looking to streamline data governance, enhance compliance, or facilitate seamless data discovery and utilization, CastorDoc offers a comprehensive solution that meets the needs of both data teams and business users alike. To explore how CastorDoc compares to other tools and to discover how it can elevate your data observability strategy, check out more tools comparisons here.
You might also like
Get in Touch to Learn More
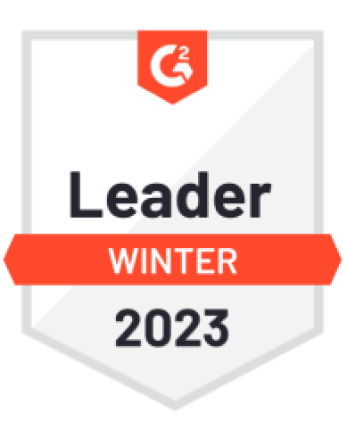
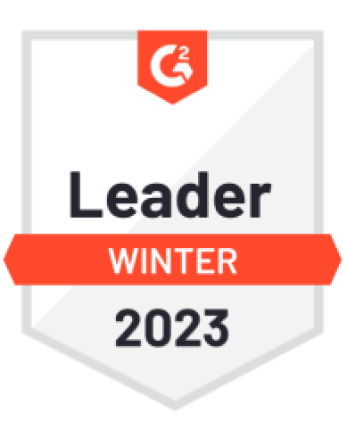

“[I like] The easy to use interface and the speed of finding the relevant assets that you're looking for in your database. I also really enjoy the score given to each table, [which] lets you prioritize the results of your queries by how often certain data is used.” - Michal P., Head of Data