Data Observability Tool Comparison: Monte Carlo vs. Validio
In today's data-driven world, organizations rely heavily on the accuracy and reliability of their data. However, data quality issues can often go unnoticed, leading to erroneous insights and decisions. This is where data observability tools come into play. In this article, we will compare two popular data observability tools: Monte Carlo and Validio. By understanding their features, pros and cons, and comparing them head-to-head, you'll be able to make an informed decision on which tool is best suited for your organization's needs.
Understanding Data Observability
Data observability is the practice of ensuring the quality and integrity of data throughout its lifecycle. It involves monitoring, validating, and verifying data to identify anomalies, inconsistencies, and errors. By implementing data observability, organizations can gain confidence in the accuracy and reliability of their data, enabling them to make data-driven decisions with greater certainty.
The Importance of Data Observability
Data observability is crucial for maintaining data integrity and ensuring the success of data-driven initiatives. Without proper observability, organizations risk making decisions based on flawed or incomplete data, which can have severe consequences. By investing in data observability tools, organizations can proactively detect and address data issues, reducing the likelihood of costly errors and improving overall operational efficiency.
Key Features of Data Observability Tools
Data observability tools offer a range of features to help organizations ensure data quality. Some key features to consider when evaluating these tools include:
- Data Monitoring: The ability to continuously monitor data pipelines, databases, and data lakes to identify anomalies and deviations from expected behavior.
- Data Validation: The ability to validate data against predefined rules and logic, ensuring its accuracy and consistency.
- Data Profiling: The ability to analyze data to discover patterns, relationships, and potential issues.
- Error Detection and Alerting: The ability to detect errors and anomalies in real-time and send alerts and notifications to relevant stakeholders.
- Data Lineage and Impact Analysis: The ability to track the origin and transformations of data, enabling organizations to understand the impact of changes on downstream processes.
One of the key benefits of data observability tools is their ability to provide organizations with a holistic view of their data ecosystem. These tools can integrate with various data sources and systems, allowing organizations to monitor and validate data across different platforms and technologies. This comprehensive view enables organizations to identify and address data issues that may arise from data integration, transformation, or migration processes.
In addition to monitoring and validating data, data observability tools also offer advanced analytics capabilities. These capabilities allow organizations to gain deeper insights into their data, uncover hidden patterns, and identify potential areas for improvement. By leveraging these analytics features, organizations can optimize their data processes, improve data quality, and enhance overall data-driven decision-making.
Introduction to Monte Carlo
Monte Carlo is a powerful data observability tool that aims to identify and prevent data quality issues before they impact critical business processes. It offers a wide range of features and capabilities to help organizations ensure the accuracy and reliability of their data.
Overview of Monte Carlo
Monte Carlo operates based on the principle of data monitoring through statistical analysis. It analyzes data patterns and behaviors, allowing organizations to detect and address data quality issues in real-time. By using advanced algorithms and machine learning techniques, Monte Carlo is able to identify anomalies and deviations, ensuring data integrity.
Key Features of Monte Carlo
Monte Carlo offers several key features that make it a robust data observability tool:
- Data Monitoring: Continuous monitoring of data pipelines and systems, ensuring real-time visibility into data quality.
- Anomaly Detection: Utilizing statistical analysis and machine learning algorithms to identify deviations and anomalies in data.
- Alerts and Notifications: Sending real-time alerts and notifications when data quality issues are detected, enabling immediate action.
- Data Lineage: Tracking the origin and transformations of data for comprehensive data lineage analysis.
- Collaboration and Workflow Management: Facilitating collaboration among data teams and streamlining workflows for efficient data quality management.
Pros and Cons of Using Monte Carlo
Like any tool, Monte Carlo has its strengths and limitations. Here are some pros and cons to consider:
Pros:
- Powerful Anomaly Detection: Monte Carlo's advanced algorithms and machine learning capabilities enable it to detect even subtle data anomalies.
- Real-time Monitoring: Continuous monitoring allows organizations to proactively address data quality issues before they impact critical processes.
- Comprehensive Data Lineage: Monte Carlo provides detailed data lineage information, enabling organizations to understand how data flows through their systems.
Cons:
- Complex Configuration: Setting up and configuring Monte Carlo can be time-consuming and require technical expertise.
- Limited Integration Options: Monte Carlo may have limitations when it comes to integrating with certain data sources and platforms.
- Cost: Depending on the scale of your organization's data infrastructure, the cost of using Monte Carlo can be a consideration.
Introduction to Validio
Validio is another popular data observability tool that provides organizations with the means to ensure data quality and integrity. With a range of features and capabilities, Validio aims to simplify the process of data validation and monitoring.
Overview of Validio
Validio focuses on data validation and quality monitoring, offering a user-friendly interface and intuitive workflows. It allows organizations to define validation rules and logic and easily apply them to their data pipelines, ensuring data integrity and consistency.
Key Features of Validio
Validio offers several key features that make it a valuable data observability tool:
- Data Validation Rules: The ability to define custom validation rules and logic, ensuring data accuracy and consistency.
- Automated Data Monitoring: Continuous monitoring of data pipelines, databases, and data lakes for anomalies and inconsistencies.
- Error Tracking and Resolution: Streamlining the process of tracking and resolving data quality issues through automated workflows.
- Integration Capabilities: Seamless integration with a variety of data sources and platforms, allowing organizations to monitor data from different systems.
- Scalability: Validio is designed to handle large volumes of data, making it suitable for organizations with complex data infrastructures.
Pros and Cons of Using Validio
Here are some pros and cons of using Validio:
Pros:
- User-Friendly Interface: Validio's intuitive interface makes it easy for non-technical users to define validation rules and monitor data quality.
- Flexible Integration: Validio offers seamless integration with a wide range of data sources and platforms, providing organizations with greater flexibility.
- Automated Error Resolution: Validio's automated workflows help streamline the process of tracking and resolving data quality issues.
Cons:
- Less Advanced Anomaly Detection: Validio's anomaly detection capabilities may not be as sophisticated as those offered by other data observability tools.
- Limited Data Lineage Tracking: Validio may provide limited visibility into the lineage and transformations of data within complex data ecosystems.
- Cost: Depending on the organization's data infrastructure and scale, the cost of using Validio may be a consideration.
Detailed Comparison Between Monte Carlo and Validio
Now that we have explored the features, pros, and cons of both Monte Carlo and Validio, let's delve into a detailed comparison between the two tools.
Comparing User Interface and Ease of Use
Both Monte Carlo and Validio offer user-friendly interfaces that prioritize ease of use. Monte Carlo's interface is sleek and modern, providing intuitive workflows for defining rules and monitoring data quality. Validio, on the other hand, focuses on simplicity, allowing non-technical users to easily specify data validation rules. Ultimately, the choice between the two tools will depend on your organization's specific needs and the technical expertise of your data team.
Comparing Data Processing Capabilities
When it comes to data processing capabilities, both Monte Carlo and Validio offer robust features. Monte Carlo leverages advanced statistical analysis and machine learning techniques to detect anomalies in data patterns, providing organizations with comprehensive insights into their data quality. Validio, on the other hand, excels at data validation, allowing organizations to define and enforce custom validation rules. Ultimately, the choice between the two tools will depend on the nature of your organization's data and the specific requirements of your data observability initiatives.
Comparing Alert and Notification Systems
Alert and notification systems are crucial for timely response to data quality issues. Monte Carlo offers real-time alerts and notifications when anomalies are detected, ensuring organizations can take immediate action. Validio also provides alerting capabilities, but the extent of customization and flexibility may be limited compared to Monte Carlo. Consider the needs of your organization and the importance of real-time notifications in your data observability workflows when making your decision.
Comparing Pricing Structures
Pricing is an important consideration when evaluating data observability tools. Both Monte Carlo and Validio offer subscription-based pricing models, but the specific costs will depend on the scale and complexity of your organization's data infrastructure. It is crucial to consider the long-term costs, including any additional charges for integration or customizations. Evaluating the pricing structures against the features and capabilities offered by each tool will help in making the right choice for your organization.
Conclusion
Choosing the right data observability tool is essential for ensuring the accuracy and reliability of your data. Monte Carlo and Validio are both powerful options that offer unique features and capabilities. By thoroughly understanding their features, pros and cons, and comparing them head-to-head, you can make an informed decision that aligns with your organization's needs. Whether you prioritize advanced anomaly detection or user-friendly interfaces, assessing your requirements and evaluating these tools will set you on the path towards improved data observability and data-driven decision-making.
While Monte Carlo and Validio offer compelling features for data observability, the quest for comprehensive data management doesn't stop there. CastorDoc takes the concept further by integrating advanced governance, cataloging, and lineage capabilities with a user-friendly AI assistant, enabling self-service analytics that cater to both data teams and business users. If you're looking to elevate your organization's data governance and empower informed decision-making with an intuitive platform, CastorDoc might be the solution you need. Check out more tools comparisons here and discover how CastorDoc can revolutionize your data strategy.
You might also like
Get in Touch to Learn More
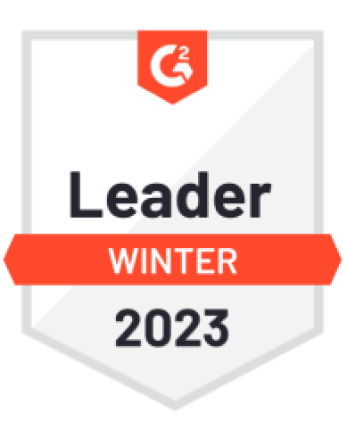
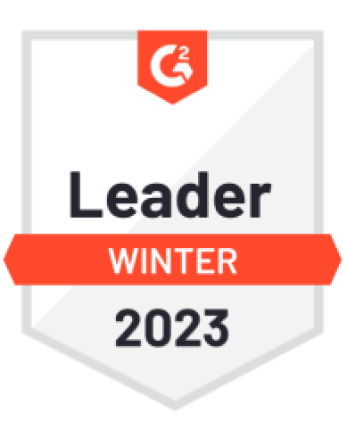

“[I like] The easy to use interface and the speed of finding the relevant assets that you're looking for in your database. I also really enjoy the score given to each table, [which] lets you prioritize the results of your queries by how often certain data is used.” - Michal P., Head of Data