Data Observability Tool Comparison: Soda vs. Lantern
In today's data-driven world, businesses rely heavily on data observability to ensure the integrity and reliability of their data operations. With the growing complexity of data landscapes, organizations need robust tools that can proactively monitor and detect anomalies, ensuring the quality and accuracy of their data. In this article, we will compare two leading data observability tools - Soda and Lantern - in terms of their functionality, unique selling points, performance, and user experience. By understanding the key differences and strengths of these tools, businesses can make an informed decision about which solution best suits their data observability needs.
Understanding Data Observability
Data observability refers to the ability to measure, monitor, and improve the quality and reliability of data in real-time. It involves a comprehensive set of practices, tools, and methodologies designed to detect, diagnose, and resolve issues related to data quality, integrity, and consistency. By implementing effective data observability strategies, organizations can enhance their decision-making processes, reduce risks, and accelerate their data-driven initiatives.
The Importance of Data Observability
Data lies at the core of every business operation, and any inaccuracies or inconsistencies can have far-reaching consequences. Data observability allows organizations to proactively monitor their data pipelines, identify potential issues, and take corrective actions before they impact critical business processes. It ensures that data remains accurate, reliable, and consistent, enabling businesses to make informed decisions, build trust with customers, and comply with regulatory requirements.
Key Features of Data Observability Tools
A robust data observability tool should offer a wide range of features to effectively monitor, analyze, and manage data quality. Some key features to look for in a data observability tool include:
- Data Profiling: The ability to analyze and understand the structure, content, and quality of data.
- Data Monitoring: Real-time monitoring of data pipelines and processes to detect anomalies and deviations.
- Data Quality Checks: The capability to perform automated data quality checks to ensure accuracy, completeness, and consistency.
- Data Lineage: Tracking and documenting the origin, transformations, and movement of data across the entire data pipeline.
- Error Detection and Alerting: Proactive detection of errors, anomalies, or data drift and timely alerts for remediation.
Data observability tools go beyond just monitoring and alerting. They provide organizations with deep insights into their data ecosystem, helping them understand the root causes of data issues and take proactive measures to prevent future problems. These tools often include advanced analytics capabilities, allowing users to visualize data flows, identify patterns, and uncover hidden relationships between different data sources.
Furthermore, data observability tools enable organizations to establish data governance frameworks, ensuring compliance with industry regulations and data privacy standards. They provide comprehensive auditing and reporting capabilities, allowing businesses to demonstrate data lineage, prove data integrity, and meet regulatory requirements.
Another important aspect of data observability is its impact on collaboration and cross-functional alignment within an organization. By providing a centralized platform for data monitoring and analysis, these tools facilitate collaboration between data engineers, data scientists, and business stakeholders. This collaboration fosters a culture of data-driven decision-making and empowers teams to work together towards common data quality goals.
Introduction to Soda
Soda is a leading data observability tool that provides organizations with the necessary capabilities to ensure data quality, integrity, and reliability. Let's take a closer look at the functionality that Soda offers.
Overview of Soda's Functionality
Soda offers a comprehensive suite of features to monitor and validate data quality. It provides data profiling capabilities that enable organizations to understand the structure and content of their data. By analyzing data schemas, statistics, and patterns, Soda helps detect anomalies, inconsistencies, and data drift.
In addition, Soda allows real-time monitoring of data pipelines, ensuring that data flows smoothly without any unexpected issues. Its automated data quality checks enable organizations to define and enforce data quality rules, ensuring that data meets predefined standards. With data lineage tracking, Soda provides end-to-end visibility into data movement, allowing organizations to trace data back to its source and identify any transformations or modifications along the way.
Unique Selling Points of Soda
Soda stands out from other data observability tools with its user-friendly interface and intuitive features. Its drag-and-drop functionality allows users to easily define and customize data quality checks, reducing the need for complex coding or scripting. Soda also provides interactive dashboards and visualizations, making it easier for users to understand and interpret data quality reports.
Furthermore, Soda's AI-powered anomaly detection capabilities enable organizations to proactively identify data quality issues and take prompt actions to rectify them. Its flexible deployment options, including cloud-based and on-premises installations, cater to the diverse needs of organizations across various industries.
Introduction to Lantern
Lantern is another popular data observability tool that offers a wide range of features to monitor, validate, and optimize data quality. Let's explore the functionality that Lantern brings to the table.
Overview of Lantern's Functionality
Lantern provides organizations with the ability to monitor and validate data quality across their entire data ecosystem. Its data profiling capabilities allow users to gain insights into data structure, metadata, and statistics. By leveraging statistical analysis, Lantern helps detect anomalies, errors, and outliers in the data.
With real-time data monitoring, Lantern ensures that data pipelines are operating smoothly and errors are identified and resolved in a timely manner. It supports customizable data quality checks, empowering users to define rules and policies aligned with their specific data quality requirements. Additionally, Lantern offers data lineage tracking, enabling users to understand the flow of data and identify potential issues or bottlenecks.
Unique Selling Points of Lantern
Lantern differentiates itself from other data observability tools through its seamless integration with popular data platforms and frameworks. It provides out-of-the-box connectors for various data sources, allowing organizations to easily integrate and monitor their data pipelines. This streamlined integration reduces implementation time and effort, enabling organizations to quickly gain insights into their data quality.
Lantern's advanced anomaly detection algorithms use machine learning techniques to identify patterns and anomalies in data, providing users with actionable insights. It also offers collaboration features, allowing teams to work together on data quality issues and resolutions. With its scalable architecture, Lantern can handle large volumes of data and support the data observability needs of enterprise-level organizations.
Comparing Tool Performance
Now that we have explored the functionality and unique selling points of Soda and Lantern, let's compare their performance in terms of speed, efficiency, and accuracy.
Speed and Efficiency Comparison
Both Soda and Lantern are designed to provide real-time monitoring and analysis of data pipelines. However, Soda's drag-and-drop interface and intuitive features make it easier for non-technical users to define and manage data quality checks. On the other hand, Lantern's seamless integration with popular data platforms allows for smoother data ingestion and reduces implementation efforts.
In terms of speed, both tools offer fast and responsive performance, ensuring that data is monitored and analyzed in near real-time. Soda's AI-powered anomaly detection capabilities can help organizations quickly identify data quality issues, while Lantern's machine learning algorithms provide valuable insights into data patterns and anomalies.
Accuracy and Reliability Comparison
When it comes to data accuracy and reliability, both Soda and Lantern excel in their respective ways. Soda's comprehensive data profiling capabilities enable organizations to identify data anomalies, inconsistencies, and inaccuracies. Its automated data quality checks help enforce data quality standards, ensuring that data meets defined criteria.
Lantern, on the other hand, leverages statistical analysis and machine learning techniques to detect anomalies and outliers in the data. Its customizable data quality checks allow users to define rules and policies specific to their data quality requirements. With both tools, organizations can rest assured that their data is accurate, reliable, and aligned with their quality standards.
User Experience Analysis
Now let's evaluate the user experience offered by Soda and Lantern in terms of ease of use and customer support.
Ease of Use: Soda vs. Lantern
Soda's user-friendly interface and drag-and-drop functionality make it easy for users to define and customize data quality checks. Its intuitive features require minimal coding or scripting, enabling non-technical users to effectively monitor and manage data quality. Soda's interactive dashboards and visualizations provide clear and actionable insights, making it easier for users to interpret and address data quality issues.
Lantern's streamlined integration with popular data platforms enhances its ease of use. By offering out-of-the-box connectors, Lantern simplifies the process of ingesting and monitoring data. Its intuitive interface and customizable data quality checks allow users to define and manage their data observability workflows effortlessly. Lantern also provides helpful documentation and resources to support users in leveraging the tool effectively.
Customer Support: Soda vs. Lantern
Both Soda and Lantern prioritize customer support to ensure a seamless user experience. Soda offers comprehensive documentation, tutorials, and knowledge bases to help users get started and troubleshoot issues. It also provides responsive customer support channels, including email and community forums, where users can seek assistance from experts and connect with other users.
Lantern provides similar customer support resources, including documentation, guides, and FAQs. Additionally, Lantern offers personalized support through email, ticketing systems, and dedicated customer success managers. These support channels enable users to get timely assistance and overcome any challenges they may encounter while using the tool.
Overall, both Soda and Lantern take the user experience seriously and strive to provide comprehensive support to their users.
Conclusion
In the realm of data observability, both Soda and Lantern offer robust capabilities to monitor, validate, and optimize data quality. While Soda's user-friendly interface and AI-powered anomaly detection provide a seamless user experience, Lantern's streamlined integration with popular data platforms and machine learning-based insights make it a strong contender. Each tool has its unique selling points, and organizations should carefully assess their specific requirements to make an informed decision.
By comparing the functionality, performance, and user experience of Soda and Lantern, businesses can choose a data observability tool that aligns with their needs, ensuring the reliability and accuracy of their data operations. Ultimately, investing in a data observability tool is a strategic step towards harnessing the full potential of data and enabling data-driven decision-making in today's fast-paced and data-centric world.
While Soda and Lantern provide valuable insights for data observability, the journey towards comprehensive data management doesn't end there. CastorDoc takes it a step further by integrating advanced governance, cataloging, and lineage capabilities with a user-friendly AI assistant, offering a powerful solution for businesses aiming to enable self-service analytics. Whether you're looking to streamline your data governance lifecycle or empower your business users to harness data with confidence, CastorDoc is designed to revolutionize your data management practices. To explore more tools that can complement your modern data stack and to see how CastorDoc stands out in the landscape, check out more tools comparisons here.
You might also like
Get in Touch to Learn More
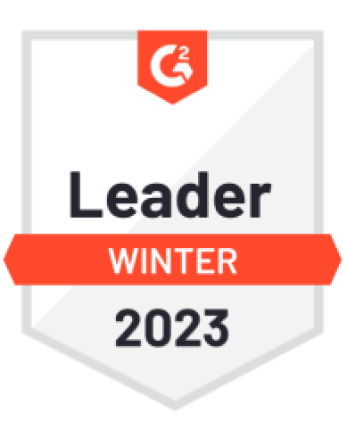
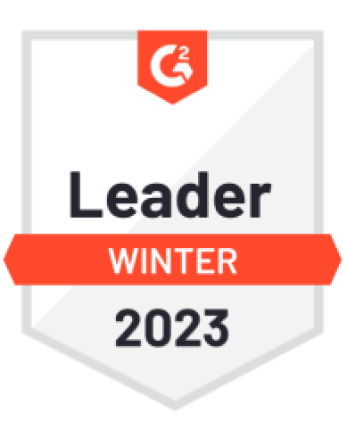

“[I like] The easy to use interface and the speed of finding the relevant assets that you're looking for in your database. I also really enjoy the score given to each table, [which] lets you prioritize the results of your queries by how often certain data is used.” - Michal P., Head of Data