Data Observability Tool Comparison: Soda vs. Sifflet
Data observability is a critical aspect of data management that ensures the quality, reliability, and trustworthiness of data. The ability to monitor, detect, and address issues with data is crucial in today's data-driven world. In this article, we will compare two popular data observability tools - Soda and Sifflet - to help you make an informed decision about which tool best meets your needs.
Understanding Data Observability
Data observability is the practice of monitoring and analyzing data to ensure its accuracy, completeness, and consistency. It involves ensuring that data meets predefined quality standards, conforming to data governance policies, and uncovering potential issues before they impact critical business processes.
But what exactly does it mean to have data observability? Let's dive deeper into the importance of this practice and explore the key features of data observability tools.
The Importance of Data Observability
Data observability plays a crucial role in maintaining data integrity and driving informed decision-making. By providing real-time insights into data quality, observability enables organizations to identify and rectify issues promptly, resulting in improved data accuracy, trust, and reliability.
Imagine a scenario where a company is relying on inaccurate sales data to make important business decisions. Without data observability, they might not even realize that the data is flawed until it's too late. This could lead to costly mistakes and missed opportunities. With data observability, organizations can proactively monitor and validate their data, ensuring that it is reliable and trustworthy.
Data observability also enhances data governance, ensuring compliance with regulations and industry standards. It enables organizations to monitor both structured and unstructured data, mitigating the risk of data breaches and maintaining data privacy. This is particularly important in industries that handle sensitive information, such as healthcare or finance.
Key Features of Data Observability Tools
Data observability tools offer a wide range of features to help organizations effectively monitor, measure, and manage their data. These features vary from tool to tool, but there are some common functionalities that you should consider when evaluating data observability tools:
- Data quality monitoring: Tools should provide comprehensive monitoring capabilities to detect and flag data issues in real-time. This includes checking for data inconsistencies, missing values, or outliers that could impact the accuracy of analysis.
- Alerting and reporting: The ability to set up automated alerts and generate detailed reports is essential for timely issue resolution and performance tracking. This ensures that any data issues are promptly addressed, minimizing the impact on business operations.
- Integration and compatibility: Tools should seamlessly integrate with existing data infrastructure, including data storage systems, databases, and data pipelines. This allows for a streamlined data observability process without disrupting existing workflows.
- Data profiling and anomaly detection: Advanced tools use machine learning algorithms to identify patterns and anomalies in data, enabling organizations to take proactive measures to maintain data quality. By detecting unusual data patterns, organizations can investigate potential issues and prevent data discrepancies before they become significant problems.
- Collaboration and data lineage: Some data observability tools offer collaboration features that allow teams to work together to resolve data issues. Additionally, data lineage capabilities provide visibility into the origin and transformation of data, helping organizations trace and understand data changes over time.
By considering these key features, organizations can choose data observability tools that best align with their specific needs and requirements.
An Introduction to Soda
Soda is a powerful data observability solution that helps organizations ensure the quality and accuracy of their data. It offers a range of features designed to monitor, analyze, and validate data across various data sources.
Overview of Soda's Functionality
Soda provides comprehensive data quality monitoring capabilities, allowing organizations to detect and resolve issues proactively. It offers a user-friendly interface and intuitive dashboards that provide real-time insights into data quality metrics such as completeness, uniqueness, and consistency.
With Soda, organizations can set up automated alerts and notifications to promptly address data issues. The tool also enables users to create customizable reports that provide in-depth analysis and visualization of data quality trends over time.
Pros and Cons of Soda
Soda has several advantages that make it a popular choice for data observability:
- Easy to use: Soda's user-friendly interface and intuitive features make it accessible to both technical and non-technical users.
- Robust data monitoring: Soda's comprehensive monitoring capabilities help organizations identify and address data issues before they impact critical processes.
- Customizable reporting: The tool allows users to create visually appealing reports that provide clear insights into data quality metrics.
Despite its strengths, Soda does have a few limitations:
- Limited data profiling capabilities: While Soda offers basic profiling functionalities, organizations that require advanced anomaly detection may need to consider additional tools.
- Integration challenges: Some users have reported challenges when integrating Soda with certain data sources and pipelines, requiring additional customization.
An Introduction to Sifflet
Sifflet is a comprehensive data observability tool that enables organizations to monitor and manage the quality of their data effectively. It offers a wide range of features designed to detect and address data issues in real-time.
Overview of Sifflet's Functionality
Sifflet provides a holistic approach to data observability, allowing organizations to monitor data quality across multiple dimensions. It offers a user-friendly interface that allows users to define customized data quality rules and metrics based on their specific requirements.
The tool provides real-time monitoring capabilities, alerting users to potential data issues promptly. It also offers comprehensive data profiling features, enabling users to analyze patterns, detect anomalies, and take corrective actions proactively.
Pros and Cons of Sifflet
Sifflet offers several advantages that make it a compelling choice for data observability:
- Flexible data quality rules: Sifflet allows users to define custom data quality rules and metrics, ensuring that the tool aligns with specific business requirements.
- Advanced data profiling: Sifflet's advanced data profiling capabilities enable organizations to detect complex patterns and anomalies for improved data quality management.
- Seamless integration: Sifflet integrates smoothly with various data storage systems, databases, and other data processing tools, reducing implementation complexities.
However, Sifflet does have a few areas where organizations may encounter challenges:
- Learning curve: Some users have reported a relatively steeper learning curve when getting started with Sifflet's advanced features and functionalities.
- Pricing structure: Sifflet's pricing structure may not be suitable for organizations with budget constraints or smaller-scale data operations.
Detailed Comparison of Features
Data Monitoring Capabilities
Both Soda and Sifflet offer robust data monitoring capabilities, allowing organizations to track and analyze data quality metrics in real-time.
Soda focuses on providing an intuitive dashboard that offers clear insights into data quality indicators such as completeness, uniqueness, accuracy, and consistency. The tool enables users to set up automated alerts and notifications, making it easier to detect and address potential issues promptly.
Sifflet, on the other hand, offers a more comprehensive approach to data monitoring. It allows users to define customized data quality rules and metrics, facilitating granular monitoring and identification of specific data issues. Sifflet's real-time monitoring capabilities and advanced anomaly detection algorithms help organizations identify and address complex data quality challenges.
Alerting and Reporting Features
Both Soda and Sifflet offer robust alerting and reporting features to facilitate timely issue resolution and performance tracking.
Soda enables users to create customizable reports that provide clear insights into data quality trends over time. Its alerting capabilities allow users to set up automated notifications for critical data issues, ensuring prompt action.
Sifflet's reporting features offer more flexibility, allowing users to define custom report templates and visualize data quality metrics through intuitive visualizations. The tool's alerting capabilities go beyond basic notifications, allowing users to define complex alert rules based on specific data quality thresholds.
Integration and Compatibility
Integration and compatibility are crucial factors to consider when selecting a data observability tool. Both Soda and Sifflet offer seamless integration with popular data storage systems, databases, and data pipelines.
Soda provides connectors and APIs that enable easy integration with various data sources, allowing organizations to monitor data quality across their entire data ecosystem. However, some users have reported challenges when integrating Soda with certain proprietary or legacy data systems, requiring additional customization.
Sifflet, on the other hand, offers out-of-the-box compatibility with a wide range of data sources, making it easier to collect and analyze data from different systems. Its flexible architecture allows for easy integration with existing data pipelines and workflows, reducing implementation complexities.
Pricing and Value for Money
Soda's Pricing Structure
Soda offers a subscription-based pricing model, with different tiers based on the size of the organization and the volume of data being monitored. The pricing is transparent, with no hidden costs. Organizations can choose the plan that best suits their needs and scale as their data operations grow.
Soda's pricing is generally considered to be reasonable, considering the features and capabilities it offers. However, organizations with tighter budgets or smaller-scale data operations may find the cost relatively higher compared to other tools in the market.
Sifflet's Pricing Structure
Sifflet offers a flexible pricing structure tailored to the specific requirements of each organization. The pricing is generally based on factors such as the volume of data being monitored, the complexity of the data quality rules, and the level of support required.
While Sifflet's pricing may vary depending on individual needs, it is generally considered to be on the higher end of the spectrum. This may make it less accessible for organizations with limited budgets or smaller-scale data operations.
Conclusion
Choosing the right data observability tool is critical for ensuring the quality, reliability, and trustworthiness of your data. Both Soda and Sifflet offer a range of features and capabilities to help organizations monitor, analyze, and manage data quality effectively.
Soda provides a user-friendly interface and comprehensive monitoring capabilities, making it a suitable choice for organizations looking for an easy-to-use tool that provides real-time insights into data quality metrics. Despite some limitations, Soda offers a solid foundation for data observability.
Sifflet, on the other hand, offers advanced data profiling and monitoring features, making it a great choice for organizations with more complex data quality requirements. However, the higher price point and potential learning curve may be factors to consider.
In the end, the choice between Soda and Sifflet depends on your specific business needs, budget, and scalability requirements. By carefully evaluating the features, pros, and cons of each tool, you can make an informed decision that aligns with your data observability goals.
While Soda and Sifflet offer valuable features for data observability, CastorDoc takes data management to the next level by integrating advanced governance, cataloging, and lineage capabilities with a user-friendly AI assistant. CastorDoc's powerful platform is designed for businesses seeking to enable self-service analytics and empower their teams with complete control and visibility over the data governance lifecycle. Whether you're a data professional looking to streamline compliance and quality assurance or a business user aiming to access and understand data with ease, CastorDoc is tailored to meet your needs. To explore how CastorDoc compares to other tools and how it can revolutionize your data management, check out more tools comparisons here.
You might also like
Get in Touch to Learn More
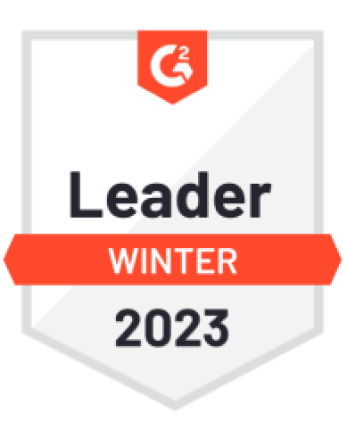
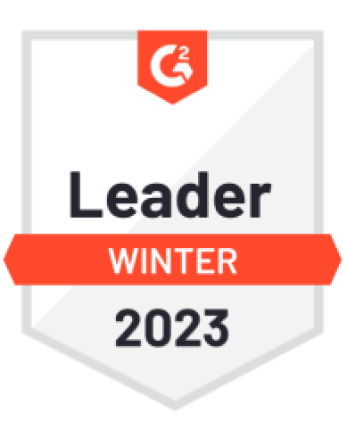

“[I like] The easy to use interface and the speed of finding the relevant assets that you're looking for in your database. I also really enjoy the score given to each table, [which] lets you prioritize the results of your queries by how often certain data is used.” - Michal P., Head of Data