Data Observability Tool Comparison: Lantern vs. Datafold
Data observability has become increasingly critical in today's data-driven world. It allows organizations to gain valuable insights, ensure data accuracy, and maintain data quality. Two prominent data observability tools in the market are Lantern and Datafold. In this article, we will compare these two tools, examining their key features, pros and cons, and pricing structures.
Understanding Data Observability
Before diving into the specifics of Lantern and Datafold, let's first understand the concept of data observability. Put simply, data observability refers to the ability to measure, track, and monitor data quality, accuracy, and reliability. It involves ensuring that data pipelines and processes are efficient, reliable, and error-free, ultimately enabling organizations to make informed decisions based on trustworthy data.
Data observability is not just about monitoring data; it's also about understanding the context in which the data is generated and used. This includes tracking data lineage, understanding data transformations, and ensuring data security and compliance throughout the entire data lifecycle.
The Importance of Data Observability
Data observability plays a vital role in maintaining the integrity and reliability of data. By having reliable data, businesses can improve decision-making, identify anomalies quickly, and prevent potential data-related issues. With the increasing volume and complexity of data, the importance of data observability cannot be overstated.
Furthermore, data observability is crucial for meeting regulatory requirements and ensuring data governance. Organizations need to have full visibility into their data processes to comply with regulations such as GDPR, HIPAA, and CCPA. Data observability tools help in providing the necessary audit trails and documentation to demonstrate compliance.
Key Features of Data Observability Tools
Both Lantern and Datafold offer a wide range of features to ensure thorough data observability. Let's explore some of their key features in greater detail.
Lantern provides real-time monitoring of data pipelines, anomaly detection, and customizable alerts to notify users of any issues. On the other hand, Datafold focuses on data profiling, schema validation, and impact analysis to help users understand the quality and implications of their data changes. These tools empower data engineers and analysts to proactively manage and optimize their data workflows for better decision-making.
An Introduction to Lantern
Lantern, a popular data observability tool, has gained recognition for its powerful features and user-friendly interface. It provides organizations with comprehensive visibility into their data pipelines, enabling them to detect and rectify problems swiftly.
With the increasing complexity of data ecosystems, organizations are constantly seeking tools that can help them manage and monitor their data effectively. Lantern has emerged as a trusted solution, offering a wide range of features that cater to the needs of data-driven enterprises.
Overview of Lantern's Features
Lantern offers several notable features. Firstly, it provides real-time monitoring, allowing users to track data flow and identify bottlenecks or inconsistencies instantly. This feature is particularly valuable in fast-paced environments where timely detection of issues can prevent costly disruptions.
Additionally, Lantern offers data quality checks, ensuring data accuracy and integrity throughout the pipeline. By implementing various validation techniques, such as schema checks and data profiling, Lantern helps organizations maintain high-quality data, reducing the risk of errors and inaccuracies.
It also provides anomaly detection capabilities, empowering users to identify and address outliers in the data. Leveraging advanced statistical algorithms and machine learning techniques, Lantern can detect unusual patterns or deviations from expected behavior, enabling proactive troubleshooting and data cleansing.
Furthermore, Lantern supports a variety of data storage platforms and data formats, enabling seamless integration into existing data infrastructure. Whether organizations store their data in traditional relational databases, cloud-based data warehouses, or even big data platforms, Lantern can connect and extract insights from these diverse sources.
Moreover, Lantern offers customizable alerts and notifications, ensuring that users stay informed about critical issues. By setting up personalized alerts based on specific conditions or thresholds, users can receive timely notifications via email, Slack, or other communication channels, enabling them to take immediate action.
Pros and Cons of Using Lantern
While Lantern offers many advantages, it is essential to consider its limitations. One significant advantage is its user-friendly interface, which makes it accessible to a wide range of users. Even those without extensive technical expertise can navigate the tool and derive meaningful insights from their data.
Lantern's ability to support different data storage platforms increases its versatility and compatibility. Organizations can leverage Lantern's capabilities without worrying about data silos or compatibility issues, as it seamlessly integrates with various systems and formats.
However, some users have expressed concerns about Lantern's performance with larger datasets. While it performs well with moderate data volumes, organizations dealing with vast amounts of data may experience slower processing times. It is crucial for enterprises to assess their data volume and processing requirements before implementing Lantern to ensure optimal performance.
Additionally, some users have reported occasional hiccups in the anomaly detection functionality. While Lantern's algorithms are designed to detect anomalies accurately, there may be instances where false positives or false negatives occur. Organizations should carefully evaluate the effectiveness of the anomaly detection feature based on their specific use cases and data characteristics.
In conclusion, Lantern is a powerful data observability tool that offers a range of features designed to enhance data visibility and streamline data management processes. By leveraging Lantern's real-time monitoring, data quality checks, anomaly detection capabilities, and seamless integration with various data storage platforms, organizations can gain valuable insights and ensure the reliability and accuracy of their data. However, it is essential to consider the tool's limitations, such as performance with larger datasets and occasional hiccups in anomaly detection, to make informed decisions about its implementation.
An Introduction to Datafold
Datafold is another prominent data observability tool that excels in providing data accuracy and consistency insights. It focuses on helping organizations detect and rectify data anomalies effectively.
Overview of Datafold's Features
Datafold's feature set provides comprehensive visibility and control over data pipelines. One standout feature is its data profiling capabilities, which allow users to gain insights into their data characteristics, such as data distribution, completeness, and consistency.
Furthermore, Datafold integrates seamlessly with popular data platforms, such as Apache Kafka and Apache Airflow. It also offers automated data testing, facilitating data validation against predefined rules and expectations. Another notable feature is its data lineage tracking, enabling users to trace data transformations and ensure data integrity across various stages of the pipeline.
Pros and Cons of Using Datafold
Datafold offers several advantages, making it a compelling choice for organizations seeking robust data observability. The tool's data profiling capabilities provide valuable insights, enabling users to understand their data better and uncover potential issues promptly.
However, some users have reported that Datafold's user interface can be overwhelming, especially for new users. While it offers powerful features, the learning curve may be steeper compared to other tools. Additionally, data integration with certain platforms may require additional configuration and setup time.
In-Depth Comparison: Lantern vs. Datafold
Now that we have explored the individual features of Lantern and Datafold, let's compare them in greater detail to understand their differences and similarities.
Comparing User Interface and Experience
Both Lantern and Datafold have intuitive interfaces, but their overall design differs slightly. Lantern's interface emphasizes simplicity and ease of use, making it accessible to users with varying levels of technical expertise. On the other hand, Datafold's interface offers extensive customization options and in-depth insights, targeting users with a deeper understanding of data analysis.
Comparing Data Analysis Capabilities
When it comes to data analysis, Lantern focuses on real-time monitoring and anomaly detection. While it provides robust capabilities in these areas, its analysis features may be limited compared to Datafold. Datafold's emphasis on data profiling and lineage tracking offers a comprehensive view of data quality, delivering more in-depth analysis capabilities.
Comparing Integration and Compatibility
Both Lantern and Datafold integrate well with various data platforms and formats, ensuring compatibility with existing data infrastructure. However, Datafold's ability to seamlessly integrate with Apache Kafka and Apache Airflow gives it an edge in terms of compatibility with popular tools in the data ecosystem.
Pricing: Lantern vs. Datafold
When considering a data observability tool, pricing is an important factor. Let's examine the pricing structures of both Lantern and Datafold.
Pricing Structure of Lantern
Lantern offers a subscription-based pricing model, with different tiers based on the organization's needs. The pricing is determined by factors such as data volume, number of users, and additional features. Users can choose a plan that aligns with their requirements, ensuring flexibility and scalability.
Pricing Structure of Datafold
Datafold also adopts a subscription-based pricing structure. Similar to Lantern, Datafold offers different tiers that cater to varying organizational needs. Pricing factors include data volume, number of users, and additional features. The tiered pricing model allows organizations to select a plan that suits their requirements and budget.
It's worth noting that as data observability tools evolve and organizations' requirements change, it is crucial to regularly evaluate pricing plans to ensure cost-effectiveness and adaptability.
Conclusion
In the realm of data observability, both Lantern and Datafold stand out as powerful tools. Lantern's emphasis on user-friendliness and real-time monitoring makes it an attractive choice for organizations seeking a straightforward yet effective solution. On the other hand, Datafold's comprehensive data profiling and lineage tracking capabilities make it a strong contender for organizations looking for in-depth data analysis.
Ultimately, the choice between Lantern and Datafold depends on specific business needs, the complexity of data pipelines, and the importance placed on various features. By carefully considering the features, pros and cons, and pricing structures of both tools, organizations can make an informed decision to enhance their data observability efforts and drive better business outcomes.
As you consider enhancing your data observability with tools like Lantern and Datafold, don't overlook the transformative capabilities of CastorDoc. With its advanced governance, cataloging, and lineage features, combined with a user-friendly AI assistant, CastorDoc stands out as a comprehensive solution for businesses aiming to enable self-service analytics. Whether you're a data professional seeking complete control over the data governance lifecycle or a business user desiring to access and understand data with ease, CastorDoc caters to all your needs. Experience a revolution in data management and unlock the full potential of your data for informed decision-making across your enterprise. Check out more tools comparisons here and discover how CastorDoc can elevate your organization's data observability and governance.
You might also like
Get in Touch to Learn More
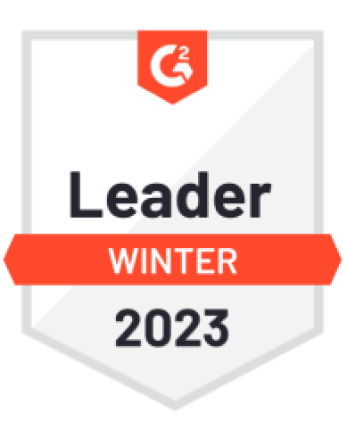
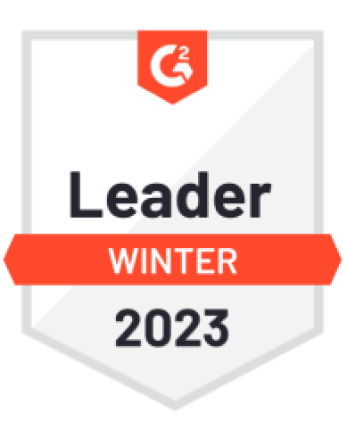

“[I like] The easy to use interface and the speed of finding the relevant assets that you're looking for in your database. I also really enjoy the score given to each table, [which] lets you prioritize the results of your queries by how often certain data is used.” - Michal P., Head of Data