Embedding Analytics for Better Business Decisions: The Power of Embedded Analytics
Learn how embedding analytics into your business processes can revolutionize your decision-making.
In today's business landscape, the ability to make data-driven decisions is crucial for success. With the ever-increasing availability of data, organizations need effective tools and strategies to harness its potential. This is where embedded analytics comes into play. Embedded analytics refers to the practice of integrating analytics capabilities directly into business applications, providing users with real-time insights and empowering them to make informed decisions.
Understanding Embedded Analytics
First, let's delve deeper into the concept of embedded analytics. In simple terms, it is the process of seamlessly integrating analytical capabilities into existing business systems and software applications. This allows users to access and analyze data without the need for separate tools or platforms. By embedding analytics, organizations can provide their stakeholders with a unified experience, enabling them to derive valuable insights without disrupting their workflow.
Definition and Importance of Embedded Analytics
Embedded analytics refers to the integration of analytical functions, such as reporting, data visualization, and predictive modeling, directly into operational applications. It eliminates the need for users to switch between different tools, streamlining the decision-making process. The importance of embedded analytics lies in its ability to provide real-time insights, leading to faster and more accurate decision-making.
How Embedded Analytics Enhances Business Decisions
The integration of analytics capabilities into business applications presents several benefits for decision-making. Firstly, it empowers users by providing them with relevant data and insights at their fingertips. Instead of relying on static reports or cumbersome data exports, stakeholders can access real-time information within the context of their work. This enables them to make timely decisions and take immediate action when needed.
Furthermore, embedded analytics facilitates data-driven collaboration within an organization. With the ability to share and collaborate on insights directly within the application, teams can align their decision-making processes and work towards common goals. In addition, the insights derived from embedded analytics can drive innovation and strategic initiatives, helping organizations stay ahead of the competition.
Moreover, embedded analytics offers a personalized and tailored experience for users. By embedding analytics capabilities into existing applications, organizations can customize the user interface and functionality to match the specific needs of different user groups. This ensures that users have access to the most relevant and meaningful insights, enhancing their decision-making process.
Additionally, embedded analytics can improve operational efficiency by automating repetitive tasks and reducing manual effort. With embedded analytics, users can set up automated reports, alerts, and notifications, ensuring that they are always up-to-date with the latest insights. This not only saves time but also allows users to focus on more strategic and value-added activities.
Furthermore, embedded analytics enables organizations to leverage their existing infrastructure and investments. By integrating analytics capabilities into their current systems and applications, organizations can maximize the value of their technology stack without the need for additional investments or complex integrations. This makes embedded analytics a cost-effective solution for organizations looking to enhance their decision-making capabilities.
The Architecture of Embedded Analytics
Now, let's dive deeper into the architecture of embedded analytics and explore its key components. Understanding the underlying structure is crucial for designing and implementing an effective embedded analytics solution that empowers organizations with valuable insights.
Key Components of Embedded Analytics
An embedded analytics system typically consists of three key components: data integration, analytics engine, and visualization layer. Let's take a closer look at each of these components:
- Data Integration: This component plays a vital role in the embedded analytics ecosystem. It involves the extraction, transformation, and loading (ETL) process, which ensures that data from various sources is integrated into a central repository. By consolidating data from disparate sources, organizations can access a comprehensive and up-to-date dataset for analysis. This seamless integration enables users to gain a holistic view of their data, uncovering hidden patterns and trends.
- Analytics Engine: The analytics engine is the powerhouse of the embedded analytics system. It is responsible for applying advanced analytical algorithms and models to the integrated data. By leveraging cutting-edge techniques such as machine learning and predictive analytics, the analytics engine empowers users to perform complex analysis, generate reports, and uncover meaningful insights. This component acts as a catalyst for data-driven decision-making, enabling organizations to stay ahead of the competition.
- Visualization Layer: The visualization layer is the face of the embedded analytics system. It focuses on presenting the analysis results in a clear and intuitive manner. By utilizing data visualization techniques, such as charts, graphs, and interactive dashboards, organizations can transform complex data into visually appealing representations. This empowers users to explore the data, identify patterns, and gain actionable insights effortlessly. The visualization layer acts as a bridge between data and decision-makers, enabling effective communication and understanding.
Designing an Effective Embedded Analytics System
Designing an effective embedded analytics system requires careful consideration of various factors. Let's explore some key aspects that organizations need to keep in mind:
Identifying Key Metrics: Firstly, organizations need to identify the key metrics and performance indicators that align with their business goals. By understanding the specific metrics that drive success, organizations can ensure that the embedded analytics solution provides the right information to drive decision-making. This targeted approach enables organizations to focus on the most critical aspects of their business, leading to better outcomes.
User Experience and Usability: Next, the design of the embedded analytics system should prioritize user experience and usability. The interface should be intuitive and user-friendly, enabling users to navigate the analytics capabilities seamlessly. By providing a smooth and intuitive user experience, organizations can empower users to explore data, generate insights, and make informed decisions effortlessly. This user-centric approach enhances adoption and maximizes the value derived from the embedded analytics system.
Scalability, Security, and Integration: Additionally, organizations need to consider scalability, security, and integration with existing systems when designing an embedded analytics system. Scalability ensures that the system can handle increasing data volumes and user demands without compromising performance. Robust security measures, such as data encryption and access controls, safeguard sensitive information and protect against unauthorized access. Integration with existing systems, such as customer relationship management (CRM) or enterprise resource planning (ERP) systems, enables seamless data flow and enhances the overall efficiency of the organization.
By carefully considering these factors and leveraging the key components of embedded analytics, organizations can design and implement a robust and efficient system that empowers users with actionable insights, driving growth and success.
The Power of Embedded Analytics in Business
Embedded analytics has the potential to revolutionize how organizations leverage data for business intelligence. Let's explore some of the key advantages it offers.
Improving Business Intelligence with Embedded Analytics
Traditional business intelligence (BI) solutions often suffer from inefficiencies and delays due to the fragmentation of data and tools. Embedded analytics overcomes these limitations by integrating analytics directly into daily operations. This enables users to access real-time insights, empowering them to make informed decisions based on accurate and up-to-date data.
Embedded analytics also enhances data governance and compliance. By consolidating data within a centralized system, organizations can ensure data consistency and integrity. This not only improves data accuracy but also enables organizations to adhere to regulatory requirements and industry standards.
Predictive Capabilities of Embedded Analytics
Another powerful aspect of embedded analytics is its predictive capabilities. By leveraging advanced algorithms and machine learning models, organizations can uncover patterns and trends in their data. This enables them to make accurate predictions and forecasts, assisting in strategic planning, risk management, and proactive decision-making.
Through predictive analytics, businesses can identify future opportunities and mitigate potential risks. Whether it's forecasting sales trends, optimizing supply chain operations, or identifying customer behavior patterns, embedded analytics equips organizations with the tools to stay ahead in an increasingly competitive market.
Implementing Embedded Analytics
Now that we understand the significance of embedded analytics, let's explore the process of implementing it within your business.
Steps to Embed Analytics into Your Business
The implementation of embedded analytics requires a systematic approach. Here are some key steps to guide you:
- Evaluate Your Needs: Begin by assessing your organization's analytics requirements. Determine the specific insights you need to drive decision-making and align them with your business goals.
- Select the Right Tools and Technologies: Research and select the analytics tools and technologies that best match your needs. Consider factors such as scalability, ease of use, integration capabilities, and vendor support.
- Data Integration: Establish a robust data integration process to consolidate data from various sources into a centralized repository. Ensure data quality, integrity, and accessibility.
- Analytics Engine Configuration: Configure the analytics engine to perform the necessary analysis and generate relevant insights. This may involve defining metrics, creating models, and setting up predictive algorithms.
- Visualization and Reporting: Design and deploy a visualization layer that presents the analysis results in a user-friendly and intuitive manner. Develop interactive dashboards and reports that cater to different user roles and requirements.
- User Training and Adoption: Lastly, train your users to effectively utilize the embedded analytics capabilities. Encourage adoption by showcasing the benefits and providing ongoing support and guidance.
Overcoming Challenges in Implementing Embedded Analytics
While the benefits of embedded analytics are substantial, implementation can present challenges. It is crucial to anticipate and address these challenges proactively. Common obstacles include data integration complexities, resource constraints, limited user acceptance, and maintaining data security. By planning ahead and involving key stakeholders, organizations can overcome these challenges and ensure a successful implementation.
Future Trends in Embedded Analytics
As technology continues to evolve, embedded analytics is poised to witness further advancements. Let's explore some key trends shaping its future.
The Role of AI and Machine Learning in Embedded Analytics
Artificial intelligence (AI) and machine learning (ML) are rapidly transforming industries across the globe. In the realm of embedded analytics, AI and ML offer exciting opportunities for enhanced insights and automation. AI-powered analytics engines can analyze vast amounts of data, identify patterns, and make data-driven recommendations. This empowers users to make more accurate decisions and drives innovation within organizations.
The Impact of Big Data on Embedded Analytics
The explosion of big data has revolutionized the way organizations capture, store, and analyze information. Embedded analytics enables businesses to leverage big data effectively by providing real-time analysis and insights. As big data continues to grow exponentially, embedded analytics will play a pivotal role in helping organizations unlock its full potential and gain a competitive edge.
Conclusion
In conclusion, embedding analytics into business applications is a powerful strategy for enhancing decision-making. It enables organizations to access real-time insights, improve business intelligence, leverage predictive capabilities, and drive innovation. By implementing embedded analytics and staying abreast of future trends in the field, businesses can gain a competitive advantage and make data-driven decisions with confidence.
Ready to harness the transformative power of embedded analytics and elevate your business decision-making? CastorDoc is here to guide you through the journey. As the most reliable AI Analytics Agent, CastorDoc empowers your teams with instantaneous, trustworthy data answers, enabling self-service analytics and breaking down data literacy barriers. Experience how CastorDoc can activate your data stack's ROI and give your business users the autonomy and trust they need to make informed decisions. Try CastorDoc today and start your path to data-driven excellence.
You might also like
Get in Touch to Learn More
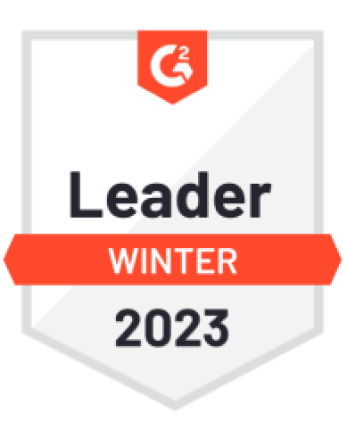
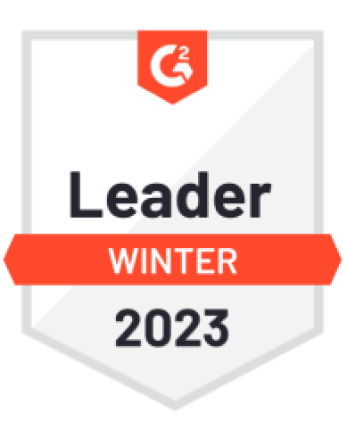

“[I like] The easy to use interface and the speed of finding the relevant assets that you're looking for in your database. I also really enjoy the score given to each table, [which] lets you prioritize the results of your queries by how often certain data is used.” - Michal P., Head of Data