How to Determine the Original Set of Data in Data Analysis
Learn how to accurately identify the original set of data in your data analysis process.
In the field of data analysis, determining the original set of data is of utmost importance. The accuracy and reliability of any analysis depend on accessing the authentic and unaltered raw data. However, with the abundance of data sources and the ease of manipulation, identifying the original data set can be a challenging task. In this article, we will delve into the significance of original data in analysis, discuss the steps to identify it, explore the challenges involved, and highlight the tools and techniques that can be employed for successful identification.
Understanding the Importance of Original Data in Analysis
Before delving into the intricacies of identifying the original data set, it is crucial to grasp why it holds such significance in the realm of data analysis. The essence lies in the fact that the validity of any analysis hinges upon the authenticity of the data used. Original data serves as the foundation upon which accurate and reliable conclusions can be drawn. By utilizing unadulterated data, analysts can uncover valuable insights and make informed decisions, driving businesses and research forward.
The Role of Original Data in Accurate Analysis
Original data acts as the starting point for any analysis. It forms the basis for statistical computations, hypothesis testing, and model building. By working with the actual data collected during the research or data acquisition process, analysts can ensure that their conclusions are based on real-world observations. This not only enhances the credibility of their findings but also enables them to make predictions and recommendations that are applicable in practical scenarios.
Why Original Data is Crucial for Reliable Results
Reliability is a cornerstone of data analysis. Researchers and analysts aim to generate results that can be replicated and validated. This requires working with the original data set to eliminate any potential biases, errors, or inconsistencies introduced through data manipulation or duplication. Only by accessing the unmodified data can analysts confidently claim the accuracy and dependability of their results, paving the way for further analysis or decision-making processes.
Moreover, original data allows for a more in-depth exploration of the underlying patterns and trends within the dataset. By examining the raw data, analysts can identify outliers, anomalies, and correlations that may not be apparent in pre-processed or summarized versions. This level of granularity enables a comprehensive understanding of the data, leading to more robust and nuanced analysis.
Furthermore, original data provides a valuable resource for future research and analysis. By preserving the integrity of the data, analysts contribute to the collective knowledge and understanding of a particular field or industry. This allows for the replication and extension of studies, fostering scientific progress and innovation. Additionally, original data can serve as a benchmark for comparison with new datasets, enabling researchers to identify changes, trends, or shifts in the data over time.
Steps to Identify the Original Set of Data
Identifying the original set of data necessitates a systematic approach to ensure the accuracy and integrity of the analysis. By following a series of steps, analysts can mitigate the risk of using inaccurate or altered data.
Recognizing Data Duplication
The first step in identifying the original data set is to detect and handle instances of data duplication. Duplicate data can arise due to various reasons such as system errors, multiple data sources, or mistakenly merged datasets. By employing appropriate techniques like matching algorithms or data profiling, analysts can identify and eliminate duplicated records or identify the original source of the duplicated data.
Tracing Data Sources
Tracing the sources of data is an essential step in determining the original data set. Understanding the provenance of data helps in ascertaining its reliability and credibility. By documenting the sources and capturing relevant metadata such as timestamps, data providers, and data collection methods, analysts can establish a trail that leads them back to the original data source.
Verifying Data Authenticity
Data authenticity refers to the assurance that the data has not been tampered with or modified during the data lifecycle. To ensure the authenticity of data, cryptographic techniques like hashing or digital signatures can be employed. By comparing the computed values with the original values, analysts can verify the integrity of the data and ensure that it has not been tampered with.
Once the data duplication has been addressed and the sources have been traced, analysts can delve deeper into the process of identifying the original set of data. This involves examining the data for any inconsistencies or anomalies that may indicate data manipulation or corruption.
An important aspect of this step is to analyze the data for any patterns or trends that may help in identifying the original data set. By conducting statistical analysis or using machine learning algorithms, analysts can uncover hidden patterns that can provide valuable insights into the authenticity of the data.
Furthermore, it is crucial to consider the context in which the data was collected. Understanding the purpose and objectives of the data collection process can shed light on the original data set. By consulting with domain experts or conducting interviews with data collectors, analysts can gain a deeper understanding of the data and its origins.
In addition to these steps, it is essential to establish robust data governance practices. This involves implementing data quality controls, ensuring data privacy and security, and maintaining a comprehensive data documentation process. By adhering to these practices, analysts can create a strong foundation for identifying the original set of data and ensuring the reliability of their analysis.
Challenges in Determining Original Data
Despite the criticality of identifying the original data set, several challenges can hinder the process. These challenges arise due to the ever-increasing volume of data, incomplete data records, concerns related to data privacy, and other factors that require careful consideration.
One of the primary challenges in determining the original data set is dealing with large data sets. As data volumes continue to explode, analyzing and determining the original data set becomes more arduous. Large data sets may involve multiple data sources, making it challenging to identify the original data. However, analysts can employ data profiling techniques or utilize advanced tools to efficiently navigate through vast amounts of data and separate the genuine data from duplicates or irrelevant information.
Another obstacle that analysts face is overcoming incomplete data records. Data records can often be incomplete due to various factors such as missing values or incomplete data collection processes. This can make it difficult to determine the original data set accurately. To overcome this challenge, analysts can adopt techniques like data imputation or data cleaning. These techniques help fill in missing values or complete incomplete records based on patterns or association rules, enabling a more comprehensive understanding of the original data set.
Addressing data privacy concerns is yet another challenge in determining the original data set. With the increasing focus on data privacy and protection, obtaining access to original data can become challenging. Data providers may impose restrictions or anonymization techniques to comply with privacy regulations. In such cases, analysts can leverage encryption methods or employ privacy-preserving techniques to work with the original data without compromising privacy requirements. By ensuring data security and privacy, analysts can confidently navigate the complexities associated with determining the original data set.
Tools and Techniques for Original Data Identification
To facilitate the identification of the original data set, various tools and techniques can be utilized, each catering to different aspects of the identification process.
When it comes to identifying the original data set, data analysis software is a valuable tool in an analyst's arsenal. These software solutions provide a comprehensive suite of tools designed to analyze, cleanse, and verify data authenticity. With functionalities like data profiling, data quality assessment, and data visualization, analysts can gain deep insights into the data, enabling them to identify the original data set efficiently. By utilizing data analysis software, analysts can navigate through complex data landscapes with ease, ensuring accurate identification of the original data.
In addition to data analysis software, data forensics techniques are also instrumental in the identification process. These techniques involve the use of investigative methods to uncover the authenticity and integrity of data. By employing data reconstruction, data validation, and data lineage analysis, analysts can pinpoint the original data with high accuracy. Drawing upon digital forensics principles, these techniques not only ensure the reliability of the data but also provide a means to reconstruct the data trail back to its original source. With data forensics techniques, analysts can confidently trace the origins of the data, making the identification process more robust.
Another valuable resource for data verification and identification is metadata. Metadata, which provides information about the data, plays a vital role in establishing the authenticity of the data. By examining metadata attributes such as data source, data creation dates, or data modification information, analysts can trace the origins of the data and verify its authenticity. Leveraging metadata platforms or frameworks further streamlines the process of identifying the original data set. With metadata as a guiding compass, analysts can navigate through vast amounts of data, ensuring accurate identification and verification.
Conclusion
In data analysis, the accuracy and reliability of results rest upon the quality and authenticity of the original data set. By understanding the importance of original data, following a systematic approach to identify it, overcoming the challenges involved, and utilizing appropriate tools and techniques, analysts can ensure that their analysis is based on trustworthy and unaltered data. Emphasizing the significance of original data in data analysis leads to reliable conclusions, informed decision-making, and ultimately, successful outcomes in various domains.
Ready to elevate your data analysis and ensure you're working with the original, unaltered datasets? CastorDoc is here to assist. As the most reliable AI Agent for Analytics, CastorDoc empowers your team to tackle strategic challenges with confidence. Experience the freedom of self-service analytics, enhance data literacy, and maximize your data stack's ROI. Activate your data's full potential and make informed decisions with ease. Try CastorDoc today and transform the way your business interacts with data.
You might also like
Get in Touch to Learn More
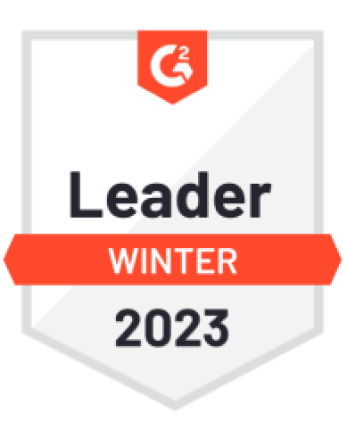
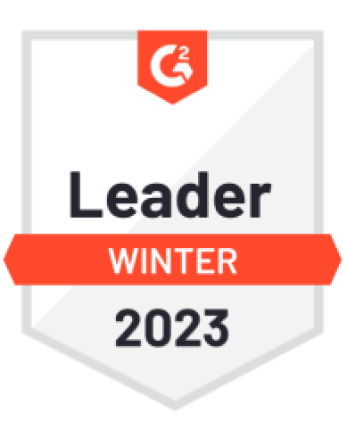

“[I like] The easy to use interface and the speed of finding the relevant assets that you're looking for in your database. I also really enjoy the score given to each table, [which] lets you prioritize the results of your queries by how often certain data is used.” - Michal P., Head of Data