Mastering Data Preparation for AI: Key Factors to Ensure Success
Learn how to effectively prepare data for AI applications with this guide.
Artificial intelligence (AI) is revolutionizing the way businesses operate, from automating tedious tasks to delivering valuable insights. However, the success of any AI project relies heavily on the quality and preparation of the underlying data. In this article, we will explore the key factors that are essential for mastering data preparation for AI and ensuring success in your projects.
Understanding Data Preparation for AI
The Importance of Clean and Structured Data
One of the critical aspects of data preparation for AI is the cleanliness and structure of your data. Clean data is free from errors, duplicates, and inconsistencies, which can adversely affect the accuracy and reliability of AI models. Structured data, on the other hand, is organized in a consistent format that allows for efficient processing and analysis. By ensuring your data is both clean and structured, you lay the foundation for successful AI implementation.
Data Quality Assessment for AI Readiness
Before diving into AI implementation, it is crucial to assess the quality of your data. Conducting a comprehensive data quality assessment helps identify any gaps or deficiencies that could hamper AI readiness. This assessment should evaluate factors such as completeness, accuracy, integrity, and relevance. By understanding the quality of your data, you can make informed decisions on the steps needed to enhance its suitability for AI.
Data Integration for AI Success
AI projects often require combining data from multiple sources to gain deeper insights and improve decision-making. Data integration plays a crucial role in this process. It involves merging and consolidating data from various systems and formats into a unified dataset. By integrating your data effectively, you enable seamless analysis and derive enhanced value from AI models.
Furthermore, data integration can also help in enriching your dataset by bringing in external data sources that provide additional context or insights. This enriched dataset can lead to more robust AI models with a broader understanding of the problem domain. Additionally, data integration allows for real-time data updates, ensuring that your AI models are constantly fed with the latest information for accurate predictions and decisions.
Another benefit of data integration in AI projects is the ability to create a holistic view of your organization's data landscape. By consolidating data from various departments and systems, you can break down data silos and uncover hidden patterns or correlations that were previously not apparent. This comprehensive view enables data-driven decision-making at a strategic level, driving innovation and competitive advantage in the AI space.
Key Factors in Identifying AI-Ready Data
Data Consistency and Accuracy
Consistency and accuracy are vital aspects of identifying AI-ready data. Consistency ensures uniformity in data formats, definitions, and conventions, enabling reliable analysis. For example, imagine you are working with a dataset that contains information about customer purchases. Consistency in this context would mean that the data consistently follows the same format, such as using the same units of measurement or date format. This uniformity allows AI algorithms to process the data effectively and draw meaningful insights.
Accuracy, on the other hand, guarantees that the data is both correct and precise. Inaccurate or imprecise data can lead to flawed AI models and unreliable predictions. To ensure accuracy, organizations often implement a rigorous data validation process. This process involves checking the data against known sources, conducting data quality assessments, and performing statistical analyses. By validating the accuracy of the data, you can have confidence in the results generated by AI applications.
Data Accessibility and Compatibility
To harness the power of AI effectively, your data should be easily accessible and compatible with the AI platform or tools you plan to use. Accessibility ensures that relevant stakeholders can retrieve and work with the data efficiently. For instance, imagine you are a data scientist working on an AI project. Easy accessibility means that you can quickly access the data you need without any unnecessary delays or obstacles. This accessibility allows you to focus on analyzing the data and deriving valuable insights.
Compatibility, on the other hand, ensures seamless integration between your data and AI systems, avoiding any technical challenges or limitations. For example, if you are using a specific AI platform that requires data in a particular format, ensuring compatibility means transforming your data to meet those requirements. This compatibility enables smooth data integration and enhances the overall efficiency of your AI workflows.
Data Security and Privacy Compliance
Protecting the security and privacy of your data is paramount, especially in the age of AI. Working with sensitive or personal data necessitates strict adherence to data protection regulations and industry standards. Implementing robust security measures, data anonymization techniques, and ensuring compliance with privacy regulations safeguards against unauthorized access and misuse of data.
For instance, imagine you are working with healthcare data that contains sensitive patient information. Ensuring data security involves implementing encryption protocols, access controls, and secure storage systems. Additionally, complying with privacy regulations such as the General Data Protection Regulation (GDPR) ensures that individuals' rights and privacy are respected when using AI applications. By prioritizing data security and privacy compliance, organizations can build trust with their stakeholders and mitigate potential risks associated with AI implementation.
Data Scalability and Flexibility
As your AI initiatives grow and evolve, so does the volume and diversity of data. Scalability and flexibility are key factors in ensuring your data can handle this growth. Scalability refers to the ability to process increasing amounts of data without impacting performance. For example, imagine you are working on a project that involves analyzing large-scale sensor data from an Internet of Things (IoT) network. Scalability in this context means that your data infrastructure can handle the exponential increase in data volume without experiencing significant slowdowns or bottlenecks.
Flexibility enables seamless integration of new data sources or modifications to existing ones. As new data sources become available or existing ones undergo changes, your data infrastructure should be able to adapt accordingly. For example, if you are incorporating social media data into your AI models, flexibility means that your data infrastructure can easily incorporate and process this new data source. By designing your data infrastructure with scalability and flexibility in mind, you can future-proof your AI initiatives and ensure they can handle the growing demands of data processing.
Assessing Your Data's AI Suitability
Evaluating Data Readiness for AI Implementation
Before investing time and resources in an AI project, it is crucial to evaluate your data's readiness for implementation. This evaluation involves examining if your data meets the necessary quality standards, is fit for the intended purpose, and aligns with the specific AI algorithms or models you plan to employ. Assessing your data's suitability helps identify any gaps or challenges that need to be addressed before proceeding with AI integration.
One important aspect to consider when assessing data readiness for AI implementation is the data labeling process. Labeled data is essential for training AI models, as it provides the necessary ground truth for the algorithms to learn from. Ensuring accurate and comprehensive data labeling is crucial for the success of an AI project, as it directly impacts the model's performance and predictive capabilities.
Common Challenges in Data Preparation for AI
Preparing data for AI can be a complex endeavor, often accompanied by various challenges. Some common hurdles include data silos, incompatible formats, missing values, insufficient data volume, and biases. Understanding these challenges and devising appropriate strategies to overcome them is crucial for successful data preparation and seamless AI deployment.
Another challenge in data preparation for AI is ensuring data privacy and security. With the increasing focus on data protection and privacy regulations, such as GDPR and CCPA, organizations need to ensure that the data used for AI training is handled in a compliant and secure manner. Implementing robust data governance practices and encryption techniques can help mitigate risks associated with data privacy and security breaches.
Steps to Optimize Data for AI Deployment
Data Cleaning and Preprocessing Techniques
Data cleaning and preprocessing are essential steps in optimizing your data for AI deployment. Cleaning involves removing errors, outliers, and irrelevant information, while preprocessing encompasses tasks like normalization, feature engineering, and handling missing values. By applying these techniques, you streamline your data to make it more suitable and effective for AI algorithms.
Implementing Data Governance Practices
Data governance involves establishing policies, processes, and controls to ensure the quality, security, and privacy of data. Implementing strong data governance practices is crucial in the context of AI, as it helps maintain data integrity, compliance, and accountability. By defining clear roles, responsibilities, and guidelines, you create a solid foundation for successful data preparation and AI deployment.
Leveraging Data Management Tools for AI Integration
The proliferation of data management tools provides invaluable assistance in data preparation for AI. These tools offer functionalities such as data profiling, data cleansing, data integration, and data governance, simplifying complex tasks and accelerating the data preparation process. By leveraging these tools, you can streamline your data preparation efforts and optimize your AI integration workflows.
Conclusion
Mastering data preparation for AI is an essential element in ensuring the success of your AI projects. By understanding the importance of clean and structured data, identifying AI-ready data factors, assessing your data's suitability, and taking appropriate optimization steps, you pave the way for effective AI implementation. Embracing these key factors positions your organization to unlock the full potential of AI and drive transformative outcomes.
Ready to elevate your data preparation and harness the full power of AI in your business? Try CastorDoc today and experience a seamless integration of advanced governance, cataloging, and lineage capabilities with the convenience of a user-friendly AI assistant. CastorDoc is not just a tool; it's your AI copilot, guiding you through the complexities of data management and enabling self-service analytics that drive informed decision-making. Whether you're a data professional seeking control and visibility or a business user desiring accessible and understandable data, CastorDoc is your partner in unlocking the transformative outcomes of AI. Don't wait to transform your data governance—start your journey with CastorDoc today.
You might also like
Get in Touch to Learn More
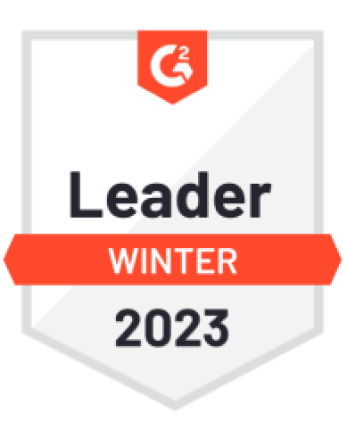
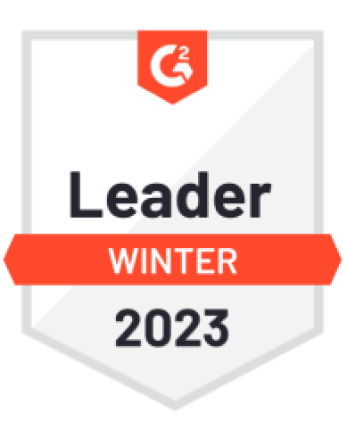

“[I like] The easy to use interface and the speed of finding the relevant assets that you're looking for in your database. I also really enjoy the score given to each table, [which] lets you prioritize the results of your queries by how often certain data is used.” - Michal P., Head of Data