Understanding the Modern Data Stack: From Data Warehouses to AI Analytics
Uncover the intricacies of the modern data stack with this comprehensive guide.
Navigating the complexities of the modern data stack has become essential for businesses to thrive. With an increasing volume and variety of data sources, organizations are looking for ways to streamline insights, harness the power of artificial intelligence (AI), and make data-driven decisions with ease. In this ultimate guide, we will demystify the modern data stack, explore the role of automation and natural language processing (NLP) in data analysis, delve into the world of machine learning, and uncover best practices for leveraging data warehouses and data lakes. By the end, you will have a comprehensive understanding of the components that make up a modern data stack, and how they can be integrated into your data strategy.
Demystifying the Modern Data Stack
Before we plunge into the intricacies of the modern data stack, let's take a moment to understand its core components. A modern data stack typically consists of three key elements: the data source, data engineering, and data visualization. A data source refers to the various platforms or systems that generate or collect data. These can include customer relationship management (CRM) tools, enterprise resource planning (ERP) systems, social media platforms, and more. Data engineering involves cleaning, transforming, and structuring the raw data into a more usable format. Finally, data visualization enables users to explore, analyze, and present the data in a visually appealing and easily understandable manner.
Over the years, the data stack has evolved significantly. In the past, organizations relied on traditional data stacks, which comprised on-premises infrastructure and slow batch processing. However, with the advent of cloud computing and real-time data processing, modern data stacks have emerged as a game-changer. These contemporary stacks offer scalability, flexibility, and faster processing capabilities, empowering organizations to make data-driven decisions with agility. Understanding the evolution of data stacks is crucial to grasp the full potential they bring to the table.
One of the key advantages of modern data stacks is their ability to handle diverse data types. Whether it's structured data from databases, semi-structured data like JSON files, or unstructured data from sources like social media feeds, modern data stacks are designed to ingest, process, and analyze a wide range of data formats. This versatility is essential in today's data-rich environment, where organizations need to extract insights from a variety of sources to stay competitive.
Furthermore, modern data stacks often incorporate machine learning and artificial intelligence capabilities to enhance data processing and analysis. By leveraging algorithms and models, these advanced data stacks can automate tasks such as anomaly detection, predictive analytics, and pattern recognition. This integration of AI technologies not only streamlines data workflows but also uncovers valuable insights that might have been overlooked with traditional approaches. As organizations continue to harness the power of AI, modern data stacks play a pivotal role in driving innovation and unlocking new possibilities in the realm of data analytics.
Streamlining Insights with Automated Processes
In today's fast-paced business environment, time is of the essence. The role of automation in data analysis cannot be overstated. By automating repetitive tasks, organizations can save valuable time, reduce human errors, and focus on more strategic and high-value activities. Automation can range from data extraction and transformation to report generation and anomaly detection. By embracing automated insights, decision-makers can access real-time data, uncover valuable patterns and trends, and make informed decisions that drive business success.
One of the key benefits of automated insights is the ability to leverage AI algorithms to uncover complex patterns within large datasets. These algorithms can unveil hidden relationships, identify anomalies, and provide recommendations for optimization. The power of automation combined with AI has the potential to revolutionize the way we analyze and interpret data, making it more accessible and impactful.
Moreover, automated processes can enhance collaboration within an organization by providing a centralized platform for data sharing and analysis. By streamlining workflows and enabling real-time collaboration, teams can work more efficiently and make data-driven decisions collectively. This collaborative approach not only fosters a culture of transparency and accountability but also ensures that insights are shared across departments, leading to a more cohesive and aligned business strategy.
Additionally, automated insights can help organizations stay ahead of the competition by enabling proactive decision-making based on predictive analytics. By leveraging historical data and machine learning algorithms, businesses can forecast trends, anticipate market shifts, and identify potential risks or opportunities. This proactive approach allows companies to adapt quickly to changing market conditions and maintain a competitive edge in today's dynamic business landscape.
Unleashing the Power of Natural Language in Data Analysis
Data analysis can often be a daunting task, especially for non-technical users. Natural Language Processing (NLP) offers a solution to this challenge by bridging the gap between humans and machines. With NLP, users can interact with data using everyday language, making data analysis more intuitive and accessible to a wide range of users.
NLP enables users to ask questions, extract insights, and gain a deeper understanding of their data without the need for complex coding or technical expertise. By enhancing communication with data through natural language, organizations can democratize data analysis, empowering business users to make data-driven decisions without relying solely on data scientists or analysts.
Furthermore, the applications of NLP in data analysis extend beyond just querying and extracting information. NLP algorithms can also be used for sentiment analysis, text summarization, and language translation, providing a comprehensive toolkit for exploring and understanding textual data. Sentiment analysis, for example, allows businesses to gauge public opinion and customer feedback by analyzing text data from social media, reviews, and surveys.
Additionally, NLP techniques can be integrated with machine learning models to automate tasks such as document classification, named entity recognition, and speech recognition. This integration not only streamlines data analysis processes but also enhances the accuracy and efficiency of decision-making based on textual data.
Making Machine Learning Accessible to All
Machine learning has attracted significant attention in recent years due to its ability to uncover patterns and make predictions from data. However, for many non-data scientists, machine learning can seem like a distant realm. Demystifying machine learning for beginners is crucial to unlocking its potential for all users.
Machine learning can be applied to various use cases, ranging from recommendation systems and fraud detection to predictive maintenance and demand forecasting. By understanding the basics of machine learning, even non-technical users can leverage pre-built models or low-code platforms to derive valuable insights and drive business outcomes.
One key aspect of making machine learning accessible is through education and training programs. Many organizations now offer online courses, workshops, and tutorials specifically designed for beginners to learn the fundamentals of machine learning in a structured and easy-to-understand manner. These resources often cover topics such as data preprocessing, model training, and evaluation metrics, providing a solid foundation for individuals looking to delve into the world of machine learning.
Moreover, the democratization of machine learning tools has played a significant role in lowering the barrier to entry for non-experts. User-friendly platforms and software with intuitive interfaces allow users to interact with machine learning models without requiring in-depth knowledge of programming or algorithms. This shift towards user-friendly tools empowers individuals from diverse backgrounds to explore the possibilities of machine learning and apply it to their specific domains or industries.
Maximizing Data Warehouses and Data Lakes for Analytics
Data storage plays a vital role in the success of any data analytics strategy. Two popular storage solutions are data warehouses and data lakes. Data warehouses excel at storing structured data, enabling fast query performance and facilitating business intelligence (BI) reporting. On the other hand, data lakes provide a repository for storing raw, unstructured, and semi-structured data, enabling organizations to explore and analyze diverse datasets in their original form.
Understanding the advantages of data warehouses versus data lakes is crucial for making informed decisions about data storage. While data warehouses offer structured and optimized storage for specific use cases, data lakes provide flexibility and scalability for data exploration and experimentation.
One key benefit of data warehouses is their ability to integrate data from multiple sources into a single, unified view. This consolidation of data simplifies reporting and analysis processes, making it easier for organizations to derive insights from their data. Additionally, data warehouses often come with built-in tools for data transformation and cleansing, ensuring data quality and consistency.
On the other hand, data lakes are known for their ability to store vast amounts of data in its native format, without the need for pre-defined schemas. This flexibility allows organizations to capture and store data from various sources without worrying about data structure upfront. Data lakes also support advanced analytics use cases, such as machine learning and artificial intelligence, by providing a rich source of diverse data for training and model development.
Integrating AI Analytics into Your Data Strategy
Artificial intelligence has revolutionized data analysis, empowering organizations to uncover valuable insights and patterns that were once hidden in massive datasets. By embracing AI analytics, organizations can enhance decision-making, improve operational efficiency, and gain a competitive edge in the market.
AI analytics encompasses a wide range of techniques, including machine learning, NLP, deep learning, and predictive modeling. By integrating AI analytics into your data strategy, you can unlock the full potential of your data, unveil previously unnoticed patterns, and derive actionable insights that drive business growth.
In conclusion, understanding the modern data stack is essential for organizations seeking to leverage the power of data and make informed decisions. By demystifying the components of a modern data stack, streamlining insights through automation, embracing natural language processing, unlocking the potential of machine learning, maximizing data storage solutions, and integrating AI analytics into your data strategy, you can pave the way for data-driven success. So, embark on this ultimate guide, harness the power of the modern data stack, and unlock the full potential of your organization's data.
Ready to take the next step in your data journey? CastorDoc is here to guide you through the complexities of the modern data stack with its advanced governance, cataloging, and lineage capabilities. With CastorDoc's user-friendly AI assistant, you can enable self-service analytics, making data more accessible and actionable for everyone in your organization. Whether you're a data professional looking to streamline governance and maintain compliance, or a business user seeking to harness data for strategic decisions, CastorDoc's robust platform and intuitive AI copilot will empower you to unlock the full potential of your data. Try CastorDoc today and revolutionize the way you manage and leverage your data for informed decision-making across the enterprise.
You might also like
Get in Touch to Learn More
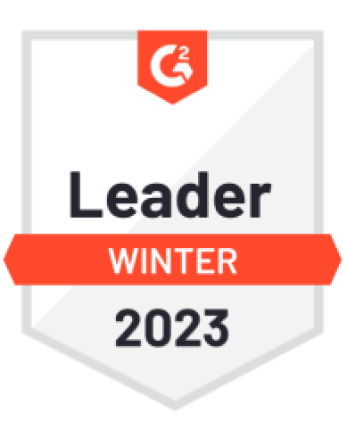
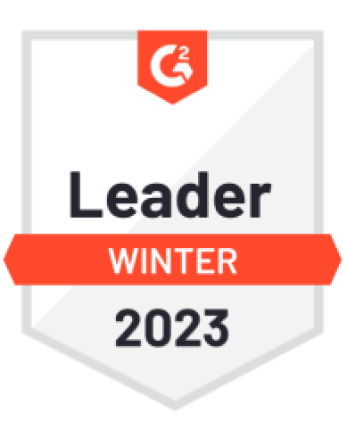

“[I like] The easy to use interface and the speed of finding the relevant assets that you're looking for in your database. I also really enjoy the score given to each table, [which] lets you prioritize the results of your queries by how often certain data is used.” - Michal P., Head of Data