5 Data Governance Standards Your Data Assets Need Now
Discover the top 5 data governance standards that are essential for safeguarding your data assets.
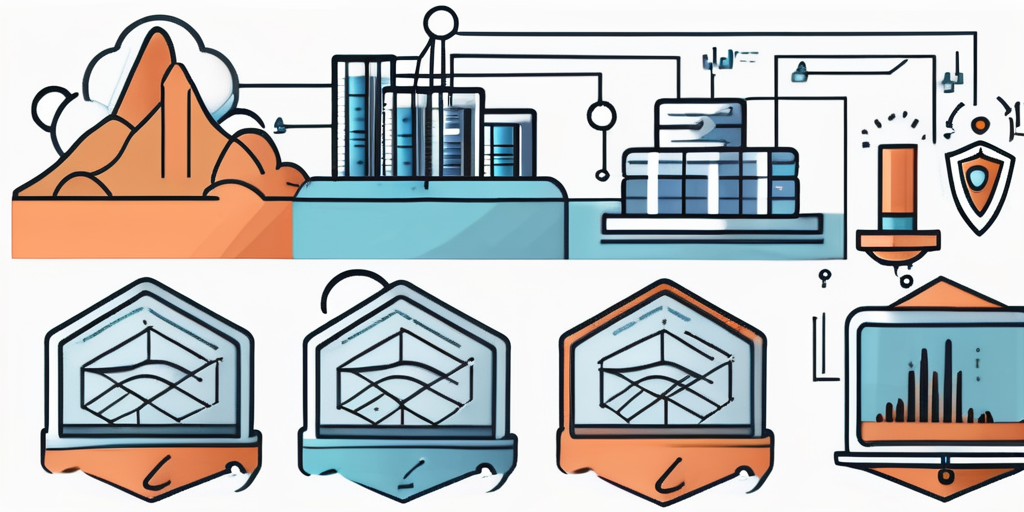
Data has become one of the most valuable assets for businesses across various industries. With the exponential growth of data, it has become crucial for organizations to implement robust data governance standards. Data governance serves as the foundation for effective management and utilization of data, ensuring its accuracy, security, and compliance with regulatory requirements. This article will delve into the importance of data governance, the essential data governance standards, the implementation process, and the methods to measure its success.
Understanding the Importance of Data Governance
Data governance plays a pivotal role in business operations and decision-making processes. By establishing data governance practices, organizations can gain a deeper understanding of their data assets and unlock their true potential. Data governance transcends beyond data management; it encompasses strategies, policies, and procedures that govern the overall lifecycle of data. With robust data governance in place, organizations can mitigate risks associated with data breaches, improve data quality, and enhance decision-making based on reliable and trusted data.
The Role of Data Governance in Business
Data governance has a profound impact on various aspects of a business. It enables organizations to establish a clear data ownership framework, ensuring accountability and responsibility for data assets. With well-defined data governance, organizations can streamline data integration, enabling seamless data flow across systems and departments. Additionally, data governance helps in aligning data initiatives with business objectives, enabling strategic decision-making based on accurate and relevant data.
Key Benefits of Implementing Data Governance Standards
Implementing data governance standards can yield significant benefits for organizations. Firstly, it enhances data quality by establishing guidelines and processes for data validation, cleansing, and enrichment. This ensures that the data used for analysis and decision-making is accurate, consistent, and reliable. Secondly, data governance standards prioritize data security and privacy, safeguarding sensitive information from unauthorized access or breaches. Thirdly, data architecture and integration standards facilitate seamless data exchange between different systems, enabling a single view of data for informed decision-making. Fourthly, metadata management standards enable efficient data discovery, understanding, and lineage tracing, ensuring transparency and accountability for data assets. Lastly, regulatory compliance standards ensure adherence to industry-specific regulations and data protection laws, mitigating legal and reputational risks faced by organizations.
Moreover, data governance also plays a crucial role in fostering data collaboration and knowledge sharing within organizations. By establishing data governance frameworks, organizations create a centralized platform for data stakeholders to collaborate, share insights, and leverage collective knowledge. This promotes a data-driven culture, where employees across departments can access and analyze data to make informed decisions and drive innovation.
Furthermore, data governance helps organizations in effectively managing data assets throughout their lifecycle. It ensures that data is properly classified, categorized, and tagged, making it easier to locate and retrieve when needed. This not only saves time and effort but also improves operational efficiency. Additionally, data governance enables organizations to establish data retention and disposal policies, ensuring compliance with legal and regulatory requirements. By properly managing data throughout its lifecycle, organizations can reduce storage costs, optimize data usage, and minimize the risk of data breaches or unauthorized access.
The Five Essential Data Governance Standards
Standard 1: Data Quality Management
Data quality management focuses on establishing processes and controls to improve and maintain the quality of data throughout its lifecycle. Organizations need to define data quality metrics and implement data cleansing and validation mechanisms to continuously monitor and enhance the quality of their data assets.
Ensuring data quality is crucial for organizations as it directly impacts the accuracy and reliability of business insights and decision-making. By implementing robust data quality management standards, organizations can minimize the risks associated with poor data quality, such as incorrect analysis, flawed reporting, and compromised customer satisfaction.
Effective data quality management involves regular data profiling and monitoring, identifying and resolving data anomalies, and implementing data governance policies to ensure data accuracy, completeness, and consistency. By investing in data quality management, organizations can enhance the trustworthiness of their data assets and drive better business outcomes.
Standard 2: Data Security and Privacy
In the era of constant data breaches and privacy concerns, data security and privacy standards are of utmost importance. Organizations should define policies and practices to protect data assets from unauthorized access, ensure data encryption during transmission and storage, and comply with privacy regulations such as GDPR and CCPA.
Data security and privacy are critical for maintaining customer trust and complying with legal requirements. Organizations must implement robust security measures, including access controls, encryption, and data classification, to safeguard sensitive information from unauthorized disclosure or misuse.
Additionally, organizations should establish privacy policies and practices that outline how personal data is collected, used, and shared. By prioritizing data security and privacy, organizations can demonstrate their commitment to protecting customer information, mitigate the risks of data breaches, and avoid potential legal and reputational consequences.
Standard 3: Data Architecture and Integration
Efficient data architecture and integration are vital for organizations to effectively leverage their data assets. Establishing standards for data integration frameworks, data modeling, and data exchange protocols ensures seamless integration of data across systems, enabling a unified view of information for analysis and decision-making.
An optimized data architecture allows organizations to efficiently store, manage, and access data, enabling faster and more accurate data processing. By defining data integration standards, organizations can ensure that data flows smoothly across different systems, eliminating data silos and enabling a holistic view of the organization's data landscape.
Furthermore, organizations should establish data modeling standards to ensure consistency and standardization in data representation. By adhering to data modeling best practices, organizations can enhance data quality, improve data interoperability, and facilitate effective data analysis and reporting.
Standard 4: Metadata Management
Metadata management focuses on capturing, organizing, and maintaining metadata, which provides contextual information about data assets. Organizations should implement metadata management standards to enable efficient data discovery, documentation, and lineage tracing, enhancing data understanding and facilitating decision-making processes.
Metadata plays a crucial role in data governance by providing valuable insights into the meaning, structure, and relationships of data. By implementing metadata management standards, organizations can improve data discoverability, understand data lineage, and ensure the accuracy and relevance of data assets.
Efficient metadata management involves capturing and documenting metadata attributes such as data source, data type, data owner, and data usage. By maintaining a comprehensive metadata repository, organizations can enhance collaboration, enable effective data governance, and empower data consumers to make informed decisions based on accurate and reliable data.
Standard 5: Regulatory Compliance
To mitigate legal and reputational risks, organizations must adhere to industry-specific regulations and data protection laws. Establishing standards for regulatory compliance ensures that data governance practices align with applicable regulations, enabling organizations to avoid penalties, maintain customer trust, and safeguard their reputation.
Compliance with regulatory requirements is essential for organizations operating in various industries, such as healthcare, finance, and telecommunications. By implementing robust regulatory compliance standards, organizations can ensure that their data governance practices align with legal obligations, industry standards, and customer expectations.
Organizations should establish data governance policies and procedures that address specific regulatory requirements, such as data retention, data access, and data sharing. By proactively monitoring and adapting to regulatory changes, organizations can maintain compliance, mitigate risks, and build a strong foundation for ethical and responsible data management.
Implementing Data Governance Standards
Steps to Adopt Data Governance Standards
Adopting data governance standards is a comprehensive process that requires careful planning and execution. Organizations should start by assessing their current data governance maturity level and identifying gaps and areas of improvement. Next, they should establish a cross-functional data governance team and define their data governance framework, including policies, roles, responsibilities, and processes. Implementing the chosen data governance standards should be done incrementally, aligning with the organization's objectives and priorities. Communication and training programs should also be conducted to facilitate a smooth transition and ensure buy-in from stakeholders at all levels.
Overcoming Challenges in Implementation
Implementing data governance standards can pose various challenges for organizations. Resistance to change, lack of awareness about the benefits of data governance, and insufficient resources are some common hurdles. To overcome these challenges, organizations should emphasize the value proposition of data governance, demonstrate its impact on business outcomes, and secure executive sponsorship. Additionally, establishing a culture of data governance and promoting collaboration across departments can help in overcoming resistance and fostering a data-driven mindset throughout the organization.
Measuring the Success of Data Governance Standards
Key Performance Indicators for Data Governance
Measuring the success of data governance standards requires the establishment of key performance indicators (KPIs). These KPIs should align with the goals and objectives of the organization. Common KPIs include data quality metrics, data accessibility and availability, data security incidents, and compliance with regulatory requirements. Regular monitoring and reporting of these KPIs enable organizations to track the effectiveness of their data governance initiatives and identify areas for improvement.
Continuous Improvement and Adaptation in Data Governance
Data governance is an ongoing process that requires continuous improvement and adaptation to evolving business needs and technological advancements. Organizations should regularly assess the effectiveness of their data governance standards and practices, solicit feedback from stakeholders, and incorporate lessons learned into their data governance framework. By adopting a continuous improvement mindset, organizations can ensure that their data assets are governed effectively, enabling them to derive maximum value and stay competitive in today's data-driven business landscape.
You might also like
Get in Touch to Learn More
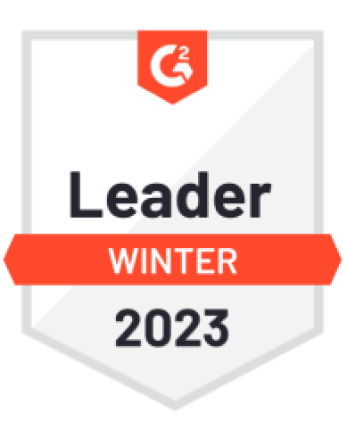
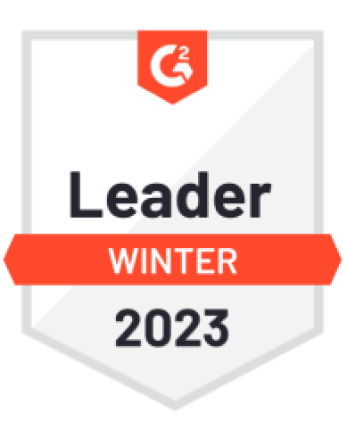

“[I like] The easy to use interface and the speed of finding the relevant assets that you're looking for in your database. I also really enjoy the score given to each table, [which] lets you prioritize the results of your queries by how often certain data is used.” - Michal P., Head of Data