9 Components to Build the Best Data Quality Framework
Discover the essential components to create a robust and effective data quality framework.
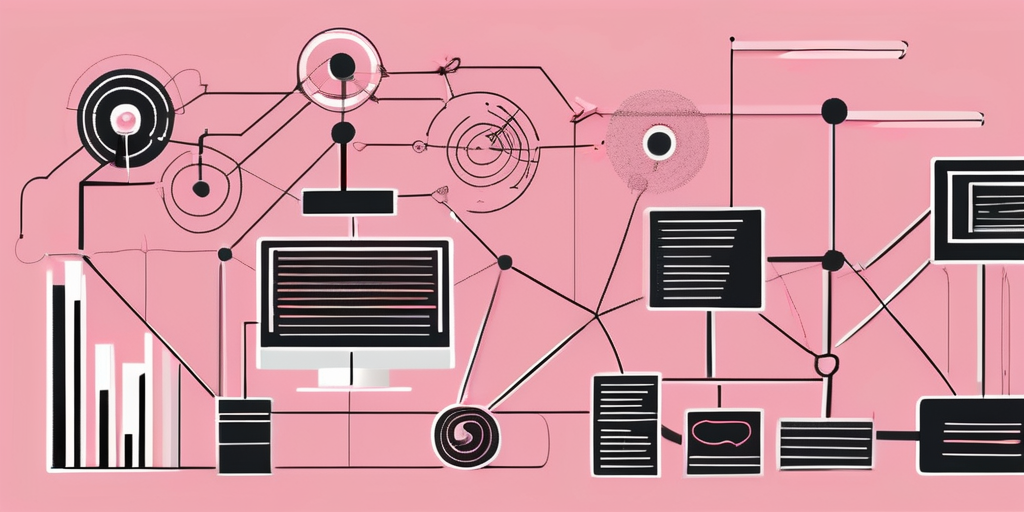
Data Quality is paramount for the success of any business. A strong data quality framework ensures that accurate, reliable, and consistent data is readily available for analysis and decision-making. This article outlines the nine essential components that will help you build the best data quality framework for your organization.
Understanding the Importance of a Data Quality Framework
Data quality is not just a matter of cleanliness and integrity; it goes beyond that. A data quality framework provides a structured approach to manage data throughout its lifecycle, from creation to retirement. By establishing a framework, organizations can ensure that data is fit for purpose and supports their business objectives.
Implementing a data quality framework involves defining data quality standards, establishing processes for data collection and validation, and implementing tools for monitoring and improving data quality over time. This systematic approach helps organizations maintain high-quality data that is reliable, accurate, and consistent.
The Role of Data Quality in Business Success
Data quality directly impacts business success in numerous ways. Firstly, accurate and reliable data enables better decision-making, leading to more informed strategies and improved operational efficiency. Secondly, it enhances customer satisfaction by ensuring that interactions are based on accurate information. Lastly, it ensures compliance with regulatory requirements and minimizes the risk of financial or reputational damage resulting from data inaccuracies.
Furthermore, high-quality data can drive innovation and facilitate the development of new products and services. By leveraging accurate data insights, organizations can identify market trends, customer preferences, and areas for growth, giving them a competitive edge in the industry.
The Impact of Poor Data Quality
Poor data quality can have severe consequences for businesses. Inaccurate data can lead to wrong decisions, inefficient processes, and dissatisfied customers. It can also result in missed opportunities, increased costs, and compromised business performance. Recognizing the negative impact of poor data quality is crucial in encouraging organizations to prioritize the development of a sound data quality framework.
Moreover, poor data quality can erode trust in an organization's brand and credibility. Customers, partners, and stakeholders rely on accurate data to make informed decisions and build long-term relationships. When data quality is compromised, it can damage the reputation of the organization and lead to a loss of trust among key stakeholders.
The Essential Components of a Data Quality Framework
Component 1: Data Governance
Data governance sets the foundation for a data quality framework. It defines the policies, procedures, and responsibilities for managing and ensuring data quality. This component includes establishing data ownership, defining data standards, and implementing data stewardship roles to enforce data quality guidelines.
Effective data governance is like the backbone of an organization's data quality framework. It provides the necessary structure and framework to ensure that data is managed and maintained in a consistent and reliable manner. With clear data ownership, organizations can assign accountability and responsibility for data quality, ensuring that everyone understands their role in maintaining high-quality data.
Data governance also involves defining data standards, which serve as a set of guidelines and best practices for data management. These standards help organizations establish a common understanding of data quality expectations and ensure that data is collected, stored, and used consistently across different systems and processes.
Component 2: Data Profiling
Data profiling involves analyzing and assessing the quality of data to identify anomalies, inconsistencies, and inaccuracies. It helps organizations understand their data, uncover potential issues, and prioritize data quality improvement efforts. Effective data profiling involves examining data completeness, accuracy, consistency, and uniqueness.
When it comes to data profiling, organizations need to dive deep into their data to uncover hidden insights and potential problems. By examining data completeness, organizations can identify missing or incomplete data that may impact the accuracy of their analyses. Accuracy assessment helps to uncover data that may contain errors or inconsistencies, allowing organizations to take corrective actions and improve data quality.
Consistency analysis is another crucial aspect of data profiling. It helps organizations identify data that may have different formats or values across different systems or databases. By ensuring consistency, organizations can avoid confusion and ensure that data is interpreted correctly. Lastly, uniqueness assessment helps organizations identify duplicate or redundant data, which can lead to inaccuracies and inefficiencies in data analysis and decision-making processes.
Component 3: Data Cleansing
Data cleansing aims to correct or remove errors, inconsistencies, and redundancies within datasets. This component involves techniques such as deduplication, standardization, normalization, and validation. By cleansing data, organizations ensure that it is accurate, consistent, and reliable for analysis.
Data cleansing is like giving your data a makeover. It involves various techniques to improve data quality and ensure that it is fit for purpose. Deduplication helps organizations identify and remove duplicate records, reducing data redundancy and improving data accuracy. Standardization involves transforming data into a consistent format, making it easier to compare and analyze. Normalization ensures that data is organized in a structured manner, eliminating inconsistencies and improving data integrity.
Validation is another critical aspect of data cleansing. It involves applying predefined rules and checks to ensure that data meets specific criteria or requirements. By validating data, organizations can identify and correct errors or inconsistencies, ensuring that only accurate and reliable data is used for analysis and decision-making.
Component 4: Data Integration
Data integration involves combining data from various sources and formats to create a unified and consistent view. This component ensures that data is integrated seamlessly, eliminating data silos and improving data accessibility. By integrating data, organizations can unlock its full potential for analysis and decision-making.
Data integration is like connecting the dots. It brings together data from different sources, such as databases, applications, or external systems, to provide a holistic view of information. By integrating data, organizations can break down data silos and enable cross-functional analysis, leading to better insights and more informed decision-making.
Effective data integration involves mapping and transforming data from different sources into a common format or structure. This process ensures that data is consistent and compatible, enabling organizations to combine and analyze information seamlessly. By integrating data, organizations can also improve data accessibility, making it easier for users to find and retrieve the information they need.
Component 5: Data Validation
Data validation verifies the accuracy, completeness, and integrity of data through various validation checks and rules. It ensures that data conforms to predefined standards and meets business requirements. Data validation is crucial for preventing the ingestion of incorrect or unreliable data into systems.
Data validation is like having a quality control checkpoint for your data. It involves applying validation checks and rules to ensure that data meets specific criteria or requirements. This process helps organizations identify and flag data that may be inaccurate, incomplete, or inconsistent, preventing the ingestion of unreliable data into systems.
Effective data validation involves defining and implementing validation rules that align with business requirements and data quality standards. These rules can range from simple checks, such as data type validation, to more complex validations, such as cross-field or cross-record validations. By validating data, organizations can ensure that it is reliable and trustworthy, enabling users to make informed decisions based on accurate information.
Component 6: Data Monitoring
Data monitoring involves continuous monitoring and tracking of data quality metrics and key performance indicators (KPIs). It enables organizations to identify deviations from established data quality standards and take corrective actions promptly. Effective data monitoring ensures that data quality is maintained over time.
Data monitoring is like having a watchful eye over your data. It involves regularly tracking and analyzing data quality metrics and KPIs to ensure that data meets predefined standards and expectations. By monitoring data, organizations can identify any deviations or anomalies that may indicate a decline in data quality and take corrective actions promptly.
Effective data monitoring involves establishing data quality metrics and KPIs that align with business requirements and data quality objectives. These metrics can include data completeness, accuracy, consistency, timeliness, and relevancy. By continuously monitoring data quality, organizations can ensure that data remains reliable and up-to-date, enabling users to make well-informed decisions.
Component 7: Data Enrichment
Data enrichment enhances the value of data by supplementing it with additional attributes or information. This component involves enriching data through external sources, machine learning algorithms, or manual processes. By enriching data, organizations gain deeper insights and improve the accuracy and relevance of their analyses.
Data enrichment is like adding extra layers of information to your data. It involves enhancing existing data by incorporating additional attributes or information from external sources or through advanced analytical techniques. By enriching data, organizations can gain a more comprehensive understanding of their data and uncover hidden patterns or relationships.
Data enrichment can be achieved through various methods, such as data augmentation using external data sources, sentiment analysis, or predictive modeling. By leveraging these techniques, organizations can enhance the accuracy and relevance of their analyses, leading to more meaningful insights and better decision-making.
Component 8: Metadata Management
Metadata management involves capturing, organizing, and maintaining metadata, which provides context and meaning to data. This component includes defining data dictionaries, data lineage, and data classification. Effective metadata management ensures that data is well-understood and correctly interpreted.
Metadata management is like creating a roadmap for your data. It involves capturing and organizing metadata, which provides essential information about data, such as its source, structure, and meaning. By managing metadata effectively, organizations can ensure that data is well-documented and correctly interpreted, enabling users to understand and utilize data accurately.
Effective metadata management includes defining data dictionaries, which serve as a centralized repository of data definitions and descriptions. It also involves establishing data lineage, which tracks the origin and transformation of data, ensuring data traceability and accountability. Data classification is another critical aspect of metadata management, as it helps organizations categorize and organize data based on its sensitivity, usage, or other relevant attributes.
Component 9: Master Data Management
Master Data Management (MDM) focuses on managing critical business data, such as customer or product information, to ensure its consistency across systems and applications. This component involves establishing data governance practices, data quality rules, and data stewardship for master data. MDM enables organizations to have a single, accurate version of master data across their entire ecosystem.
Master Data Management is like having a single source of truth for your most important data. It involves establishing data governance practices and data quality rules specifically for critical business data, such as customer or product information. By implementing MDM, organizations can ensure that master data is consistent, accurate, and reliable across different systems and applications.
Effective MDM involves defining data governance practices that align with business requirements and data quality objectives. It also requires establishing data quality rules and validation checks to ensure the integrity and reliability of master data. Data stewardship plays a crucial role in MDM, as it involves assigning responsibility and accountability for the maintenance and quality of master data.
Implementing Your Data Quality Framework
Steps to Implement a Successful Data Quality Framework
Implementing a data quality framework involves a systematic approach. Begin by conducting a data quality assessment to understand the current state and areas of improvement. Develop a comprehensive data quality strategy, outlining the goals, objectives, and milestones. Identify the appropriate tools and technologies required for each component and establish data quality metrics to measure progress and success. Finally, implement the framework in stages, focusing on continuous improvement and ongoing data quality management.
Overcoming Challenges in Data Quality Framework Implementation
Implementing a data quality framework can present various challenges. These may include organizational resistance to change, lack of executive buy-in, limited budget and resources, and complexity in dealing with diverse data sources. To overcome these challenges, it is crucial to emphasize the business value of data quality, engage stakeholders at all levels, secure management support, and establish a structured approach with clear accountability. Additionally, leveraging data quality tools and technologies can streamline implementation and help address specific challenges.
In conclusion, a robust data quality framework is essential for organizations to ensure that their data is accurate, reliable, and aligned with the business objectives. By implementing the nine components outlined in this article, organizations can build a comprehensive data quality framework that drives informed decision-making, improves operational efficiency, and enhances overall business success. Remember, data quality is not a one-time effort but an ongoing process that requires commitment, dedication, and continuous improvement.
You might also like
Get in Touch to Learn More
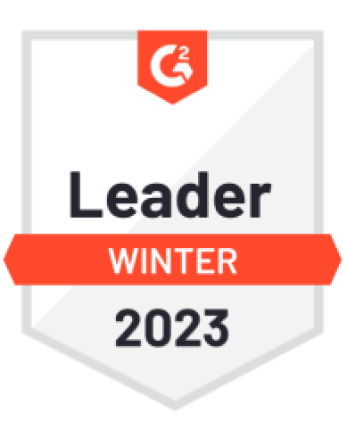
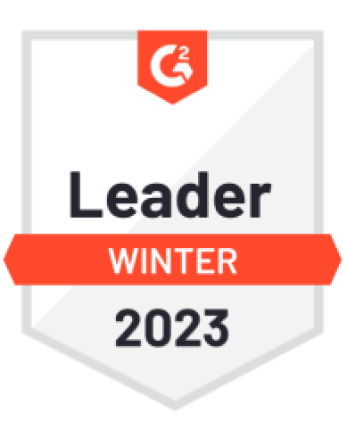

“[I like] The easy to use interface and the speed of finding the relevant assets that you're looking for in your database. I also really enjoy the score given to each table, [which] lets you prioritize the results of your queries by how often certain data is used.” - Michal P., Head of Data