Column-Level Lineage: What It Is and How To Use It
Uncover the power of column-level lineage in your data management strategy with this guide
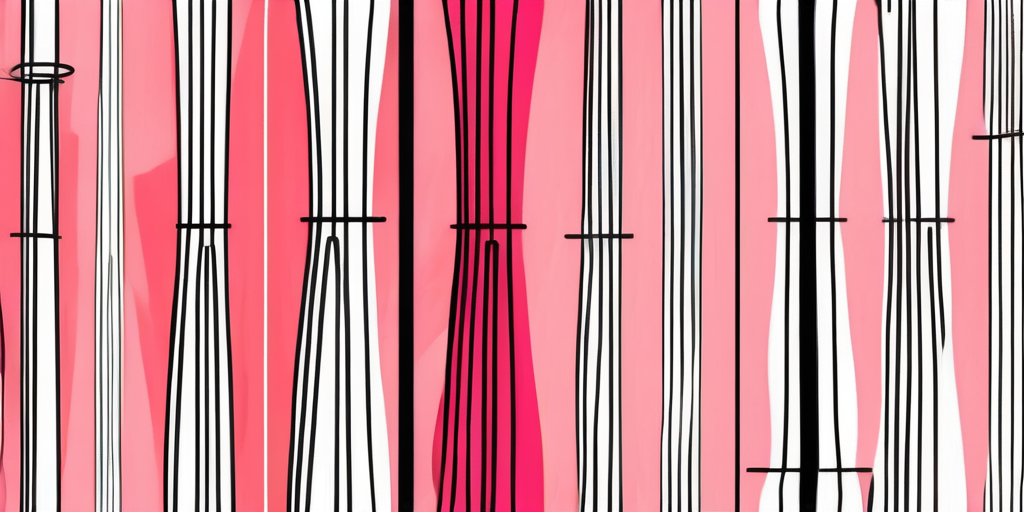
In the realm of data management and governance, column-level lineage has emerged as a vital concept. It provides a comprehensive understanding of the flow of data within an organization, from its sources to its targets. This article will delve into the intricacies of column-level lineage, explaining its importance and exploring its various components. Additionally, we will discuss the process of implementing column-level lineage and the role it plays in data governance. Finally, we will provide tips on optimizing the use of column-level lineage and discuss its future prospects.
Understanding the Concept of Column-Level Lineage
Before delving into the specifics, let's first grasp the basics of column-level lineage. At its core, column-level lineage refers to the documentation of how data flows from one column to another within a system. It provides a granular view of data transformations, allowing organizations to trace the origins and transformations of each data element.
Column-level lineage is not just a static representation of data relationships; it is a dynamic map that evolves as data moves through various processes and systems. This dynamic nature enables organizations to adapt to changes in data sources, transformations, and consumption patterns, ensuring that data lineage remains accurate and up to date.
The Basics of Column-Level Lineage
Column-level lineage can be seen as the "family tree" of data. It outlines the relationships between columns in a database or data warehouse, enabling stakeholders to navigate the complex intertwining of data flows. By visualizing the connections, businesses gain insights into how data is consumed and aggregated, facilitating streamlined decision-making.
When examining column-level lineage, it's essential to consider not only the direct relationships between columns but also the indirect dependencies that may exist. These indirect dependencies can reveal hidden connections that impact data quality and integrity, providing a more comprehensive understanding of the data ecosystem.
The Importance of Column-Level Lineage in Data Management
Clear and accurate column-level lineage is crucial for effective data management. It provides a solid foundation for data governance initiatives, ensuring compliance, data quality, and regulatory requirements are met. Additionally, column-level lineage promotes data transparency, making it easier to identify and rectify anomalies or errors within the data pipeline.
By establishing robust column-level lineage practices, organizations can enhance data trustworthiness and facilitate collaboration among data users. This transparency fosters a data-driven culture where stakeholders can confidently make decisions based on a clear understanding of data origins and transformations.
The Components of Column-Level Lineage
Column-level lineage comprises two fundamental components: data sources and data targets. Understanding these components is essential to comprehending the full scope of column-level lineage.
When delving into column-level lineage, it's crucial to recognize the intricate web of connections that exist between data sources and targets. This interconnectedness forms the backbone of data lineage, providing a detailed roadmap of how data moves through an organization's ecosystem.
Data Sources in Column-Level Lineage
Data sources refer to the origins of the data. These can range from internal databases and applications to external data feeds and APIs. By mapping data sources, organizations gain insights into the credibility and reliability of the information they receive, enabling them to make informed decisions.
Exploring data sources further unveils the complexity of data acquisition processes within an organization. It involves understanding not just where the data comes from, but also how it is collected, processed, and transformed along its journey. This level of granularity is essential for ensuring data quality and regulatory compliance.
Data Targets in Column-Level Lineage
Data targets represent the destinations of the data as it flows through the system. This could include data marts, data warehouses, or analytical platforms. Knowing where data ends up is critical for ensuring data accuracy and integrity, allowing organizations to trust their data-driven insights.
Understanding data targets goes beyond just identifying storage locations; it involves comprehending how data is utilized and leveraged to drive business decisions. By tracing data to its ultimate destination, organizations can optimize their data infrastructure and ensure that the right information reaches the right stakeholders at the right time.
The Process of Implementing Column-Level Lineage
Implementing column-level lineage requires a structured approach. Let's break down the steps involved in establishing this invaluable data management practice.
Column-level lineage is a critical aspect of data governance that provides organizations with a clear understanding of how data moves through their systems. By tracing the lineage of individual columns, organizations can ensure data quality, compliance, and decision-making based on accurate information.
Steps to Establish Column-Level Lineage
1. Identify and inventory all data sources and targets within the organization.2. Map the relationships between columns, capturing the flow of data from source to target.3. Document and maintain an up-to-date lineage repository, ensuring it is easily accessible to relevant stakeholders.4. Regularly validate and verify the accuracy of the lineage information to ensure its reliability.5. Establish processes and governance policies to enforce the consistent documentation of column-level lineage across the organization.
Creating a comprehensive column-level lineage involves collaboration between data architects, data engineers, business analysts, and other stakeholders. Each team plays a crucial role in ensuring that the lineage information is accurate, complete, and aligned with the organization's data strategy.
Challenges in Implementing Column-Level Lineage
Although column-level lineage offers immense benefits, organizations often face challenges in its implementation. These can include the complexity of data flows, inadequately documented systems, and the need for collaboration across multiple teams. Overcoming these hurdles requires a proactive and dedicated approach, involving stakeholders from various disciplines to ensure comprehensive coverage and accuracy.
Effective communication and transparency are key to successfully implementing column-level lineage. Organizations must prioritize education and training to empower their teams with the knowledge and skills needed to maintain and leverage column-level lineage effectively.
The Role of Column-Level Lineage in Data Governance
Data governance encompasses the processes, policies, and standards that ensure the effective management of data resources. The implementation of column-level lineage plays a crucial role in supporting data governance efforts.
When delving into the realm of data governance, it is essential to recognize the intricate web of relationships that exist within datasets. Column-level lineage offers a granular view of how data moves through various systems and processes, shedding light on dependencies and interactions that might otherwise go unnoticed. This level of detail empowers organizations to make informed decisions regarding data management strategies and resource allocation.
Enhancing Data Quality with Column-Level Lineage
Column-level lineage enables organizations to maintain data accuracy and quality. By understanding the flow of data, stakeholders can identify potential bottlenecks, data integrity issues, or inconsistencies. This insight allows organizations to implement data quality measures effectively and take corrective actions when necessary.
Moreover, the utilization of column-level lineage opens up opportunities for proactive data quality management. By proactively monitoring data lineage, organizations can anticipate and address issues before they escalate, thus fostering a culture of continuous improvement and data excellence.
Column-Level Lineage in Compliance and Auditing
Complying with regulatory requirements and undergoing audits is a key aspect of data governance. With column-level lineage, organizations can easily trace the path of data, aiding in compliance efforts. Additionally, it provides auditors with a clear understanding of data transformations, ensuring transparency, and facilitating smooth audits.
Furthermore, the detailed insights provided by column-level lineage serve as a valuable resource during regulatory audits. Auditors can leverage this information to verify data accuracy, assess compliance with data handling regulations, and gain a comprehensive understanding of how data is utilized across different functions within an organization.
Optimizing the Use of Column-Level Lineage
To leverage the full potential of column-level lineage, organizations should adhere to some best practices.
Column-level lineage is a crucial aspect of data management that provides insights into the flow of data from its source to its destination. By understanding the lineage of each column in a dataset, organizations can ensure data quality, traceability, and compliance with regulations. This level of granularity allows for better decision-making processes and enhances overall data governance.
Best Practices for Using Column-Level Lineage
- Maintain comprehensive documentation of data sources and targets.
- Regularly validate and update lineage information.
- Establish data governance policies and procedures to enforce consistent lineage documentation.
- Train and educate relevant stakeholders on the importance and usage of column-level lineage.
- Integrate column-level lineage into the broader data management strategy.
Implementing these best practices ensures that organizations can fully harness the power of column-level lineage and derive maximum value from their data assets.
The Future of Column-Level Lineage
As organizations continue to grapple with increasing data complexity, column-level lineage will become more critical than ever. Advancements in technology, such as automated lineage generation and integration with data governance platforms, will drive the adoption of column-level lineage across industries. By embracing this practice, organizations can unlock the true potential of their data assets and stay ahead in the ever-evolving data landscape.
In addition to technological advancements, regulatory requirements around data privacy and security are also driving the importance of column-level lineage. Organizations are under increasing pressure to demonstrate data lineage for compliance purposes, making it essential to have robust lineage tracking mechanisms in place. The ability to trace the origins of data fields and understand their transformations is key to meeting regulatory standards and building trust with stakeholders.
You might also like
Get in Touch to Learn More
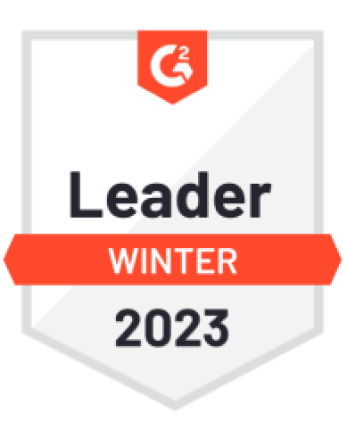
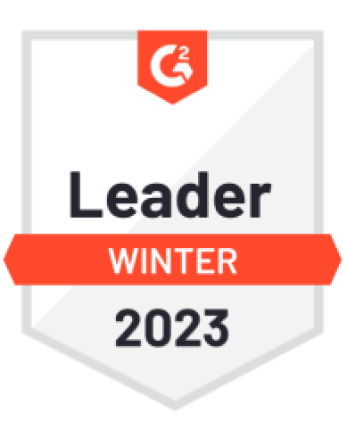

“[I like] The easy to use interface and the speed of finding the relevant assets that you're looking for in your database. I also really enjoy the score given to each table, [which] lets you prioritize the results of your queries by how often certain data is used.” - Michal P., Head of Data