Data Fabric vs Data Lake: What's Right for You and When?
Discover the differences between data fabric and data lake, and learn which one is the right choice for your organization.

Businesses are faced with the challenge of managing vast amounts of information and extracting valuable insights from it. Two popular approaches to data management are data fabric and data lake. While they share similarities in terms of storing and organizing data, they have distinct characteristics that make them suitable for different scenarios. In this article, we will explore the basics of data fabric and data lake, discuss their key differences, analyze the pros and cons of each, and provide guidance on determining which approach is right for your business.
Understanding the Basics: Data Fabric and Data Lake
Defining Data Fabric
Data fabric is an architecture that enables seamless data integration and access across different systems and locations. It provides a unified view of data spread across various sources, whether they are on-premises or in the cloud. Data fabric leverages technologies such as data virtualization, data integration, and data governance to create a consistent and easily accessible data layer.
One of the key advantages of implementing a data fabric architecture is its ability to adapt to the evolving data landscape. As organizations deal with an increasing volume and variety of data, data fabric ensures that data can be accessed and utilized efficiently, regardless of its source or format. By incorporating data virtualization techniques, data fabric allows users to query and retrieve data from disparate sources as if it were all stored in a single location, reducing the complexities associated with data silos.
Defining Data Lake
Data lake, on the other hand, is a storage repository that holds a vast amount of raw and unprocessed data in its native format. It serves as a centralized location for collecting data from various sources without the need for predefined schemas or data transformations. Data lake allows for data exploration, experimentation, and iterative analysis without the constraints of traditional data warehouses.
Within a data lake environment, organizations can store structured, semi-structured, and unstructured data in its raw form, preserving the data's original fidelity. This raw data can then be used for a variety of purposes, including advanced analytics, machine learning, and real-time processing. Data lakes are designed to scale horizontally, enabling organizations to store petabytes of data cost-effectively while maintaining flexibility in data processing and analysis.
Key Differences Between Data Fabric and Data Lake
Data Structure and Management
Data fabric imposes structure and governance on data, ensuring its consistency and quality. It provides a semantic layer that allows users to query and analyze data using standardized business terms. This structured approach not only enhances data organization but also facilitates collaboration and data sharing across different teams within an organization. By enforcing a specific structure, data fabric enables data scientists, analysts, and business users to easily understand and interpret the data, leading to more accurate insights and informed decision-making.
In contrast, data lake does not enforce any specific structure. It accepts any type of data, including structured, semi-structured, and unstructured formats. This flexibility allows for the storage of diverse data types, such as text, images, videos, and sensor data. However, the lack of structure in a data lake can pose challenges when querying or analyzing the data. Without predefined schemas or standardized business terms, users may encounter difficulties in extracting meaningful information from the data lake, leading to potential delays in data analysis and decision-making processes.
Scalability and Flexibility
Data fabric is designed to be scalable and flexible, allowing businesses to adapt to changing data requirements. It can seamlessly handle growing data volumes and accommodate new data sources as needed. With its distributed architecture, data fabric can scale horizontally by adding more nodes to the cluster, ensuring high availability and performance even with increasing data loads. This scalability empowers organizations to efficiently manage their data growth and leverage emerging technologies, such as Internet of Things (IoT) devices and edge computing, without compromising on data accessibility and performance.
On the other hand, data lake offers unparalleled scalability, thanks to its distributed storage architecture. It can scale horizontally by adding more servers to the cluster, providing virtually unlimited storage capacity for massive amounts of data. This scalability makes data lake an ideal choice for organizations dealing with exponential data growth or those that require long-term data retention. With its ability to handle petabytes or even exabytes of data, data lake can support the storage and analysis of large-scale datasets, enabling advanced analytics and machine learning applications.
Data Integration and Accessibility
Data fabric excels in data integration and accessibility. It can connect to various data sources, whether they are traditional databases, cloud services, or file systems. By leveraging connectors and APIs, data fabric enables seamless data integration and real-time data access, eliminating the need for data movement or replication. This real-time accessibility empowers users to make data-driven decisions based on the most up-to-date information available, enhancing business agility and responsiveness.
In contrast, data lake focuses more on data storage than integration. It enables data ingestion from multiple sources, including structured and unstructured data, but may require additional processing and transformation before it becomes easily accessible to users. Data lake often serves as a central repository for raw data, acting as a staging area for data scientists and analysts to perform data cleansing, transformation, and modeling. While this approach allows for greater flexibility in data exploration and experimentation, it may introduce additional complexities and delays in the data analysis workflow.
Pros and Cons of Data Fabric
Advantages of Data Fabric
Data fabric provides a unified and consistent view of data, making it easier for users to access and analyze information across different systems. It enables agile data integration, allowing businesses to respond quickly to changing data requirements. With data fabric, organizations can implement data governance and security policies more effectively, ensuring data quality and compliance.
One key advantage of data fabric is its ability to streamline data management processes by reducing data silos within an organization. By breaking down these silos, data fabric facilitates a more holistic view of data, enabling better decision-making and more efficient operations. Additionally, data fabric can enhance data accessibility and usability, empowering users to derive valuable insights from a wide range of data sources.
Disadvantages of Data Fabric
Implementing data fabric requires careful planning and coordination across multiple systems and stakeholders. It may involve significant upfront investment in infrastructure and technologies. Data fabric can also introduce additional complexity, particularly when dealing with heterogeneous data sources and complex integration scenarios.
Another challenge associated with data fabric is the potential for data redundancy and inconsistency if not implemented properly. Managing data across various systems and ensuring synchronization can be a daunting task, requiring continuous monitoring and maintenance. Moreover, the scalability of data fabric solutions can pose challenges as data volumes grow, necessitating ongoing optimization and resource allocation.
Pros and Cons of Data Lake
Advantages of Data Lake
Data lake offers unparalleled scalability and flexibility. It can accommodate various data formats, making it suitable for storing diverse types of data, including structured, semi-structured, and unstructured data. With data lake, businesses can capture and store data rapidly without the need for extensive data modeling or ETL processes. It enables data exploration and supports data science initiatives, facilitating advanced analytics and machine learning.
Furthermore, data lakes provide a cost-effective solution for storing massive amounts of data. By leveraging technologies like Hadoop and cloud storage, organizations can scale their data lakes according to their needs without incurring exorbitant costs. This scalability ensures that businesses can adapt to changing data requirements and storage demands without significant financial investments.
Disadvantages of Data Lake
Data lake may suffer from data quality issues, as it accepts raw and unprocessed data without enforcing any predefined schema or validation rules. It can become a data dumping ground if not properly managed, leading to data sprawl and increased complexity. Without proper governance and data cataloging, finding and understanding relevant data within the lake can be challenging.
Moreover, another drawback of data lakes is the potential for data security and privacy concerns. Since data lakes store vast amounts of data from various sources, ensuring compliance with data protection regulations and safeguarding sensitive information becomes increasingly complex. Without robust security measures and access controls in place, data lakes can be vulnerable to breaches and unauthorized access, putting sensitive data at risk.
Determining What's Right for Your Business
Assessing Your Business Needs
When deciding between data fabric and data lake, it is crucial to understand your business requirements and data management goals. Evaluate factors such as the types and sources of data you deal with, the need for data integration and accessibility, and the level of data governance and security required.
Evaluating Your Data Volume and Variety
If your organization deals with vast volumes of data from diverse sources and requires scalability and flexibility, data lake may be a favorable choice. However, if your focus is on data integration, consistency, and real-time access, data fabric may better suit your needs.
Considering Your Future Growth
Consider your organization's growth plans and future data requirements. If you anticipate significant data growth or the need to incorporate new data sources, a scalable solution like data lake might be more suitable. However, if you prioritize data governance, standardization, and integration with existing systems, data fabric may be the better option.
Ultimately, the decision between data fabric and data lake depends on your unique business needs and priorities. In some cases, a combination of both approaches may provide the optimal solution. By carefully assessing your requirements, evaluating the pros and cons, and considering your future growth, you can determine the right data management strategy for your business.
You might also like
Get in Touch to Learn More
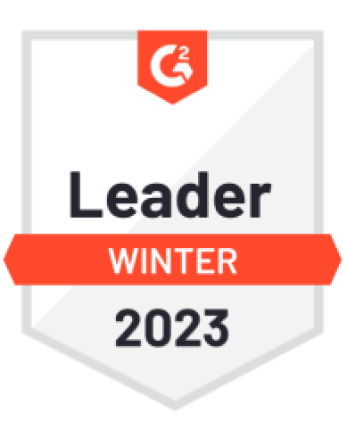
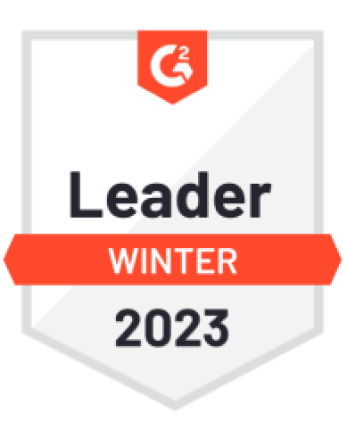

“[I like] The easy to use interface and the speed of finding the relevant assets that you're looking for in your database. I also really enjoy the score given to each table, [which] lets you prioritize the results of your queries by how often certain data is used.” - Michal P., Head of Data