Data Governance vs Data Analytics: Which is Best for You?
Discover the key differences between data governance and data analytics to determine which is the best fit for your organizational needs.

Data governance and data analytics are two critical aspects of managing and deriving insights from data in today's business landscape. Understanding the differences between data governance and data analytics is essential for organizations to make informed decisions about which approach is best suited for their specific needs. This article aims to explore the basics of data governance and data analytics, highlight their key differences, discuss their respective roles in business, and examine their impact on business performance. Finally, it will provide guidance on how to choose between data governance and data analytics based on business needs, available resources, and long-term goals.
Understanding the Basics
What is Data Governance?
Data governance refers to the overall management of data availability, usability, integrity, and security within an organization. It involves the establishment of policies, procedures, and controls to ensure that data is captured, stored, and used in a standardized and compliant manner. The primary goal of data governance is to ensure the accuracy, consistency, and reliability of data across the entire organization.
Effective data governance requires a clear understanding of the organization's data assets, including the identification of data owners, data stewards, and data custodians. Data owners are responsible for the strategic direction and decision-making related to data, while data stewards ensure the quality, integrity, and proper use of data. Data custodians, on the other hand, are responsible for the technical aspects of data management, such as data storage and security.
In addition to defining roles and responsibilities, data governance involves defining data governance frameworks, implementing data quality measures, and establishing data governance committees to oversee data-related decisions and activities. These committees, composed of representatives from different departments, work together to develop and enforce data governance policies, resolve data-related issues, and drive continuous improvement in data management practices.
What is Data Analytics?
Data analytics refers to the process of analyzing and interpreting raw data to gain insights, identify patterns, and make informed business decisions. It involves using statistical techniques, machine learning algorithms, and data visualization tools to extract meaningful information from vast amounts of structured and unstructured data.
Data analytics encompasses various approaches, including descriptive analytics, which provides a summary of historical data; diagnostic analytics, which helps identify the causes of past events; predictive analytics, which uses historical data to predict future outcomes; and prescriptive analytics, which suggests optimal actions based on the predicted outcomes.
Within the field of data analytics, there are different types of analytics techniques that organizations can employ. These include exploratory data analysis, which involves searching for patterns and relationships in data; regression analysis, which helps understand the relationship between variables; and clustering analysis, which groups similar data points together based on their characteristics.
Furthermore, data analytics is not limited to just structured data. It also encompasses unstructured data, such as text documents, images, and videos. By leveraging natural language processing, image recognition, and other advanced techniques, organizations can extract valuable insights from unstructured data sources, enabling them to make data-driven decisions across various domains, including marketing, finance, operations, and customer service.
Key Differences Between Data Governance and Data Analytics
Purpose and Function
Data governance focuses on establishing processes and controls to ensure the quality, integrity, and security of data. Its primary purpose is to enable better decision-making by providing trusted and reliable data. On the other hand, data analytics is concerned with extracting insights and patterns from data to support informed decision-making and strategic planning.
Data governance is more focused on data management and establishing guidelines, while data analytics leverages data to derive actionable insights and drive business performance. Data governance sets the stage for effective data analytics by ensuring data quality and integrity throughout the analytics process.
For example, in the healthcare industry, data governance plays a crucial role in ensuring patient data privacy and security. It establishes protocols and standards for data handling, ensuring that sensitive information is protected and only accessed by authorized personnel. On the other hand, data analytics in healthcare can be used to identify patterns in patient data that can help in early disease detection or improve treatment outcomes.
Required Skills and Expertise
Implementing and managing data governance requires individuals with a thorough understanding of data management practices, data quality principles, and regulatory compliance requirements. Data governance professionals should possess strong organizational, communication, and analytical skills to effectively coordinate data-related activities and enforce data governance policies.
Data analytics, on the other hand, requires individuals with expertise in data analysis, statistical modeling, programming, and data visualization. These professionals must be proficient in using various analytics tools and techniques to extract insights from data and communicate them effectively to stakeholders.
Moreover, data governance professionals often collaborate with legal and compliance teams to ensure that data governance policies align with industry regulations and standards. They work together to develop data governance frameworks that not only protect data but also ensure compliance with laws such as the General Data Protection Regulation (GDPR) or the Health Insurance Portability and Accountability Act (HIPAA).
Tools and Techniques
Data governance relies on a combination of processes, policies, and tools to manage and control data effectively. These tools may include data governance software, metadata management systems, data lineage tools, and data quality management solutions. Data governance frameworks, such as DAMA-DMBOK, provide guidance on establishing data governance practices and selecting appropriate tools.
Data analytics utilizes a wide range of tools and techniques, including statistical software packages (e.g., R, Python), data mining algorithms, machine learning algorithms, and data visualization tools. These tools enable data analysts to explore and analyze data effectively and derive meaningful insights from complex datasets.
Furthermore, advancements in technology have led to the emergence of specialized tools for data governance and data analytics. For instance, data governance software not only helps organizations establish data policies but also provides features like data cataloging, data lineage visualization, and data access controls. Similarly, data analytics platforms offer integrated environments where data analysts can perform data exploration, modeling, and visualization tasks seamlessly.
In conclusion, while data governance and data analytics are distinct disciplines, they are interconnected and complementary. Data governance ensures the availability of high-quality data for analytics, while data analytics uncovers valuable insights that can inform and improve data governance practices. Together, they contribute to the effective and responsible use of data in organizations across various industries.
The Role of Data Governance in Business
Ensuring Data Quality
Data governance plays a crucial role in ensuring the accuracy, consistency, and completeness of data. By implementing standardized data management practices, organizations can eliminate duplicate records, resolve data inconsistencies, and improve data quality. Reliable and trusted data is essential for making informed business decisions and driving operational efficiency.
Data governance also helps organizations comply with regulatory requirements related to data privacy and security. By establishing data governance policies and procedures, organizations can protect sensitive information, prevent data breaches, and mitigate regulatory risks.
Compliance and Security Measures
Data governance helps organizations establish a robust framework for compliance with data protection and privacy regulations, such as the General Data Protection Regulation (GDPR) or the Health Insurance Portability and Accountability Act (HIPAA). By implementing data governance practices, organizations can ensure that data is handled and processed in accordance with legal and ethical requirements.
Data governance also helps organizations implement appropriate security measures to protect data from unauthorized access, data breaches, and cyber threats. This includes defining access controls, encryption standards, and data classification policies to safeguard sensitive information.
The Impact of Data Analytics on Business Performance
Informed Decision Making
Data analytics enables organizations to make data-driven decisions based on factual insights rather than intuition or guesswork. By analyzing historical data and identifying trends, organizations can uncover valuable insights that can inform strategic decision-making, optimize business processes, and drive innovation.
By leveraging data analytics, organizations can gain a competitive advantage by identifying emerging market trends, understanding customer preferences, and predicting future demand patterns. This enables better resource allocation, improved customer targeting, and enhanced overall business performance.
Predictive Analysis and Future Planning
Data analytics enables organizations to predict future outcomes and trends based on historical data patterns. By building predictive models and conducting scenario analysis, organizations can anticipate potential risks, identify growth opportunities, and develop effective strategies to navigate changing market conditions.
Through advanced analytics techniques, such as machine learning and artificial intelligence, organizations can uncover hidden patterns and correlations in data, leading to more accurate forecasting and better long-term planning.
Choosing Between Data Governance and Data Analytics
Assessing Your Business Needs
When deciding whether to prioritize data governance or data analytics, organizations should carefully assess their specific business needs and priorities. If the primary concern is ensuring data accuracy, reliability, and compliance, data governance should be the top priority. However, if the organization's key objective is extracting valuable insights from data to drive business growth and innovation, data analytics should take precedence.
Organizations should consider the nature of their industry, the scale of their data operations, and the level of regulatory compliance requirements when determining the importance of data governance and data analytics in their unique context.
Evaluating Your Resources
Implementing data governance or data analytics requires adequate resources, including skilled personnel, tools, and technology infrastructure. Organizations should assess their existing capabilities and resources to determine whether they have the necessary expertise and infrastructure to support either data governance or data analytics initiatives.
If the organization lacks sufficient resources for both, it may be necessary to prioritize one approach over the other. This prioritization should align with the organization's strategic objectives and long-term goals.
Understanding Your Long-Term Goals
Lastly, organizations should consider their long-term objectives when choosing between data governance and data analytics. If the focus is on establishing a strong foundation for data management, ensuring compliance, and establishing data quality, data governance should be the primary focus. However, if the organization's vision includes leveraging data as a strategic asset, driving innovation, and achieving a competitive advantage, data analytics becomes paramount.
Ultimately, a balanced approach that considers both data governance and data analytics is ideal. By prioritizing data governance in the early stages and gradually incorporating data analytics capabilities, organizations can effectively manage data while deriving maximum value from it.
In conclusion, while data governance and data analytics are distinct practices, they are both essential components of effective data management and utilization. Organizations need to define their specific needs, evaluate available resources, and align their long-term goals to determine whether data governance or data analytics should take precedence in their strategic roadmap. By implementing a well-rounded approach that combines both practices, organizations can build a strong foundation for data-driven decision-making, compliance, and innovation.
You might also like
Get in Touch to Learn More
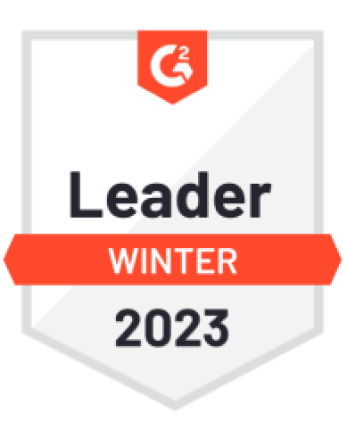
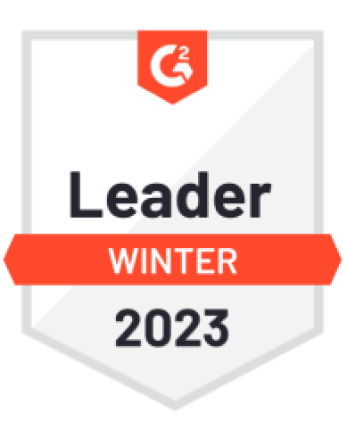

“[I like] The easy to use interface and the speed of finding the relevant assets that you're looking for in your database. I also really enjoy the score given to each table, [which] lets you prioritize the results of your queries by how often certain data is used.” - Michal P., Head of Data