Data Lineage Explained Through Examples
Discover the importance of data lineage and gain a deeper understanding through real-world examples in this comprehensive article.

Data lineage is a critical aspect of data management that provides insights into the origins, transformations, and movements of data within an organization. By mapping the journey of data from its source to its destination, data lineage offers transparency and traceability, ensuring data quality and compliance.
Understanding the Concept of Data Lineage
At its core, data lineage refers to the ability to track and document the flow of data throughout its lifecycle. It helps organizations answer questions such as where the data came from, how it was transformed, and where it is currently being used.
Data lineage plays a crucial role in ensuring data integrity and transparency within an organization. By providing a detailed history of data movement and transformation, it enables data stewards and analysts to make informed decisions based on accurate and reliable information. This level of insight is especially valuable in industries where regulatory compliance and data security are top priorities.
Definition and Importance of Data Lineage
Data lineage can be defined as the end-to-end traceability of data, enabling organizations to understand the provenance, transformation, and consumption of their data assets. It captures information about data sources, data transformations, data quality checks, and data consumption. This level of visibility is crucial not only for compliance but also for improving data governance, data quality management, and decision-making processes.
Furthermore, data lineage serves as a foundation for data lineage mapping, which involves creating visual representations of data flows to identify dependencies and relationships between different data elements. This mapping process helps organizations optimize their data architecture, streamline data processes, and uncover potential bottlenecks or inefficiencies in their data pipelines.
Key Components of Data Lineage
Data lineage is composed of several key components: data sources, data transformations, data quality checks, and data consumption. Data sources include databases, files, applications, or external providers that generate or provide data to the organization. Data transformations involve any changes or modifications that occur to the data as it moves through different processes or systems. Data quality checks ensure that the data meets specific standards and guidelines. Finally, data consumption involves the various ways in which the data is used within the organization, such as reporting, analysis, or decision-making.
Overall, a comprehensive understanding of data lineage empowers organizations to establish data governance best practices, enhance data quality and accuracy, and drive strategic business initiatives with confidence and clarity. By leveraging data lineage insights, organizations can unlock the full potential of their data assets and gain a competitive edge in today's data-driven landscape.
The Role of Data Lineage in Data Management
Data lineage plays a pivotal role in effective data management, providing organizations with the necessary insights to make informed decisions and ensure data quality and compliance.
Understanding the lineage of data is like tracing the ancestry of information within an organization. It involves tracking the origins, movements, and transformations of data from its source to its destination. This comprehensive view allows organizations to not only comprehend how data is created and utilized but also to identify any potential risks or dependencies associated with it.
Data Governance and Data Lineage
Data governance encompasses the policies, processes, and technologies that organizations implement to manage and protect their data assets. Data lineage contributes to data governance by providing a clear understanding of data flow, facilitating data discovery, and enabling organizations to establish data stewardship and accountability.
Imagine data governance as the guardian of an organization's data integrity, ensuring that information is handled responsibly and ethically. Data lineage acts as the map that guides this guardian, showing the intricate paths data traverses and highlighting any areas of vulnerability or non-compliance. By integrating data lineage into data governance practices, organizations can enhance their data management strategies and fortify their data-driven decision-making processes.
Data Lineage in Data Quality Management
Data quality is a critical component of any organization's data management strategy. Data lineage offers insights into data quality issues by identifying potential sources of errors or anomalies throughout the data lifecycle. By tracking data lineage, organizations can detect and address data quality issues effectively, ensuring the reliability and accuracy of their data.
Picture data lineage as a detective in the realm of data quality management, diligently investigating the whereabouts and conditions of data as it travels through various systems and processes. This detective work not only helps in uncovering discrepancies or inconsistencies in data but also aids in maintaining a high standard of data quality. With data lineage as a key ally, organizations can proactively manage data quality challenges, uphold the trustworthiness of their data assets, and ultimately drive better business outcomes.
The Process of Data Lineage
The process of data lineage involves various stages and requires the use of appropriate tools and techniques to capture and represent data flow within an organization.
Understanding the journey of data within an organization is crucial for ensuring data quality, compliance, and decision-making processes. Data lineage provides a detailed map of how data moves through systems, applications, and processes, helping organizations trace the origins of data elements and understand their transformations.
Stages of Data Lineage
The process of data lineage typically involves three stages: data discovery, data mapping, and data visualization. Data discovery involves identifying the sources of data within the organization. Data mapping refers to the process of capturing and documenting the flow of data between different systems or processes. Data visualization allows stakeholders to easily understand and interpret the data lineage through visual representations, such as diagrams or flowcharts.
During the data discovery stage, organizations conduct thorough assessments to identify all data sources, including databases, applications, and files. This stage often involves collaboration with data owners and subject matter experts to ensure comprehensive coverage of data origins.
Tools and Techniques for Data Lineage
There are several tools and techniques available to facilitate the capture, management, and visualization of data lineage. These include metadata management systems, data lineage tools, and data modeling techniques. Metadata management systems enable organizations to store and manage metadata, such as data definitions, data sources, and data transformations. Data lineage tools automate the process of capturing and documenting data lineage, providing organizations with a comprehensive view of data flow. Data modeling techniques, such as entity-relationship diagrams, can be used to represent data lineage visually.
Advanced data lineage tools offer features such as impact analysis, which helps organizations understand the downstream effects of changes to data sources or structures. These tools also support data governance initiatives by establishing data quality rules and tracking data usage across the organization.
Challenges and Solutions in Data Lineage
Implementing data lineage comes with its own set of challenges. However, organizations can overcome these hurdles by adopting effective strategies and best practices.
Common Obstacles in Implementing Data Lineage
Some of the common challenges in implementing data lineage include data silos, lack of metadata management, complex data transformations, and the need for cross-functional collaboration. Data silos occur when different departments or systems within an organization have isolated data repositories, making it difficult to track data lineage. Metadata management, including the consistent documentation of data definitions and transformations, is crucial for accurate data lineage. Complex data transformations, such as data aggregations or derivations, can pose challenges in capturing and representing data lineage. Effective implementation of data lineage requires cross-functional collaboration between IT, data governance, and business stakeholders.
Strategies for Effective Data Lineage
To overcome the challenges associated with data lineage, organizations can adopt several strategies. Breaking down data silos and establishing a centralized data repository can facilitate the capture and management of data lineage. Implementing metadata management systems and establishing data governance practices enable consistent documentation of data definitions and transformations. Organizations should prioritize data quality checks and establish data stewardship practices to ensure the reliability and accuracy of data lineage. Lastly, fostering cross-functional collaboration and involving business stakeholders throughout the data lineage implementation process ensures that data lineage serves the needs of the entire organization.
The Future of Data Lineage
As organizations continue to generate and consume massive amounts of data, data lineage is expected to evolve and adapt to emerging technologies and trends.
Emerging Trends in Data Lineage
One of the emerging trends in data lineage is the integration of artificial intelligence (AI) and machine learning (ML) technologies. AI and ML can automate the capture and interpretation of data lineage, making it easier for organizations to maintain and update data lineage information in real-time. Additionally, advancements in big data technologies, such as Hadoop and cloud computing, are expected to enhance the scalability and performance of data lineage solutions.
The Impact of AI and Machine Learning on Data Lineage
AI and ML technologies are poised to revolutionize data lineage by enabling organizations to automate the discovery, mapping, and visualization of data flow. By leveraging AI and ML algorithms, organizations can not only reduce the manual effort required in capturing and managing data lineage but also gain more comprehensive insights into data lineage patterns and dependencies.
In conclusion, data lineage is an integral part of data management, providing organizations with valuable insights into the flow and transformation of their data. By understanding the concept of data lineage, its role in data governance and data quality management, the process involved, and the challenges and solutions, organizations can harness the power of data lineage to ensure data quality, compliance, and informed decision-making. As the industry continues to advance, embracing emerging technologies like AI and ML will further enhance the effectiveness of data lineage, paving the way for a data-driven future.
Ready to elevate your organization's data management capabilities? Try CastorDoc today and experience firsthand how our advanced governance, cataloging, and lineage capabilities, combined with a user-friendly AI assistant, can transform your approach to self-service analytics. CastorDoc is not just a tool; it's your partner in unlocking the full potential of your data, ensuring compliance, and empowering informed decision-making. Embrace the future of data management with CastorDoc and turn your data into a strategic asset.
You might also like
Get in Touch to Learn More
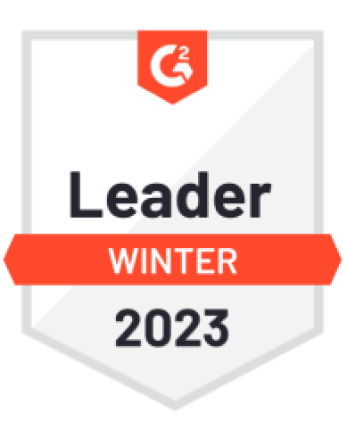
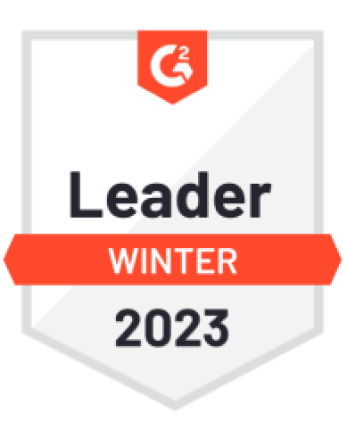

“[I like] The easy to use interface and the speed of finding the relevant assets that you're looking for in your database. I also really enjoy the score given to each table, [which] lets you prioritize the results of your queries by how often certain data is used.” - Michal P., Head of Data