Data Mesh vs Data Fabric: What Suits You & How to Choose Them?
Explore the differences between Data Mesh and Data Fabric to determine which one best suits your organization's needs.

Organizations are constantly seeking effective solutions to manage and harness the power of their data. Two popular approaches that have gained significant attention are Data Mesh and Data Fabric. But what exactly are these concepts and how do they differ from each other? In this article, we will delve into the basics of Data Mesh and Data Fabric, explore their key differences, weigh their advantages and disadvantages, and provide guidance on how to determine which approach best suits your needs.
Understanding the Basics: Data Mesh and Data Fabric
Defining Data Mesh
Data Mesh is a decentralized approach to data architecture that aims to address the challenges of scalability and flexibility in managing complex data ecosystems. It shifts the traditional centralized data infrastructure model to a domain-oriented structure, where each domain is responsible for its own data products and operates as an independent business unit.
This shift to a domain-oriented architecture enables teams within an organization to take ownership of their data and promote a culture of data-driven decision-making. Data Mesh encourages the adoption of product thinking, which means treating data as a product and designing it to serve specific business needs. This approach empowers domain teams to independently design, build, and operate their data products, fostering agility and autonomy.
For example, imagine a large e-commerce company that operates in multiple countries. With Data Mesh, each country's domain team can have control over their own data products, such as customer data, sales data, and inventory data. They can tailor their data products to meet the specific needs of their market, allowing for localized decision-making and faster response times to market trends. This level of autonomy also fosters innovation, as domain teams can experiment with different data models and technologies without impacting the entire organization.
Defining Data Fabric
Data Fabric, on the other hand, is a centralized approach to data architecture that focuses on creating a unified and consistent data layer across an organization. It provides a comprehensive view of the enterprise data assets, regardless of their location or format. Data Fabric acts as a virtual layer that abstracts the underlying complexity of data integration and management, enabling seamless access and interaction with data.
The key principle of Data Fabric is to provide a single source of truth for data, ensuring consistency, reliability, and quality. It achieves this by harmonizing data across the organization, eliminating data silos, and establishing standardized data governance practices. Data Fabric also facilitates the integration of diverse data sources, such as structured, semi-structured, and unstructured data, into a coherent and unified view.
For instance, let's consider a global financial institution that has multiple business units spread across different regions. With Data Fabric, the organization can create a centralized data layer that consolidates data from various sources, such as customer transactions, market data, and regulatory information. This unified view of data enables the institution to gain insights into its operations, identify patterns, and make informed decisions at a global level. Data Fabric also ensures data consistency and accuracy by implementing data quality checks and data cleansing processes, resulting in improved data reliability and trustworthiness.
In summary, while Data Mesh promotes decentralization and empowers domain teams to take ownership of their data products, Data Fabric focuses on centralization and creating a unified data layer. Both approaches have their merits and can be applied based on the specific needs and goals of an organization. Ultimately, the choice between Data Mesh and Data Fabric depends on factors such as the organization's size, complexity of data ecosystems, and desired level of control and standardization.
Key Differences Between Data Mesh and Data Fabric
Architecture and Design
In terms of architecture, Data Mesh follows a decentralized model, with autonomous domain teams responsible for their own data products. On the other hand, Data Fabric adopts a centralized model, where data governance and integration are managed centrally.
The design philosophy of Data Mesh promotes a domain-oriented approach, with each domain owning and managing their data products independently. In contrast, Data Fabric emphasizes a unified and consistent design across the entire organization, aiming to create a seamless data ecosystem.
Data Integration and Management
Data Mesh places the responsibility of data integration and management on individual domain teams. Each team has the autonomy to decide on the tools, technologies, and approaches they use to integrate and manage their data products. This flexibility allows domains to choose solutions that are best suited to their specific requirements.
On the other hand, Data Fabric centralizes data integration and management, providing a standardized and controlled environment for data integration processes. It offers a comprehensive suite of tools and technologies that enable efficient data integration, data quality management, and data governance throughout the organization.
Scalability and Flexibility
Data Mesh offers scalability and flexibility by distributing the responsibility of data products across individual domains. This decentralized approach allows for faster development and deployment of data products, enabling teams to adapt to changing business needs more rapidly.
Data Fabric, on the other hand, provides scalability and flexibility through its centralized data infrastructure. By establishing a unified data layer, it facilitates seamless access and integration of data, enabling organizations to scale and evolve their data capabilities with ease.
Advantages and Disadvantages of Data Mesh
Pros of Using Data Mesh
One of the significant advantages of Data Mesh is its ability to foster collaboration and ownership within domain teams. By empowering teams to manage their own data products, it promotes a sense of responsibility and accountability. This distributed ownership leads to improved data quality, as teams have a vested interest in ensuring accurate and reliable data.
Data Mesh also enables agility and faster time-to-market. Autonomous domain teams can develop and deploy data products independently, eliminating bottlenecks and reducing dependencies on centralized teams. This agility allows organizations to respond quickly to changing business requirements and gain a competitive edge.
Another advantage of Data Mesh is its scalability. As organizations grow and their data needs expand, domain teams can easily scale their data products without impacting the overall data ecosystem. This scalability enables organizations to accommodate evolving data requirements while maintaining a high level of performance and efficiency.
Cons of Using Data Mesh
One potential downside of Data Mesh is the increased complexity of managing a decentralized data ecosystem. Each domain team operates independently, leading to potential inconsistencies in data modeling, data governance, and data integration. This decentralized nature requires robust coordination and communication mechanisms to ensure alignment and avoid data fragmentation.
Another challenge with Data Mesh is the need for domain teams to acquire specialized skills and knowledge to effectively manage their data products. This can present a learning curve and additional training requirements for domain teams, especially if they are not accustomed to working with data infrastructure and governance.
Advantages and Disadvantages of Data Fabric
Pros of Using Data Fabric
Data Fabric offers a unified and consistent view of data across the organization, ensuring data quality, reliability, and accessibility. By centralizing data integration and management, it eliminates data silos and enables seamless data sharing and collaboration.
One of the strengths of Data Fabric is its ability to provide a scalable and extensible data architecture. Centralized data infrastructure simplifies the management and governance of data, making it easier to scale and adapt to evolving business needs. This scalability ensures that organizations can handle growing data volumes and complexity effectively.
Data Fabric also enables organizations to leverage advanced data analytics and AI capabilities. By creating a unified data layer, it facilitates data discovery, integration, and analysis, allowing organizations to derive valuable insights and make data-driven decisions. This capability opens up opportunities for innovation and competitive advantage.
Cons of Using Data Fabric
One of the potential disadvantages of Data Fabric is the complexity and effort required to establish and maintain a centralized data infrastructure. It involves significant upfront investment in terms of architecture design, data integration, and standardization. Organizations need to carefully plan and execute the implementation to ensure a smooth transition and minimize disruptions.
Another challenge of Data Fabric is the potential for increased reliance on centralized teams for data integration and management. This can create bottlenecks and reduce the agility of an organization in responding to changing business needs. Effective collaboration and communication between centralized teams and domain teams are crucial to maintaining a balance between central control and autonomous decision-making.
Determining What Suits Your Needs
Assessing Your Data Infrastructure
When choosing between Data Mesh and Data Fabric, it is essential to start by assessing your existing data infrastructure and understanding its strengths and weaknesses. Evaluate the maturity of your data management practices and identify any pain points or gaps in your current approach.
Consider factors such as the complexity of your data ecosystem, the volume and variety of data you deal with, and the scalability requirements of your organization. Assessing your data infrastructure will help you determine whether a decentralized approach like Data Mesh or a centralized approach like Data Fabric is more suitable for your needs.
Evaluating Your Business Requirements
Alongside your data infrastructure assessment, it is crucial to evaluate your business requirements and objectives. Consider factors such as the level of collaboration and autonomy you want to provide to domain teams, the need for agility and faster time-to-market, and the importance of data consistency and governance.
Based on your business requirements and priorities, you can weigh the advantages and disadvantages of Data Mesh and Data Fabric. It is also worth considering potential hybrid approaches that combine the strengths of both concepts to create a tailored solution that fits your specific needs.
Remember, there is no one-size-fits-all solution when it comes to data architecture. Each organization is unique and requires an approach that aligns with its goals, capabilities, and culture. By carefully analyzing your data infrastructure and business requirements, you can make an informed decision and choose the approach that best suits you - whether it be Data Mesh, Data Fabric, or a hybrid model.
In conclusion, Data Mesh and Data Fabric offer distinct approaches to managing and leveraging data within organizations. While Data Mesh empowers autonomous domain teams and promotes a distributed ownership model, Data Fabric focuses on centralized data integration and governance. To determine the best approach for your organization, assess your data infrastructure and evaluate your business requirements. By striking the right balance between autonomy and centralization, you can unlock the full potential of your data and drive business success.
You might also like
Get in Touch to Learn More
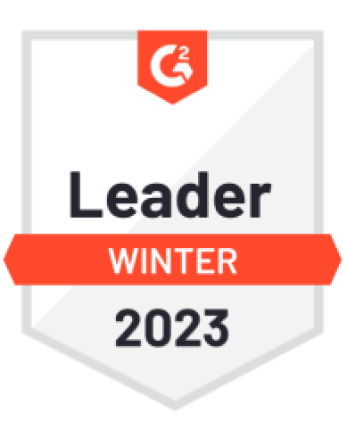
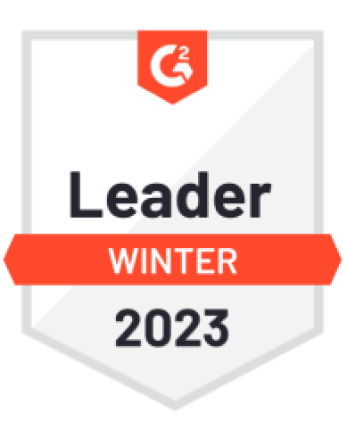

“[I like] The easy to use interface and the speed of finding the relevant assets that you're looking for in your database. I also really enjoy the score given to each table, [which] lets you prioritize the results of your queries by how often certain data is used.” - Michal P., Head of Data