Data Quality in Data Governance: The Crucial Link That Ensures Data Accuracy and Integrity
Discover the vital role of data quality in data governance and how it ensures the accuracy and integrity of your organization's data.

In the era of big data and advanced analytics, the importance of data quality cannot be overstated. Data quality plays a pivotal role in ensuring the accuracy and integrity of organizational data, which in turn impacts the overall success of data governance initiatives. Without high-quality data, data governance is rendered ineffective, leading to erroneous insights, flawed decision-making, and potential compliance and legal issues.
Understanding the Concept of Data Quality
Data quality refers to the accuracy, completeness, consistency, and reliability of data. It encompasses various dimensions, including validity, timeliness, relevance, and uniqueness, among others. In simple terms, data quality determines the extent to which data meets its intended purpose and can be trusted for decision-making.
Defining data quality precisely can be challenging since it varies across industries and organizations. However, key characteristics such as accuracy, reliability, consistency, and integrity are universally acknowledged as essential elements of high-quality data.
Defining Data Quality
Data quality can be defined as the measure of the degree to which data meets specific criteria or requirements. It encompasses processes, technologies, and methodologies used to ensure data accuracy and reliability. Data quality factors include completeness, consistency, timeliness, uniqueness, and precision.
While data quality is influenced by various technical aspects, it is also impacted by business rules, governance policies, and user expectations. Organizations must consider both technical and non-technical factors when defining data quality standards and aligning them with their strategic goals.
Importance of High-Quality Data
High-quality data is vital for organizations across all sectors and industries. It serves as the foundation for reliable insights, informed decision-making, and effective business strategies. When data quality is compromised, organizations face numerous challenges, including:
- Limited trust in data: Inaccurate or incomplete data reduces confidence in its reliability, making it difficult for decision-makers to rely on it.
- Inefficient operations: Poor data quality leads to inefficiencies in processes, resulting in wasted time, effort, and resources.
- Missed opportunities: Inaccurate or outdated data can prevent organizations from identifying growth opportunities, understanding customer needs, and staying competitive.
Furthermore, high-quality data enables organizations to gain a competitive edge by providing them with accurate insights into market trends, customer behavior, and operational efficiency. It allows businesses to make data-driven decisions that can lead to improved customer satisfaction, increased revenue, and enhanced overall performance.
Moreover, data quality plays a crucial role in regulatory compliance. Many industries, such as healthcare and finance, have strict regulations regarding data privacy and security. Ensuring high-quality data not only helps organizations meet these regulatory requirements but also protects sensitive information from unauthorized access or breaches.
Additionally, high-quality data fosters better collaboration and integration within an organization. When data is accurate, consistent, and reliable, different departments can trust and rely on the same information, leading to improved communication, streamlined processes, and better coordination.
In conclusion, data quality is a critical aspect of any organization's success. It impacts decision-making, operational efficiency, competitive advantage, regulatory compliance, and internal collaboration. By prioritizing data quality and implementing robust data management practices, organizations can unlock the full potential of their data and drive meaningful business outcomes.
The Role of Data Governance in Maintaining Data Quality
Data governance is the framework that organizations use to manage and protect their data assets effectively. It encompasses the processes, policies, standards, and guidelines that ensure data is maintained, protected, and utilized in a controlled and governed environment. Data governance plays a crucial role in maintaining data quality through:
One key principle of data governance is accountability. Clearly defining roles, responsibilities, and ownership for data quality management ensures that everyone understands their role in maintaining data quality. When individuals are held accountable for the quality of data, they are more likely to take the necessary steps to ensure its accuracy and reliability.
In addition to accountability, transparency is another important principle of data governance. Making data quality processes and policies visible and accessible to all stakeholders promotes trust and collaboration. When stakeholders have a clear understanding of how data quality is managed, they can actively contribute to its improvement and identify any potential issues or gaps.
Consistency is also a fundamental principle of data governance. Ensuring that data quality practices are applied consistently across the organization helps to maintain a high level of data integrity. By establishing standardized processes and guidelines, organizations can minimize variations in data quality and ensure that data is reliable and consistent across different systems and departments.
Continuous improvement is an essential aspect of data governance. Regularly evaluating and enhancing data quality practices allows organizations to adapt to changing requirements and emerging technologies. By staying proactive and continuously improving data quality processes, organizations can mitigate risks and maintain a high level of data accuracy and reliability.
Data Governance Strategies for Ensuring Data Quality
Data governance strategies can help organizations maintain data quality by providing a systematic approach to data management. Some common strategies include:
- Data profiling and cleansing: Regularly analyzing and cleaning data to identify and correct any inconsistencies or errors. Data profiling involves examining the data to understand its structure, content, and quality. By identifying any issues or anomalies, organizations can take corrective actions to improve data quality.
- Data standardization: Implementing standardized formats and structures to ensure consistency and interoperability. Standardizing data formats and structures helps to eliminate variations and inconsistencies, making it easier to integrate and analyze data from different sources. This promotes data accuracy and enhances the overall quality of the data.
- Data documentation: Documenting metadata, data lineage, and data definitions to enhance understanding and trust in data. By documenting important information about the data, such as its source, transformations, and meaning, organizations can improve data governance and ensure that data is interpreted correctly. This documentation also helps in data lineage analysis, which traces the origin and transformation of data, further enhancing data quality.
- Data stewardship: Appointing data stewards who are responsible for ensuring data quality and adherence to governance policies. Data stewards play a critical role in data governance by overseeing data quality initiatives, resolving data-related issues, and enforcing data governance policies. They act as advocates for data quality and help drive continuous improvement efforts.
By implementing these data governance strategies, organizations can establish a strong foundation for maintaining data quality. These strategies provide a structured approach to data management, ensuring that data is accurate, reliable, and fit for its intended purpose. With effective data governance in place, organizations can make informed decisions, drive innovation, and gain a competitive edge in today's data-driven world.
The Interconnection Between Data Quality and Data Governance
Data quality and data governance are interconnected and mutually reinforcing. Data governance provides the framework and processes necessary to establish and maintain data quality, while data quality acts as a measure of the effectiveness of data governance efforts.
How Data Governance Influences Data Quality
Data governance influences data quality in several ways:
- Establishing data standards: Data governance ensures the establishment and enforcement of data standards, leading to improved data consistency and accuracy.
- Enforcing data policies: Data governance policies govern the collection, storage, and sharing of data, ensuring adherence to quality requirements.
- Defining data ownership: Data governance clarifies data ownership, accountability, and responsibility, enhancing data quality management.
The Impact of Data Quality on Effective Data Governance
High-quality data is crucial for effective data governance as it enables organizations to make informed decisions, mitigate risks, and comply with regulatory requirements. Data quality impacts data governance in several ways:
- Trustworthy insights: High-quality data ensures accurate and reliable insights, enabling decision-makers to have confidence in the data-driven decisions they make.
- Compliance and risk management: Data quality is essential for compliance with regulations and reducing the risk of legal and financial consequences resulting from data inaccuracies.
- Efficient operations: With reliable data, organizations can streamline processes, optimize resource utilization, and improve operational efficiency.
Ensuring Data Accuracy and Integrity through Data Governance
Data accuracy and integrity are critical aspects of data quality. By implementing robust data governance practices, organizations can maintain data accuracy and integrity effectively.
Techniques for Enhancing Data Accuracy
To enhance data accuracy, organizations can employ various techniques:
- Data validation: Implementing validation rules and checks to ensure data entered into systems meets predefined criteria.
- Data reconciliation: Comparing data from different sources to identify discrepancies and resolve inconsistencies.
- Data profiling: Analyzing data patterns to identify anomalies, outliers, and other data quality issues.
Measures to Maintain Data Integrity
Data integrity refers to the consistency, accuracy, and reliability of data throughout its lifecycle. Some measures to maintain data integrity include:
- Data encryption: Encrypting data at rest and in transit to prevent unauthorized access and tampering.
- Data backups: Regularly backing up data to ensure recoverability in case of data loss or corruption.
- Data access controls: Implementing strict access controls to ensure only authorized users can modify or delete data.
Challenges in Implementing Data Quality in Data Governance
Implementing data quality within data governance initiatives is not without challenges. Organizations must overcome these obstacles to ensure successful data governance and high-quality data.
Common Obstacles in Data Quality Management
Some common obstacles organizations face in data quality management include:
- Lack of data governance buy-in: Without strong support from senior management, implementing data quality initiatives becomes challenging.
- Data silos: Data silos hinder the integration and standardization of data, making data quality management difficult.
- Poor data documentation: Inadequate documentation hampers understanding of data lineage and definitions, impacting data quality management.
Overcoming Challenges in Data Governance Implementation
To overcome challenges in data governance implementation, organizations can adopt various strategies:
- Executive sponsorship: Securing executive support and sponsorship is essential for successful data governance implementation.
- Data integration: Breaking down data silos and integrating disparate data sources enable a holistic view of data, promoting data quality management.
- Data governance frameworks: Implementing robust data governance frameworks provides structure and guidelines for effectively managing data quality.
Conclusion
Data quality is the cornerstone of data governance, ensuring the accuracy and integrity of organizational data. Organizations must understand the concept of data quality, its importance, and the interconnection between data quality and data governance. By implementing data governance strategies, organizations can enhance data accuracy and integrity while overcoming challenges in data quality management. High-quality data, backed by effective data governance, empowers organizations to make informed decisions, increase operational efficiency, and drive success in the data-driven era.
You might also like
Get in Touch to Learn More
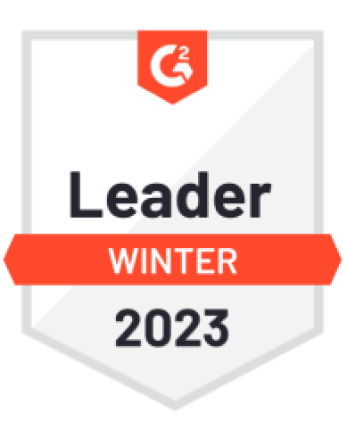
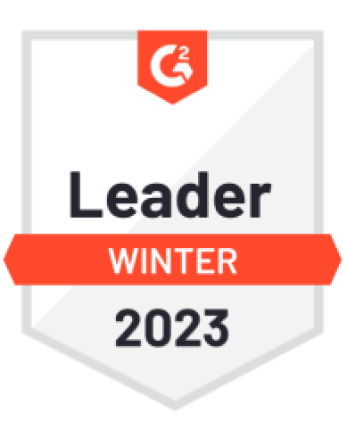

“[I like] The easy to use interface and the speed of finding the relevant assets that you're looking for in your database. I also really enjoy the score given to each table, [which] lets you prioritize the results of your queries by how often certain data is used.” - Michal P., Head of Data