How to Avoid Bad Data in 2024?
Learn effective strategies and tips to steer clear of bad data in 2024 with our comprehensive guide.

In today's digital age, data has become the lifeblood of businesses. However, with the exponential growth in data volume, it has become increasingly difficult to maintain data quality. Bad data, if not effectively managed, can have serious consequences for businesses, ranging from skewed analytics to inefficient decision-making. In this article, we will explore the concept of bad data, its impact on businesses, and strategies to prevent it in the year 2024.
Understanding the Concept of Bad Data
Before delving into strategies to avoid bad data, it is important to grasp the concept itself. Bad data refers to inaccurate, inconsistent, incomplete, or outdated information that adversely affects the integrity and reliability of a dataset. It can originate from various sources such as human errors, system glitches, or outdated processes.
One common source of bad data is data entry errors, where individuals input incorrect information due to typos or lack of attention to detail. Another source is data migration processes, where data is transferred between systems inaccurately, leading to inconsistencies and errors. Additionally, outdated data management practices can contribute to bad data, as information becomes obsolete but is not updated or removed.
Defining Bad Data
Bad data encompasses a wide range of issues, including duplicate records, misspellings, incorrect values, and inconsistent formats. These anomalies can lead to misleading analysis and flawed insights.
Moreover, bad data can also include incomplete information, where crucial data points are missing, making it challenging to draw accurate conclusions or make informed decisions. Inconsistencies in data formatting, such as mixing date formats or using different units of measurement, can further compound the problem, making data integration and analysis more complex and error-prone.
The Impact of Bad Data on Businesses
The consequences of bad data can be severe, directly impacting a business's bottom line. Inaccurate customer information, for example, can result in failed marketing campaigns or delivery mishaps. Furthermore, bad data can erode customer trust, damage brand reputation, and hinder effective decision-making.
Businesses that rely on data-driven strategies may find their efforts compromised by bad data, leading to misguided business decisions and missed opportunities for growth. Inaccurate sales forecasts, for instance, can result in inventory mismanagement and revenue loss. Additionally, regulatory compliance can be jeopardized if critical data used for reporting purposes is flawed, potentially exposing the business to legal risks and financial penalties.
The Evolution of Data Quality Management
Data quality management techniques have come a long way in recent years. As businesses strive for more accurate and reliable data, innovative approaches have emerged to tackle the challenges posed by bad data.
In addition to the advancements in data quality management, the evolution of technology has also played a crucial role in shaping the landscape. With the proliferation of Internet of Things (IoT) devices and the exponential growth of data generated, the need for robust data quality management practices has become more pressing than ever before. This has led to a paradigm shift in how organizations perceive and prioritize data quality.
The Past and Present of Data Quality
Historically, data quality management has been a reactive process, with businesses attempting to fix errors after they occur. Today, the focus has shifted to proactive strategies that prevent bad data from entering the system in the first place. This shift has led to the rise of data governance frameworks and advanced data quality tools.
Furthermore, the increasing globalization of businesses and the interconnected nature of data systems have added layers of complexity to data quality management. Organizations now face the challenge of maintaining data quality across diverse sources and formats, requiring a more holistic approach to ensure consistency and accuracy.
Predictions for Data Quality in 2024
Looking ahead to the year 2024, we can expect further advancements in data quality management. Artificial intelligence and machine learning algorithms are expected to play a significant role in automating data validation and cleansing processes, reducing the risk of bad data entering critical systems.
Moreover, the emergence of blockchain technology is poised to revolutionize data quality management by providing a secure and transparent framework for data transactions. By leveraging blockchain for data verification and validation, organizations can enhance the trustworthiness and integrity of their data, paving the way for new standards in data quality assurance.
Identifying Sources of Bad Data
To effectively prevent bad data, it is crucial to identify its sources. Bad data can originate from both internal and external factors. Understanding the root causes of bad data is essential for maintaining data integrity and making informed decisions based on accurate information.
One additional internal source of bad data to consider is system errors or glitches within the data management software. These technical issues can lead to data corruption or loss, impacting the overall quality of the data. Implementing robust IT infrastructure, regular system maintenance, and data backup procedures are vital to mitigate the risks associated with system-related bad data.
Moreover, external factors contributing to bad data can extend beyond data feeds and third-party sources. Environmental factors such as natural disasters, power outages, or cyberattacks can disrupt data transmission and storage, leading to inaccuracies or incompleteness in the datasets. Developing a comprehensive disaster recovery plan, investing in secure data storage solutions, and implementing encryption protocols can safeguard against external threats to data integrity.
Common Internal Sources of Bad Data
Internal sources of bad data include human errors during data entry, data integration issues, and inadequate data validation processes. These issues can be addressed through employee training, improved data entry protocols, and the establishment of data quality standards.
External Factors Contributing to Bad Data
External sources of bad data can include data feeds from unreliable sources, outdated third-party databases, and unreliable APIs. To tackle this, businesses should establish stringent data acquisition procedures, conduct regular audits of external data sources, and collaborate closely with data providers.
Strategies to Prevent Bad Data
Preventing bad data requires a combination of proactive strategies and technological solutions. Let's explore some effective approaches.
Implementing Data Governance
Data governance involves establishing processes, roles, and responsibilities to ensure data quality throughout its lifecycle. This comprehensive approach not only focuses on data accuracy but also addresses data security, privacy, and compliance. By implementing data governance frameworks, businesses can create a culture of data stewardship and accountability, leading to improved decision-making and operational efficiency.
Furthermore, data governance helps organizations maintain data lineage and traceability, crucial for regulatory audits and internal investigations. It also facilitates collaboration between different departments by providing a unified understanding of data definitions and usage, ultimately enhancing cross-functional alignment and strategic initiatives.
Leveraging Data Quality Tools
Data quality tools can significantly aid in the prevention of bad data. These sophisticated tools leverage artificial intelligence and machine learning algorithms to continuously monitor data quality, detect patterns, and predict potential issues. By automating data profiling, cleansing, and enrichment processes, businesses can streamline their data management efforts and ensure high-quality, reliable data for analysis and decision-making.
Moreover, data quality tools offer real-time monitoring capabilities, enabling organizations to proactively identify and address data discrepancies as soon as they arise. This proactive approach not only minimizes the impact of bad data on business operations but also enhances customer satisfaction and trust by ensuring accurate and consistent information across all touchpoints.
Ensuring Data Accuracy and Consistency
While prevention is crucial, businesses must also establish measures to ensure data accuracy and consistency on an ongoing basis. In today's fast-paced digital landscape, where data serves as the lifeblood of decision-making processes, maintaining accurate and consistent data is paramount for organizational success.
Regular Data Audits and Cleansing
Regular audits and data cleansing processes are essential to maintain data accuracy. These activities involve identifying and rectifying inconsistencies, updating outdated information, and eliminating duplicate records. By conducting periodic data audits and cleansing exercises, businesses can ensure that their datasets remain reliable and up to date. Moreover, data audits not only help in identifying errors but also provide valuable insights into data usage patterns, which can further optimize data management strategies.
Establishing a Single Source of Truth
One effective way to minimize bad data is to establish a single source of truth – a centralized repository that serves as the authoritative source for all critical data. By consolidating data from various systems into a single source, businesses can reduce the risk of inconsistency and duplication, thus improving overall data quality. This centralized approach not only streamlines data access but also enhances data governance practices, ensuring that decision-makers rely on accurate and consistent information for strategic planning and operational activities.
Furthermore, beyond just data audits and establishing a single source of truth, businesses can leverage advanced technologies such as artificial intelligence and machine learning to automate data quality processes. These technologies can proactively identify anomalies, predict data inconsistencies, and recommend corrective actions in real-time, thereby enhancing data accuracy and consistency at scale.
You might also like
Get in Touch to Learn More
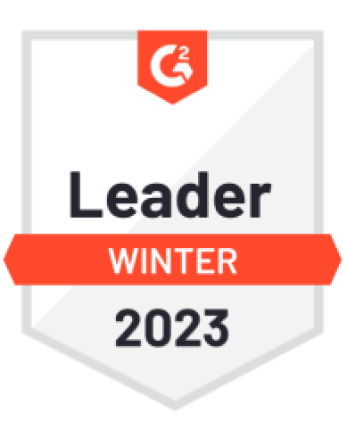
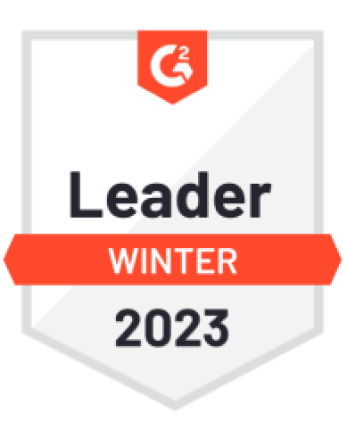

“[I like] The easy to use interface and the speed of finding the relevant assets that you're looking for in your database. I also really enjoy the score given to each table, [which] lets you prioritize the results of your queries by how often certain data is used.” - Michal P., Head of Data