Machine Learning Data Catalog: Evolution, Benefits, Business Impacts and Use Cases in 2024
Explore the transformative power of machine learning data catalogs in 2024, uncovering their evolution, business impacts, and use cases.

Understanding the Evolution of Machine Learning Data Catalogs
The field of machine learning has witnessed significant advancements over the past few decades. One key area that has seen remarkable progress is data management. In particular, the development of machine learning data catalogs has revolutionized the way organizations store, access, and utilize their data. These catalogs serve as a centralized repository for managing and organizing data sets, models, and related metadata.
The early stages of machine learning data catalogs can be traced back to the emergence of traditional data catalogs. These catalogs primarily focused on data governance, providing a means to understand and manage data assets within an organization. However, as machine learning gained prominence, the need for specialized data catalogs that could handle the complexities of machine learning workflows became evident.
In recent years, there have been significant developments in the field of machine learning data catalogs. These developments have been driven by advancements in technology, as well as the growing demand for more versatile and scalable data management solutions. Machine learning data catalogs now offer enhanced features such as automated metadata extraction, data lineage tracking, and advanced search capabilities.
One notable advancement in machine learning data catalogs is the integration of natural language processing (NLP) techniques. By leveraging NLP, data catalogs can now understand and interpret unstructured data, such as text documents and images. This capability opens up new possibilities for organizations to extract valuable insights from a wide range of data sources.
Another area of development in machine learning data catalogs is the incorporation of machine learning algorithms for data quality assessment. These algorithms can automatically identify and flag potential issues in data sets, such as missing values, outliers, or inconsistencies. By providing data quality metrics and recommendations, machine learning data catalogs help organizations ensure the reliability and accuracy of their data.
Looking ahead, there are several predictions for the future of data catalogs. One key prediction is the increased integration of machine learning capabilities within data catalogs. This integration would enable catalogs to automatically discover relationships between datasets, identify patterns, and suggest relevant datasets for specific use cases. Additionally, there is a growing emphasis on improving the user experience of data catalogs, making them more intuitive and user-friendly.
Furthermore, the future of machine learning data catalogs is likely to involve the adoption of blockchain technology. Blockchain, with its decentralized and immutable nature, can provide a secure and transparent framework for data management and sharing. By leveraging blockchain, data catalogs can enhance data privacy, ensure data integrity, and enable seamless collaboration between different stakeholders.
In conclusion, the evolution of machine learning data catalogs has been driven by the need for more efficient and effective data management solutions. With advancements in technology and the growing demand for advanced analytics, these catalogs have become indispensable tools for organizations seeking to leverage the power of machine learning. As we look to the future, the integration of machine learning capabilities, the incorporation of NLP techniques, and the adoption of blockchain technology are expected to further enhance the capabilities and value of machine learning data catalogs.
Unveiling the Benefits of Machine Learning Data Catalogs
Machine Learning Data Catalogs offer a wide range of benefits to organizations across various industries. These benefits can be categorized into three main areas: enhancing data accessibility and organization, improving data quality and consistency, and facilitating better decision-making.
Enhancing Data Accessibility and Organization
One of the key benefits of machine learning data catalogs is their ability to enhance data accessibility and organization. By providing a centralized repository for all data assets, catalogs enable data scientists and analysts to easily discover and access the data they need. This reduces the time spent searching for data and promotes collaboration across teams. Additionally, catalogs allow for the categorization and tagging of datasets, making it easier to find relevant data based on specific criteria.
Imagine a scenario where a data scientist is working on a machine learning project and needs to access a specific dataset. Without a data catalog, they would have to navigate through multiple systems and databases, wasting valuable time and effort. However, with a machine learning data catalog in place, the data scientist can simply search for the dataset using relevant keywords or tags, instantly locating the required data. This streamlined process not only saves time but also ensures that valuable data assets are easily accessible to all stakeholders.
Improving Data Quality and Consistency
Data quality is a crucial factor in ensuring accurate and reliable machine learning outcomes. Machine learning data catalogs help improve data quality by providing tools for data profiling and data cleansing. These catalogs enable organizations to identify and rectify data inconsistencies, outliers, and missing values. By ensuring data consistency, catalogs contribute to the overall reliability of machine learning models and predictions.
Let's consider a practical example. Suppose an organization is using machine learning algorithms to analyze customer data and make personalized product recommendations. However, if the data used for training the models contains inconsistencies or missing values, the accuracy of the recommendations will be compromised. With a machine learning data catalog, organizations can proactively identify and address such data issues. The catalog's data profiling capabilities can automatically detect anomalies and outliers, while its data cleansing tools can help rectify any inconsistencies. This ensures that the machine learning models are built on high-quality data, leading to more accurate predictions and better business outcomes.
Facilitating Better Decision Making
Machine learning data catalogs play a pivotal role in facilitating better decision-making processes. By providing comprehensive metadata about datasets, models, and transformations, catalogs enable data scientists and analysts to make informed decisions. Additionally, catalogs support the reuse of existing models and datasets, allowing organizations to leverage past work and avoid duplication of efforts. This promotes efficiency and enables faster time-to-insights.
Consider a scenario where a data scientist is working on a new project that involves analyzing customer churn. Instead of starting from scratch, the data scientist can refer to the machine learning data catalog to identify existing models and datasets that have been used for similar analyses in the past. By reusing these assets, the data scientist can save time and effort, accelerating the project timeline. Furthermore, the catalog's metadata provides valuable insights into the performance and limitations of the existing models, enabling the data scientist to make informed decisions about their suitability for the current project. This reuse of knowledge and resources not only enhances efficiency but also promotes consistency and standardization across the organization's machine learning initiatives.
The Business Impacts of Machine Learning Data Catalogs
Machine learning data catalogs have profound impacts on businesses of all sizes, across various industries. These impacts can be summarized in terms of transforming business operations, driving competitive advantage, and influencing business strategies and policies.
Transforming Business Operations
Machine learning data catalogs have the potential to transform business operations by streamlining data management processes. With a centralized repository for data assets, organizations can improve data governance, ensure compliance with regulations, and enhance data security. This enables organizations to make more effective use of their data and derive valuable insights to drive business growth.
Driving Competitive Advantage
Machine learning data catalogs provide organizations with a competitive advantage by enabling them to leverage their data for strategic decision making. By effectively managing and organizing data assets, organizations can gain deeper insights into customer behavior, market trends, and operational performance. These insights can then be used to identify untapped opportunities, optimize business processes, and create innovative products and services.
Influencing Business Strategies and Policies
Machine learning data catalogs have a significant impact on shaping business strategies and policies. By providing a comprehensive view of data assets, catalogs facilitate evidence-based decision making. Organizations can use the insights derived from catalogs to develop data-driven strategies, align their business operations with industry trends, and adapt to changing market dynamics. This empowers organizations to stay ahead of the competition and make informed decisions that drive business success.
Use Cases of Machine Learning Data Catalogs in 2024
Machine learning data catalogs have a wide range of applications across various industries. In 2024, these catalogs are expected to be extensively used in several key areas, including predictive analytics and forecasting, customer relationship management, and risk management and fraud detection.
Predictive Analytics and Forecasting
Machine learning data catalogs enable organizations to leverage historical data to build predictive models and perform accurate forecasting. By providing a comprehensive view of relevant datasets and models, catalogs facilitate the identification of trends and patterns. This, in turn, enables organizations to make data-driven predictions, optimize operations, and gain a competitive edge.
Customer Relationship Management
Machine learning data catalogs play a crucial role in enhancing customer relationship management (CRM) processes. By consolidating customer-related data from various sources, catalogs enable organizations to gain a holistic view of customer behavior, preferences, and needs. This allows organizations to personalize their interactions with customers, improve customer satisfaction, and drive customer loyalty.
Risk Management and Fraud Detection
Machine learning data catalogs have significant implications for risk management and fraud detection. By integrating data from multiple sources, catalogs help organizations identify anomalies and patterns that may indicate fraudulent activities. This enables organizations to take proactive measures to mitigate risks, prevent financial losses, and safeguard their reputation.
You might also like
Get in Touch to Learn More
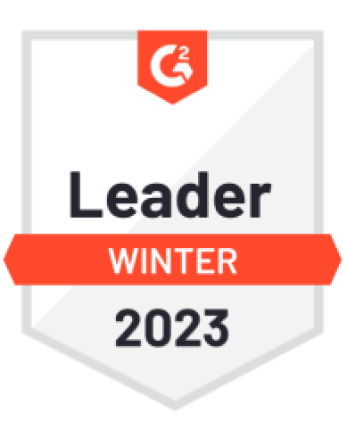
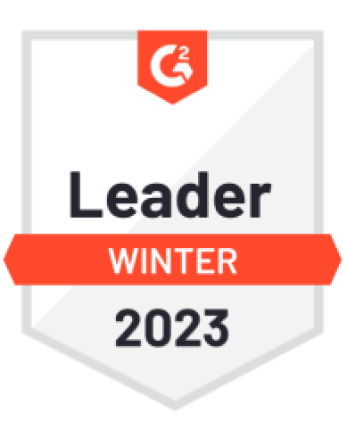

“[I like] The easy to use interface and the speed of finding the relevant assets that you're looking for in your database. I also really enjoy the score given to each table, [which] lets you prioritize the results of your queries by how often certain data is used.” - Michal P., Head of Data