Predictive Data Quality: What is It & How to Go About It
Learn all about predictive data quality and how to implement it in your business.
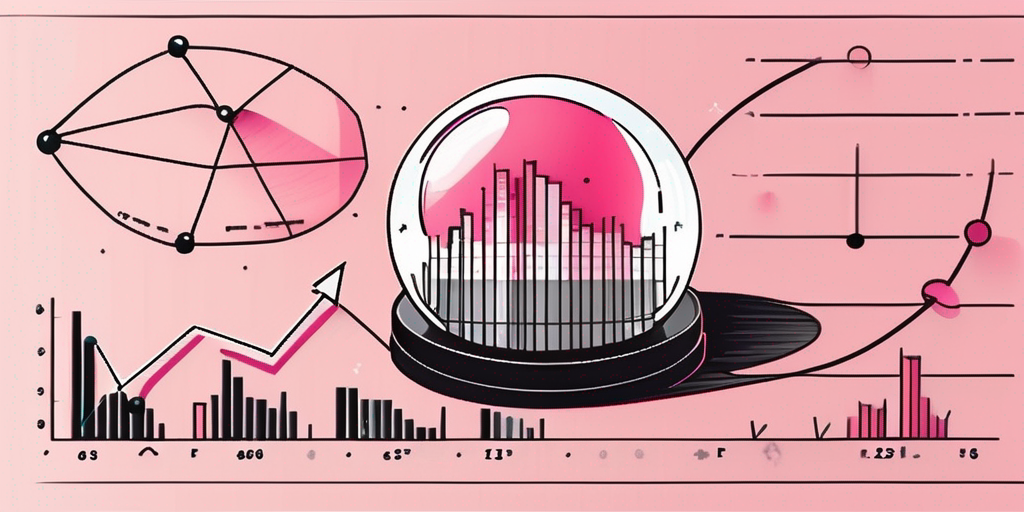
In the era of big data, the quality of data has become a critical factor in determining the success of any data-driven initiative. Predictive data quality is a proactive approach to ensuring the accuracy, consistency, and reliability of data. This article delves into the concept of predictive data quality, its importance, and how businesses can implement it effectively.
Understanding Predictive Data Quality
Predictive data quality refers to the use of advanced algorithms and statistical techniques to predict the quality of data. It is a proactive approach to data quality management that aims to identify potential issues before they become problematic. This approach is particularly useful in the context of big data, where the volume, velocity, and variety of data can make traditional data quality management techniques ineffective.
The concept of predictive data quality is rooted in the idea that data quality is not a static attribute, but rather a dynamic one that can change over time. By predicting the future quality of data, businesses can take proactive measures to ensure that their data remains accurate, consistent, and reliable.
The Importance of Predictive Data Quality
Predictive data quality is crucial in today's data-driven business environment for several reasons. First, it helps businesses maintain the accuracy and reliability of their data, which is essential for making informed decisions. Inaccurate or unreliable data can lead to poor decision-making, which can have serious consequences for a business.
Second, predictive data quality can help businesses save time and resources. By identifying potential data quality issues before they become problematic, businesses can avoid the costly and time-consuming process of correcting these issues after the fact.
Finally, predictive data quality can help businesses gain a competitive edge. By ensuring the quality of their data, businesses can gain more accurate insights from their data, which can help them make more informed decisions and stay ahead of their competitors.
Implementing Predictive Data Quality
Implementing predictive data quality requires a combination of advanced technologies and strategic planning. Here are some steps that businesses can take to implement predictive data quality effectively:
1. Understand Your Data
The first step in implementing predictive data quality is to understand your data. This involves identifying the types of data you have, where it comes from, how it is used, and how it changes over time. Understanding your data can help you identify potential data quality issues and determine the best ways to address them.
It's also important to understand the business context of your data. This involves understanding how your data is used in your business operations and decision-making processes. Understanding the business context of your data can help you prioritize your data quality efforts and focus on the data that is most important to your business.
2. Use Advanced Technologies
Predictive data quality requires the use of advanced technologies, such as machine learning and artificial intelligence. These technologies can help you analyze your data, identify patterns and trends, and predict future data quality issues.
Machine learning algorithms, for example, can be trained to recognize patterns in your data that may indicate potential data quality issues. Once these patterns are identified, you can take proactive measures to address these issues before they become problematic.
3. Implement Data Quality Management Practices
Implementing predictive data quality also requires the implementation of data quality management practices. These practices can help you maintain the quality of your data over time.
Some common data quality management practices include data cleansing, data profiling, and data governance. Data cleansing involves removing or correcting inaccurate or inconsistent data. Data profiling involves analyzing your data to identify patterns and trends. Data governance involves establishing policies and procedures for managing your data.
4. Monitor and Improve
Finally, implementing predictive data quality requires ongoing monitoring and improvement. This involves regularly monitoring your data quality, identifying areas for improvement, and taking action to improve your data quality.
Monitoring your data quality can involve using data quality metrics, such as accuracy, completeness, and consistency. These metrics can help you measure your data quality and track your progress over time. Improving your data quality can involve implementing new technologies or practices, or refining your existing ones.
Conclusion
In conclusion, predictive data quality is a proactive approach to data quality management that can help businesses maintain the accuracy and reliability of their data, save time and resources, and gain a competitive edge. Implementing predictive data quality requires understanding your data, using advanced technologies, implementing data quality management practices, and ongoing monitoring and improvement.
As the volume, velocity, and variety of data continue to increase, the importance of predictive data quality will only continue to grow. Businesses that can effectively implement predictive data quality will be well-positioned to make the most of their data and stay ahead of their competitors.
You might also like
Get in Touch to Learn More
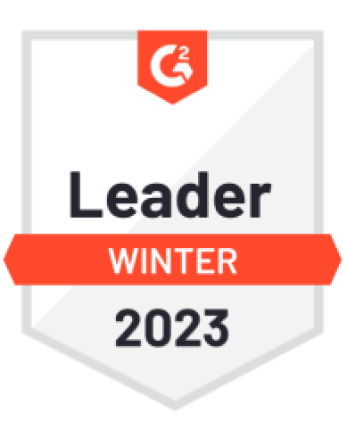
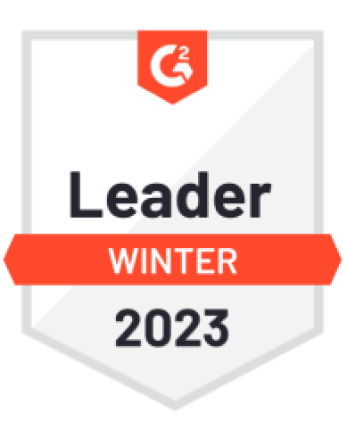

“[I like] The easy to use interface and the speed of finding the relevant assets that you're looking for in your database. I also really enjoy the score given to each table, [which] lets you prioritize the results of your queries by how often certain data is used.” - Michal P., Head of Data