Understanding the Data Governance Pillars
Discover the key pillars of data governance and gain a deeper understanding of how they form the foundation for effective data management.
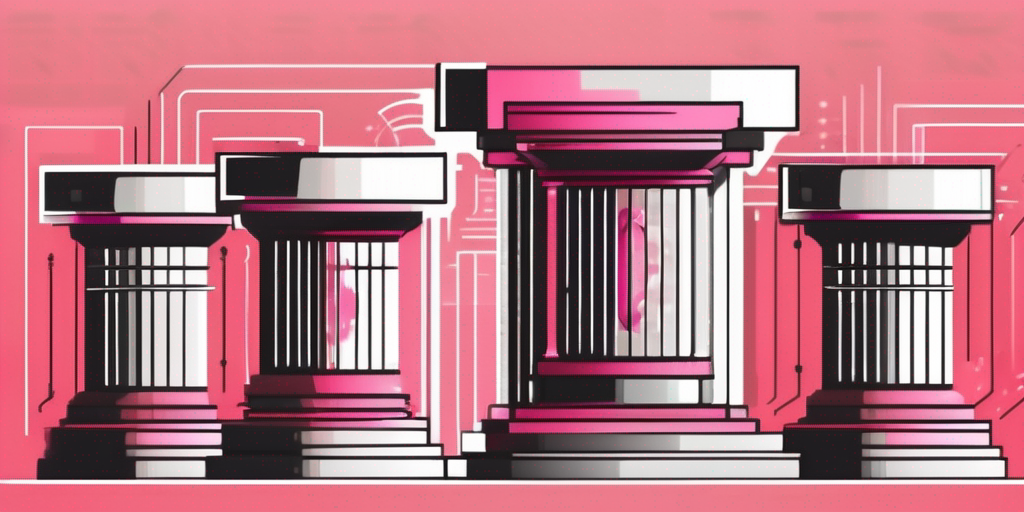
Data governance plays a critical role in organizations today, ensuring the effective management, protection, and utilization of data. By establishing a strong foundation for data governance, businesses can harness the full potential of their data assets and drive strategic decision-making. In this article, we will delve into the key pillars of data governance, exploring their significance, components, and implementation challenges.
Defining Data Governance
Data governance refers to the overall management of the availability, integrity, usability, and security of data within an organization. It involves the development and enforcement of policies, standards, and procedures to ensure the effective and efficient use of data assets. By establishing data governance practices, businesses can enhance data quality, ensure regulatory compliance, and drive data-driven decision-making.
The Importance of Data Governance
Data governance is crucial for organizations in today's data-driven world. It helps businesses build trust in their data, ensuring that it is accurate, reliable, and accessible when needed. By establishing data governance practices, organizations can make informed decisions based on reliable data, leading to improved operational efficiency and competitive advantage.
Key Components of Data Governance
Data governance consists of several key components that work together to ensure the effective management of data. These components include:
- Data Quality Management: This component focuses on ensuring the accuracy, completeness, consistency, and timeliness of data. It involves data profiling, validation, and cleansing activities to maintain data integrity.
- Data Security and Privacy: Data governance addresses the protection of sensitive data, ensuring compliance with data protection regulations and industry standards. It involves implementing access controls, encryption, and data masking techniques to safeguard data.
- Data Architecture and Integration: This component focuses on designing and implementing the data infrastructure, including data models, databases, and integration processes. It ensures data consistency, integration, and interoperability across different systems and platforms.
- Data Operations Management: Data governance encompasses the operational aspects of data management, including data provisioning, data lifecycle management, and data governance workflows. It ensures that data operations are efficient, reliable, and aligned with business objectives.
Another important component of data governance is Metadata Management. Metadata refers to the information about data, such as its source, format, and meaning. Metadata management involves capturing, organizing, and maintaining metadata to facilitate data discovery, understanding, and usage. It helps users find the right data, understand its context, and make informed decisions based on accurate information.
Furthermore, data governance also includes the component of Data Stewardship. Data stewards are responsible for overseeing the implementation and enforcement of data governance policies and procedures. They collaborate with various stakeholders to define data requirements, resolve data-related issues, and ensure compliance with data governance standards. Data stewards play a crucial role in promoting data accountability, driving data quality improvement initiatives, and fostering a data-driven culture within the organization.
The Pillars of Data Governance
Within data governance, there are four key pillars that form the foundation of a successful data governance program. These pillars encompass the essential aspects of data governance and guide organizations in establishing robust data management practices. Let's take a closer look at each of these pillars and explore the intricate details that make them crucial for any data-driven organization.
Data Quality Management
Data quality management is vital for organizations to ensure the accuracy, completeness, and consistency of their data. It involves a series of processes and techniques that allow businesses to identify and rectify data issues, leading to improved decision-making, operational efficiency, and customer satisfaction. Through data profiling, organizations can gain insights into the quality of their data, identifying anomalies and inconsistencies that may impact business processes. Data cleansing techniques, such as removing duplicate records or correcting inaccuracies, help maintain data integrity. Additionally, data validation processes ensure that data meets predefined quality standards, enabling organizations to rely on accurate and reliable information for critical operations.
Furthermore, data quality management is an ongoing effort that requires continuous monitoring and improvement. By implementing data governance frameworks and establishing data quality metrics, organizations can measure the effectiveness of their data quality management practices. This allows them to identify areas for improvement and implement corrective measures, ensuring that data remains a valuable asset for the organization.
Data Security and Privacy
With the increasing prevalence of data breaches and privacy concerns, data security and privacy have become critical components of data governance. Organizations must implement appropriate security controls, access management policies, and data protection mechanisms to safeguard sensitive data, maintain compliance with data privacy regulations, and preserve customer trust.
Data security measures include encryption, authentication, and authorization mechanisms that protect data from unauthorized access or malicious activities. Access management policies ensure that only authorized individuals can access and modify data, preventing unauthorized changes or data breaches. Additionally, organizations must implement data protection mechanisms, such as data masking or anonymization, to ensure that personally identifiable information (PII) is not exposed to unauthorized parties.
Compliance with data privacy regulations, such as the General Data Protection Regulation (GDPR) or the California Consumer Privacy Act (CCPA), is crucial for organizations operating in today's data-driven landscape. By adhering to these regulations, organizations demonstrate their commitment to protecting individuals' privacy rights and avoiding potential legal and financial consequences.
Data Architecture and Integration
Efficient and effective data architecture and integration are essential for organizations to ensure data consistency, interoperability, and scalability. Data architecture refers to the design and structure of an organization's data assets, including data models, data storage, and data flow. By implementing robust data models, organizations can define the structure and relationships of their data, ensuring consistency and accuracy across different systems and applications.
Data integration, on the other hand, focuses on the seamless exchange and utilization of data across various systems and applications. By standardizing data formats and employing integration technologies, organizations can overcome the challenges of data silos and enable accurate and timely decision-making. Integration technologies, such as extract, transform, load (ETL) processes or application programming interfaces (APIs), facilitate the movement and transformation of data, ensuring that it is available to the right people at the right time.
Moreover, data architecture and integration play a crucial role in enabling scalability and adaptability. As organizations grow and evolve, their data needs change. A well-designed data architecture and integration strategy allow organizations to accommodate new data sources, technologies, and business requirements, ensuring that data remains a valuable asset throughout the organization's journey.
Data Operations Management
Data operations management encompasses the day-to-day operational aspects of data governance, including data provisioning, data stewardship, and data governance workflows. It involves establishing clear roles, responsibilities, and processes to streamline data operations, facilitate data governance activities, and ensure the ongoing management and utilization of data assets.
Data provisioning refers to the process of making data available to users or applications in a timely and efficient manner. This involves data acquisition, data storage, and data delivery mechanisms that ensure data is accessible to those who need it. By implementing data provisioning strategies, organizations can minimize data latency, improve data availability, and support real-time decision-making.
Data stewardship, on the other hand, focuses on the responsible management and oversight of data assets. Data stewards are individuals or teams responsible for ensuring data quality, enforcing data governance policies, and resolving data-related issues. They play a crucial role in maintaining data integrity, promoting data governance best practices, and serving as a bridge between business users and technical teams.
Lastly, data governance workflows define the processes and workflows that guide data governance activities within an organization. These workflows ensure that data governance policies and procedures are followed consistently, enabling organizations to maintain data quality, security, and privacy. By automating data governance workflows, organizations can streamline data operations, reduce manual efforts, and ensure compliance with data governance frameworks.
By focusing on these four pillars of data governance, organizations can establish a solid foundation for their data management practices. Each pillar plays a unique role in ensuring the accuracy, security, and usability of data, enabling organizations to leverage data as a strategic asset and drive business success.
The Role of Data Governance in Business
Data governance is not just a technical practice; it has a significant impact on business operations and outcomes. Let's explore three key ways in which data governance enhances business performance.
Enhancing Decision Making
By establishing robust data governance practices, organizations can ensure that decision-makers have access to accurate, reliable, and timely data. This data-driven decision-making enables organizations to make informed choices, mitigate risks, and seize opportunities, resulting in improved business performance and competitive advantage.
Ensuring Regulatory Compliance
Data governance helps organizations navigate the complex landscape of data protection and privacy regulations. By implementing data governance practices, organizations can establish controls, policies, and procedures that ensure compliance with relevant regulations, reducing the risk of penalties, reputational damage, and legal complications.
Improving Operational Efficiency
Efficient data governance practices streamline data operations, resulting in improved operational efficiency. By establishing clear data processes, roles, and responsibilities, organizations can minimize data redundancy, improve data quality, and optimize data utilization, leading to cost savings, process efficiencies, and enhanced productivity.
Implementing Data Governance
Implementing effective data governance requires careful planning, collaboration, and commitment. Let's explore the key steps involved in establishing a robust data governance framework.
Steps to Establish Data Governance
1. Define Data Governance Objectives: Identify the strategic goals and objectives that data governance should address, aligning them with the organization's overall strategy and priorities.
2. Establish Governance Structure: Formulate a governance structure that defines roles, responsibilities, and decision-making processes. Assign data stewards and establish governance committees to oversee data governance activities.
3. Develop Data Policies and Standards: Create data governance policies and standards that outline data usage, quality, security, and privacy requirements. Ensure that these policies align with industry best practices and regulatory requirements.
4. Implement Data Governance Processes: Develop and implement processes for data profiling, data quality management, data security, and data integration. Define data governance workflows and procedures to ensure consistency and efficiency.
5. Educate and Train Data Stewards: Provide training and ongoing education to data stewards and other stakeholders involved in data governance. Ensure that they understand their roles, responsibilities, and the importance of data governance.
6. Monitor and Measure Data Governance: Establish metrics and key performance indicators (KPIs) to measure the effectiveness and impact of data governance. Continuously monitor data governance activities and adjust processes as needed.
Overcoming Challenges in Data Governance Implementation
Implementing data governance can be a complex endeavor, and organizations often face challenges along the way. Some common challenges include:
- Lack of Leadership and Sponsorship: Data governance requires strong leadership and support from senior management. Lack of executive sponsorship can hinder the successful implementation of data governance initiatives.
- Data Silos and Fragmented Systems: Data governance efforts are often hindered by data silos and fragmented systems. It is essential to establish data integration mechanisms and break down organizational silos to enable effective data governance.
- Resistance to Change: Data governance involves changes in processes, roles, and responsibilities, which may face resistance from employees. Effective change management strategies and communication plans can help overcome resistance and foster a positive data governance culture.
By anticipating and addressing these challenges, organizations can overcome obstacles and establish a strong data governance framework that drives data-driven decision-making and unlocks the full potential of their data assets.
In conclusion, understanding the pillars of data governance is crucial for organizations seeking to harness the power of their data. By prioritizing data quality management, data security and privacy, data architecture and integration, and data operations management, businesses can establish a strong foundation for effective data governance. Through the role of data governance in business, organizations can enhance decision-making, ensure regulatory compliance, and improve operational efficiencies. By following the steps to implement data governance and overcoming implementation challenges, businesses can unlock the true value of their data and gain a competitive edge in today's data-driven world.You might also like
Get in Touch to Learn More
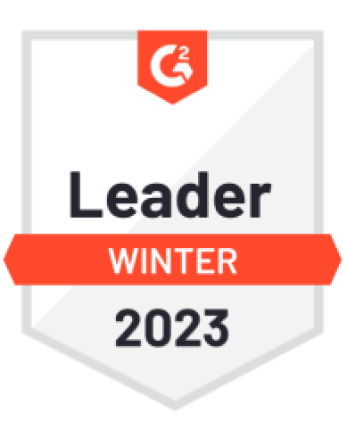
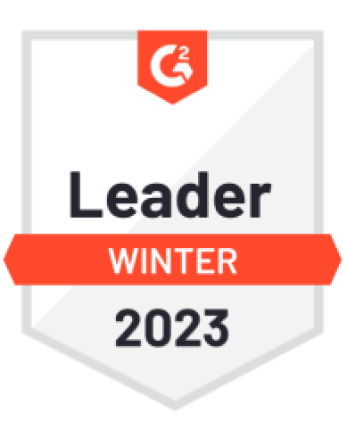

“[I like] The easy to use interface and the speed of finding the relevant assets that you're looking for in your database. I also really enjoy the score given to each table, [which] lets you prioritize the results of your queries by how often certain data is used.” - Michal P., Head of Data