What is Data Validity?
Discover the significance of data validity and how it impacts the accuracy and reliability of your information.

Data validity is a fundamental aspect of any research or data analysis. It refers to the accuracy and reliability of data, ensuring that the measurements and observations are trustworthy, consistent, and unbiased. By establishing data validity, researchers can confidently draw conclusions and make informed decisions based on the collected information. In this article, we will delve into the concept of data validity, its types, factors affecting it, strategies to enhance it, and its role in decision making.
Understanding the Concept of Data Validity
Data validity is a multifaceted concept that encompasses various dimensions. At its core, it is concerned with the extent to which collected data accurately reflects the targeted phenomenon or research question. To gain a deeper understanding of data validity, it is crucial to explore its definition and importance and distinguish it from data reliability.
Definition and Importance of Data Validity
Data validity can be defined as the extent to which a measurement or observation accurately represents the intended concept or construct. It ensures that the collected data is relevant, meaningful, and applicable to the research objectives and questions at hand. Valid data provides a solid foundation for drawing accurate conclusions, making informed decisions, and developing effective strategies.
When we talk about data validity, we are essentially discussing the quality of the data. It is like the backbone of any research or analysis. Just as a sturdy backbone supports the body, valid data supports the validity of any findings or recommendations derived from it. Without valid data, the entire research process can be compromised, leading to flawed conclusions and ineffective decision-making.
Moreover, data validity is not just important for researchers and analysts; it is equally crucial for decision-makers and stakeholders. Whether it is a business executive making strategic decisions or a policymaker formulating public policies, the accuracy and validity of the data they rely on are paramount. Valid data provides a reliable basis for decision-making, reducing the risk of making erroneous choices that may have adverse consequences.
Differentiating Data Validity from Data Reliability
While data validity and data reliability are closely related, they represent distinct concepts. While validity focuses on the accuracy and relevance of the measurements or observations, reliability concerns the consistency and stability of the measurements or observations over time or across different conditions.
Let's take an example to understand the difference between data validity and data reliability. Imagine you are conducting a survey to measure customer satisfaction with a product. Data validity would ensure that the questions in the survey accurately capture the different aspects of customer satisfaction, such as product quality, customer service, and value for money. On the other hand, data reliability would ensure that if the same survey is administered to the same group of customers at different times, it would yield consistent results.
In essence, data validity deals with whether the data truly represents the intended concept, while data reliability addresses the consistency and reproducibility of the data. Both aspects are essential for generating trustworthy and meaningful results. Without data validity, the findings may be inaccurate or irrelevant, while without data reliability, the findings may lack consistency and stability.
Therefore, researchers and analysts must pay attention to both data validity and data reliability to ensure the integrity and credibility of their work. By doing so, they can produce high-quality research that contributes to the advancement of knowledge and facilitates informed decision-making.
Types of Data Validity
Data validity is not an all-encompassing quality; it can manifest itself in different forms. Understanding the different types of data validity is crucial for researchers to choose appropriate methods and techniques to ensure the accuracy and relevance of their data. Some commonly recognized types of data validity include content validity, criterion-related validity, and construct validity.
Content Validity
Content validity refers to the extent to which the items or elements included in a measurement or assessment instrument adequately represent the targeted construct or domain. It entails examining whether the items adequately cover all aspects and dimensions of the concept being measured.
Ensuring content validity requires developing comprehensive measurement tools or assessment instruments that include items that represent the full range of the targeted construct. Expert judgment and consultation with domain experts play a crucial role in establishing content validity.
Criterion-Related Validity
Criterion-related validity assesses the extent to which a measurement or assessment instrument correlates with a criterion of interest to validate its use as a predictive or concurrent measure. In other words, it determines whether the measurements or observations accurately predict future outcomes or correspond to an existing criterion.
Criterion-related validity can be established through statistical techniques such as correlation analysis, regression analysis, or by comparing two measurement tools in terms of their ability to predict or identify a certain outcome.
Construct Validity
Construct validity focuses on the extent to which a measurement or assessment instrument effectively measures the theoretical construct it intends to quantify. It examines whether the measured variables are indeed capturing the underlying dimensions or theoretical concepts.
Establishing construct validity often involves testing hypotheses and conducting statistical analyses to assess the relationship between the measured variables and the theoretical construct. It could also include conducting factor analysis or confirmatory factor analysis to confirm the structural validity of a measurement instrument.
Factors Affecting Data Validity
Data validity can be influenced by various factors that researchers need to be aware of and account for during the research process. Factors such as data collection methods, sampling techniques, and measurement errors can significantly impact the validity of the collected data.
Data Collection Methods
The choice of data collection methods can affect data validity. Different methods, such as surveys, interviews, or observations, may yield different levels of accuracy, depending on the nature of the research question and the target population.
It is essential for researchers to carefully select and design data collection methods that align with the research objectives and minimize potential biases or measurement errors. By employing rigorous data collection techniques, researchers can enhance the validity of their findings.
Sampling Techniques
The manner in which the sample is selected can also have implications for data validity. Inappropriately selecting or biasing the sample can result in findings that are not representative of the target population, leading to invalid conclusions or generalizations.
To ensure data validity, researchers must employ appropriate sampling techniques such as random sampling or stratified sampling, which help to reduce bias and increase the likelihood of obtaining a representative sample.
Measurement Errors
Measurement errors can introduce distortions and inaccuracies in data, compromising its validity. Common sources of measurement errors include equipment malfunction, human error during data collection, or improper measurement procedures.
Researchers need to carefully design measurement instruments, train data collectors, and implement quality control measures to minimize measurement errors and enhance data validity. Regular calibration and verification of measurement equipment can also contribute to accurate and reliable data collection.
Ensuring Data Validity in Research
Ensuring data validity requires a comprehensive approach that involves implementing various strategies and best practices. By employing these strategies, researchers can enhance the quality and reliability of their data.
Strategies for Enhancing Data Validity
One effective strategy is to employ multiple sources of data. Triangulating information from different sources or using different data collection methods can increase confidence in the findings and reduce the risk of relying on flawed measurements or observations.
Another strategy is to use standardized measurement instruments or assessment tools that have been rigorously developed and validated. These tools have undergone extensive testing and are designed to effectively and accurately measure the intended constructs.
Common Pitfalls and How to Avoid Them
Researchers should be aware of common pitfalls that can compromise data validity and take measures to mitigate them. Some common pitfalls include social desirability bias, response bias, and non-response bias.
To combat these biases, researchers can ensure anonymity and confidentiality in data collection, use neutral and unbiased language in questionnaires or interviews, and employ rigorous data cleaning and validation procedures.
The Role of Data Validity in Decision Making
Data validity plays a crucial role in decision making across various domains, including business and policy-making. It serves as a foundation for sound decision making by providing reliable and meaningful information.
Impact on Business Decisions
In the business world, data validity is essential for making informed decisions regarding product development, marketing strategies, customer segmentation, and financial forecasting, among others. Accurate and valid data enables businesses to identify trends, evaluate the effectiveness of strategies, and make precise projections, leading to improved business performance.
Influence on Policy Making
Data validity is equally critical in the realm of policy making. Policymakers rely on accurate and valid data to identify societal issues, assess the impact of existing policies, and develop effective solutions. Valid data supports evidence-based policy making, ensuring that decisions are grounded in reliable information and have the potential to bring about positive social change.
Conclusion
Data validity is a cornerstone of research and analysis. It ensures that measurements and observations accurately reflect the intended concepts or constructs, allowing researchers to draw trustworthy conclusions and make informed decisions. By understanding the concept of data validity, its types, and the factors that can affect it, researchers can employ strategies to enhance data validity and leverage its role in decision making. Emphasizing data validity not only improves the quality of research but also contributes to more robust and reliable outcomes in various domains.
You might also like
Get in Touch to Learn More
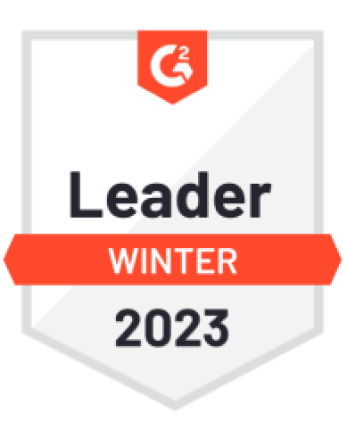
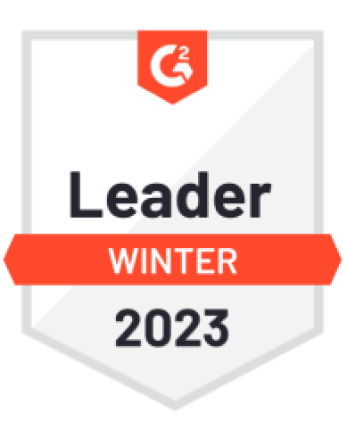

“[I like] The easy to use interface and the speed of finding the relevant assets that you're looking for in your database. I also really enjoy the score given to each table, [which] lets you prioritize the results of your queries by how often certain data is used.” - Michal P., Head of Data