Data Observability Tool Comparison: Bigeye vs. Monte Carlo
In the fast-paced and data-driven world we live in, ensuring the quality and reliability of our data has become paramount. Data observability has emerged as a critical practice for organizations to monitor and maintain the integrity of their data. In this article, we will delve into the comparison between two leading data observability tools: Bigeye and Monte Carlo. Understanding the importance and key features of data observability will set the stage for our in-depth exploration of these two tools and their functionalities. We will then evaluate their pros and cons before concluding with a pricing analysis.
Understanding Data Observability
Data observability refers to the ability to effortlessly monitor, measure, and analyze the quality and reliability of data. It goes beyond traditional data quality practices by focusing on the continuous monitoring and proactive identification of data issues. Data observability ensures that data pipelines are functioning properly, data quality is maintained, and any anomalies or inconsistencies are immediately addressed.
But what exactly makes data observability so important in today's data-driven world? Well, with the rapidly increasing volume, variety, and velocity of data, organizations cannot afford to overlook data observability. By ensuring the quality and reliability of their data, businesses can make informed decisions, optimize processes, and avoid costly errors. Data observability empowers data teams to identify and resolve issues in real-time, reducing the risk of data-driven decisions being made on inaccurate or incomplete information.
Now, let's take a closer look at some of the key features offered by data observability tools. These features are designed to facilitate the monitoring and analysis of data, ensuring that organizations have full visibility into the health and performance of their data pipelines.
The Importance of Data Observability
With the rapidly increasing volume, variety, and velocity of data, organizations cannot afford to overlook data observability. By ensuring the quality and reliability of their data, businesses can make informed decisions, optimize processes, and avoid costly errors. Data observability empowers data teams to identify and resolve issues in real-time, reducing the risk of data-driven decisions being made on inaccurate or incomplete information.
Key Features of Data Observability Tools
Data observability tools offer a range of features to facilitate the monitoring and analysis of data. These include:
- Real-time Monitoring: Data observability tools enable continuous monitoring of data pipelines, ensuring that incoming data is accurate and reliable. This real-time monitoring capability allows organizations to quickly detect and address any issues that may arise, minimizing the impact on data quality and reliability.
- Anomaly Detection: These tools utilize advanced algorithms to detect anomalies and inconsistencies in the data, alerting data teams to potential problems. By automatically identifying and flagging unusual patterns or outliers, data observability tools help organizations proactively address data issues before they escalate.
- Data Profiling: Data observability tools provide insights into the quality and structure of the data, helping data teams identify data issues and ensure compliance with data standards. With data profiling, organizations can gain a comprehensive understanding of their data, including its completeness, accuracy, and consistency.
By leveraging these key features, data observability tools empower organizations to maintain the highest standards of data quality and reliability. They enable data teams to monitor data pipelines in real-time, detect anomalies, and gain valuable insights into the health and performance of their data. With data observability, organizations can confidently rely on their data to drive business decisions and achieve their goals.
An Introduction to Bigeye
Bigeye is a comprehensive data observability tool that helps organizations ensure the reliability and quality of their data pipelines. Let's take a closer look at its functionality.
Bigeye provides an intuitive and user-friendly interface for managing and monitoring data pipelines. Its key features include:
- Visual Data Lineage: Bigeye offers a visual representation of data lineage, allowing data teams to easily track the flow and transformation of data throughout its lifecycle.
- Anomaly Detection: Bigeye's advanced algorithms detect anomalies and outliers, enabling data teams to quickly identify and troubleshoot issues.
- Automated Data Quality Checks: This tool automates data quality checks, ensuring that data meets predefined standards and alerting teams to any deviations.
In addition to these core features, Bigeye goes above and beyond to provide a comprehensive data observability experience. It offers a range of advanced functionalities that empower organizations to gain deeper insights into their data pipelines.
One such functionality is the ability to create custom alerts and notifications. Bigeye allows users to set up alerts based on specific data conditions or thresholds. This means that data teams can be instantly notified when certain events occur, such as a sudden drop in data quality or a significant increase in data processing time. These alerts help teams proactively address issues and ensure the smooth operation of their data pipelines.
Another noteworthy feature of Bigeye is its integration capabilities. It seamlessly integrates with popular data storage and processing platforms, such as Amazon S3, Google Cloud Storage, and Apache Kafka. This allows organizations to leverage their existing infrastructure and easily incorporate Bigeye into their data ecosystem. By integrating with these platforms, Bigeye can provide real-time monitoring and analysis, ensuring that data teams have up-to-date insights into the health and performance of their pipelines.
Pros and Cons of Bigeye
While Bigeye offers several benefits, it's important to consider its limitations. Let's explore the pros and cons.
Pros:
- User-friendly interface, making it accessible for both technical and non-technical users.
- Robust anomaly detection capabilities, enabling prompt identification of data issues.
- Automated data quality checks streamline monitoring processes and ensure compliance.
Cons:
- Limited scalability for handling large volumes of data.
- Relatively higher pricing compared to other data observability tools.
- Less flexibility in customization options for specific data monitoring needs.
Despite these limitations, Bigeye remains a powerful tool for organizations looking to ensure the reliability and quality of their data pipelines. Its comprehensive functionality, user-friendly interface, and advanced features make it a valuable asset for data teams across industries.
An Introduction to Monte Carlo
Monte Carlo is another prominent data observability tool that focuses on data reliability and quality assurance. Let's delve into its functionality.
Overview of Monte Carlo's Functionality
Monte Carlo provides organizations with actionable insights into their data pipelines, ensuring data integrity and reliability. Its key features encompass:
- Data Monitoring: Monte Carlo continuously monitors data pipelines, providing real-time visibility into the health and quality of data.
- Data Testing: This tool enables data teams to define and execute tests to validate the accuracy and reliability of the data.
- Root Cause Analysis: Monte Carlo's root cause analysis capabilities help identify the source of data issues, enabling efficient troubleshooting and resolution.
Pros and Cons of Monte Carlo
Let's explore the advantages and drawbacks of utilizing Monte Carlo for data observability.
Pros:
- Comprehensive data monitoring capabilities, enabling proactive identification of data issues.
- Flexible and customizable testing framework to validate data accuracy and reliability.
- Advanced root cause analysis aids in swift resolution of data-related problems.
Cons:
- Steep learning curve for users unfamiliar with data observability concepts.
- Relatively limited integration options with other data tools.
- Potential performance issues when dealing with exceptionally large datasets.
Detailed Comparison Between Bigeye and Monte Carlo
Comparing User Interface and Ease of Use
When it comes to user interface and ease of use, both Bigeye and Monte Carlo offer intuitive and user-friendly platforms. Bigeye's visually appealing interface provides a clear understanding of data lineage, making it accessible for technical and non-technical users alike. On the other hand, Monte Carlo may have a steeper learning curve due to its extensive features, but it offers flexibility and customization options to meet specific data monitoring needs.
Comparing Data Accuracy and Reliability
Both Bigeye and Monte Carlo excel in ensuring data accuracy and reliability. Bigeye's anomaly detection capabilities promptly identify data issues, enabling data teams to take immediate action. Monte Carlo's comprehensive data testing framework allows users to define and execute tests to validate the accuracy and reliability of the data, providing robust data quality control.
Comparing Scalability and Performance
When it comes to scalability and performance, Bigeye may face limitations in handling exceptionally large volumes of data. On the other hand, Monte Carlo's strong data monitoring capabilities ensure smooth performance, even with large datasets. However, both tools require proper configuration and optimization to maintain optimal efficiency.
Pricing Analysis: Bigeye vs. Monte Carlo
Understanding Bigeye's Pricing Structure
Bigeye offers various pricing plans based on the organization's needs. The pricing structure typically includes factors such as data volume, number of data sources, and additional features required. Interested users can reach out to Bigeye's sales team to obtain detailed pricing information tailored to their specific requirements.
Understanding Monte Carlo's Pricing Structure
Monte Carlo also offers flexible pricing plans based on the organization's needs and requirements. The pricing structure encompasses factors such as the number of data sources, volume of data, and additional features required. Organizations are encouraged to reach out to Monte Carlo's sales team to receive personalized pricing details.
In conclusion, both Bigeye and Monte Carlo provide robust data observability solutions, ensuring the quality and reliability of data. While Bigeye excels in user-friendliness and automated data quality checks, Monte Carlo offers comprehensive data testing capabilities and efficient root cause analysis. The choice between the two depends on the organization's specific needs, scalability requirements, and budget considerations. By carefully evaluating their functionalities, pros and cons, and pricing structures, organizations can make an informed decision on which data observability tool best aligns with their objectives.
As you consider the right data observability tool for your organization, remember that the journey doesn't end there. CastorDoc offers a seamless extension to your data management capabilities, integrating advanced governance, cataloging, and lineage features with a user-friendly AI assistant. This powerful combination enables self-service analytics and empowers your team to harness the full potential of your data. Whether you're looking to maintain data quality, ensure compliance, or enable business users to find and understand data with ease, CastorDoc is your partner in revolutionizing data governance and accessibility. Ready to explore how CastorDoc can complement tools like Bigeye and Monte Carlo? Check out more tools comparisons here and take the first step towards a more informed and data-driven future.
You might also like
Get in Touch to Learn More
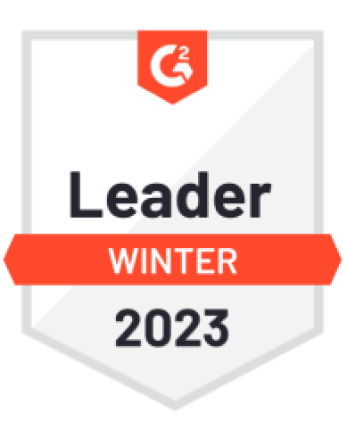
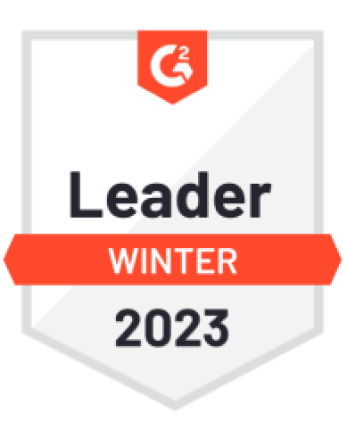

“[I like] The easy to use interface and the speed of finding the relevant assets that you're looking for in your database. I also really enjoy the score given to each table, [which] lets you prioritize the results of your queries by how often certain data is used.” - Michal P., Head of Data