Data Observability Tool Comparison: Lantern vs. Metaplane
In today's data-driven world, organizations rely heavily on data observability tools to ensure the quality, reliability, and availability of their data. Two popular options in the market are Lantern and Metaplane. In this article, we will compare and contrast these two tools to help you make an informed decision for your data observability needs.
Understanding Data Observability Tools
To comprehend the importance of Lantern and Metaplane, it is essential to understand the role that data observability tools play in an organization's data infrastructure. These tools are designed to provide visibility into data pipelines, ensuring that data flows smoothly from its source to its destination.
Implementing data observability tools goes beyond just monitoring data pipelines; it involves creating a culture of data transparency and accountability within an organization. By utilizing these tools, companies can foster a data-driven decision-making process that is rooted in trust and reliability.
The Role of Data Observability Tools
Data observability tools act as a safeguard for data quality, identifying and alerting on data anomalies, inconsistencies, and data quality issues. They enable data teams to proactively monitor their data pipelines, minimizing data downtime and ensuring accurate decision-making based on reliable data.
Moreover, data observability tools play a crucial role in ensuring compliance with data regulations and standards. By continuously monitoring data quality and lineage, organizations can easily demonstrate data compliance to regulatory bodies and maintain the trust of their customers.
Key Features of Data Observability Tools
Data observability tools typically offer a range of features designed to enhance data reliability and monitoring. These features may include data lineage tracking, schema validation, automated anomaly detection, metadata management, and data profiling. By providing these capabilities, data observability tools empower organizations to maintain data integrity and drive better business outcomes.
Additionally, some advanced data observability tools leverage machine learning algorithms to predict potential data issues before they occur, allowing data teams to take proactive measures to prevent disruptions in data flow. This predictive capability not only improves operational efficiency but also enhances the overall data governance framework of an organization.
Introduction to Lantern
Lantern is a powerful data observability tool that offers a comprehensive suite of features to enable organizations to gain full visibility into their data pipelines. With its advanced monitoring and troubleshooting capabilities, Lantern empowers data teams to ensure the reliability and efficiency of their data processes.
By leveraging Lantern, organizations can proactively monitor their data pipelines in real-time, allowing them to detect and address any issues before they escalate. This proactive approach helps in maintaining data quality and integrity, ultimately leading to better decision-making based on accurate and up-to-date information.
Overview of Lantern's Functionality
Lantern provides a user-friendly interface that allows data teams to easily monitor and troubleshoot their data pipelines. It offers real-time data monitoring, anomaly detection, and alerts, enabling teams to quickly identify and resolve data issues. Additionally, Lantern provides detailed data lineage tracking, allowing users to trace the journey of data from its source to its destination. This comprehensive visibility into the data flow helps in ensuring data governance and compliance with regulatory requirements.
Moreover, Lantern's collaboration features enable seamless communication and knowledge sharing among team members. Users can annotate data anomalies, share insights, and collaborate on troubleshooting strategies within the platform, fostering a culture of teamwork and continuous improvement.
Pros and Cons of Using Lantern
One of the key benefits of using Lantern is its intuitive user interface, which makes it easy for both technical and non-technical users to navigate and understand. The real-time monitoring and alerting capabilities are also highly regarded, as they enable teams to take immediate action in response to data anomalies. However, some users have reported that Lantern's scalability can be a limitation for large-scale data operations. Despite this, Lantern remains a valuable tool for organizations looking to enhance their data observability and ensure the reliability of their data pipelines.
Introduction to Metaplane
Metaplane is another popular data observability tool that offers robust features to ensure data reliability and quality. With the increasing reliance on data-driven decision-making in today's business landscape, tools like Metaplane play a crucial role in helping organizations harness the power of their data effectively.
Designed to cater to the needs of data professionals, Metaplane provides a user-friendly interface coupled with advanced functionalities that streamline the data monitoring and management process.
Overview of Metaplane's Functionality
Metaplane provides a comprehensive set of tools for monitoring and managing data pipelines. It offers advanced anomaly detection algorithms, data lineage visualization, and a powerful query language that enables users to gain deep insights into their data. With Metaplane, data teams can easily track data quality, identify issues, and take corrective actions. The anomaly detection algorithms employed by Metaplane are designed to detect subtle deviations in data patterns, allowing users to proactively address potential issues before they escalate.
Moreover, the data lineage visualization feature in Metaplane offers a clear and intuitive way to trace the origins and transformations of data as it moves through various stages of processing. This visualization not only aids in understanding the flow of data but also helps in pinpointing bottlenecks or areas for optimization within the data pipeline.
Pros and Cons of Using Metaplane
Metaplane's strength lies in its powerful anomaly detection capabilities and its ability to handle large-scale data operations. Users appreciate the flexible query language, as it allows them to perform complex analyses and gain deeper insights into their data. However, some users have mentioned that the learning curve for using Metaplane can be steep, requiring a certain level of technical expertise. Despite this, the investment in mastering Metaplane's features is often rewarded with enhanced data reliability and operational efficiency.
Detailed Comparison Between Lantern and Metaplane
Now let's dive into a detailed comparison between Lantern and Metaplane, focusing on three key aspects: user interface and experience, data processing capabilities, and integration and compatibility.
Comparing User Interface and Experience
Lantern offers an intuitive and user-friendly interface, making it accessible to users with varying levels of technical expertise. Its visually appealing dashboard provides a clear overview of data pipelines and makes it easy to identify potential issues. The dashboard is customizable, allowing users to arrange and prioritize the information that is most relevant to them. Additionally, Lantern provides interactive visualizations that enable users to explore and analyze data in a more engaging way.
Metaplane, on the other hand, provides a more advanced interface that may require additional training to fully leverage its capabilities. It offers a comprehensive set of features and tools for data observability, allowing users to dive deep into the data and perform complex analysis. While the learning curve may be steeper, Metaplane's interface empowers users with advanced functionalities and customization options.
Comparing Data Processing Capabilities
Both Lantern and Metaplane offer robust data processing capabilities, allowing users to monitor and analyze data in real-time. Lantern's data processing engine is optimized for speed and efficiency, ensuring quick and accurate data processing. It leverages parallel processing techniques to handle large volumes of data without compromising performance.
However, Metaplane's advanced anomaly detection algorithms give it an edge in identifying complex patterns and anomalies. Its machine learning algorithms can detect subtle deviations from expected data patterns, enabling users to proactively identify and address potential issues. This capability is particularly valuable in industries where even minor anomalies can have significant consequences.
Lantern, on the other hand, excels in providing detailed data lineage tracking, allowing users to trace data transformations and identify potential bottlenecks. Its comprehensive lineage visualization helps users understand the flow of data across different stages of processing, facilitating troubleshooting and optimization.
Comparing Integration and Compatibility
Integration capabilities are crucial for data observability tools. Lantern offers seamless integration with popular data platforms and tools, ensuring compatibility with existing data ecosystems. It provides pre-built connectors for a wide range of data sources, making it easy to ingest data from various systems. Additionally, Lantern supports bi-directional integration, allowing users to not only monitor data but also take actions based on the insights gained.
Metaplane also provides strong integration capabilities and supports a wide range of data sources. It offers connectors for popular databases, cloud storage services, and streaming platforms. However, users have reported that configuring and maintaining integrations with Metaplane can be more complex compared to Lantern. The setup process may require additional technical expertise and involve more manual configuration steps.
In conclusion, both Lantern and Metaplane offer powerful features and capabilities for data observability. The choice between the two depends on the specific needs and preferences of the users. Lantern provides a user-friendly interface and comprehensive data lineage tracking, while Metaplane offers advanced anomaly detection algorithms and a more advanced interface. Consider your organization's requirements and the technical expertise of your team when making a decision.
Pricing Analysis
When considering data observability tools, pricing is an important factor to consider. Let's take a closer look at the pricing structures of both Lantern and Metaplane.
Lantern Pricing Structure
Lantern offers a flexible pricing structure based on the size and complexity of the data infrastructure. The pricing is tailored to meet the specific needs of organizations, ensuring they pay only for the features and resources they require. It is recommended to contact Lantern's sales team for a detailed quote based on your organization's requirements.
Metaplane Pricing Structure
Metaplane follows a similar pricing model to Lantern, offering customizable packages based on the organization's requirements. The pricing structure takes into account factors such as data volume, number of data sources, and additional features required. To get a precise quote, it is advised to reach out to Metaplane's sales team.
Conclusion
Choosing the right data observability tool for your organization is a critical decision. Both Lantern and Metaplane provide powerful features to ensure data reliability and quality, but each tool has its strengths and weaknesses. Consider your organization's specific requirements, the complexity of your data infrastructure, and the desired user experience when making your choice. Ultimately, the tool that best aligns with your organization's data observability goals will be the one that empowers your data teams to make accurate and timely decisions based on trustworthy data.
While Lantern and Metaplane offer valuable features for data observability, CastorDoc takes a comprehensive approach to data management that may further enhance your organization's ability to harness the power of data. With CastorDoc's advanced governance, cataloging, and lineage capabilities, paired with a user-friendly AI assistant, your data teams and business users can achieve self-service analytics with ease. Whether you're looking to maintain data quality, ensure compliance, or empower decision-making, CastorDoc provides a seamless solution. To explore how CastorDoc compares to other tools and to discover the ideal fit for your data infrastructure, check out more tools comparisons here.
You might also like
Get in Touch to Learn More
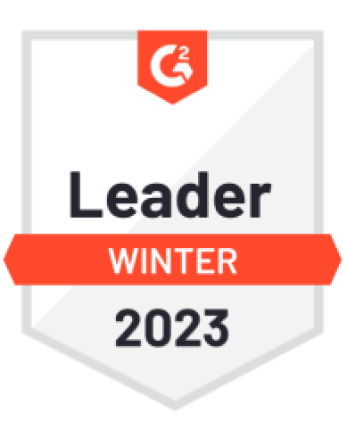
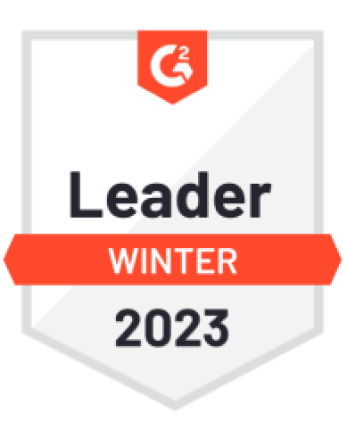

“[I like] The easy to use interface and the speed of finding the relevant assets that you're looking for in your database. I also really enjoy the score given to each table, [which] lets you prioritize the results of your queries by how often certain data is used.” - Michal P., Head of Data