Data Observability Tool Comparison: Lightup vs. Lantern
Data observability has become a critical requirement for organizations that rely on data-driven decision making. In order to ensure the accuracy, reliability, and completeness of their data, businesses are turning to data observability tools. Two popular options in the market are Lightup and Lantern. In this article, we will compare these two tools in terms of their functionality, pros and cons, user interface, data analysis capabilities, integration, compatibility, and pricing. By the end of this article, you will have a clear understanding of which tool is better suited for your data observability needs.
Understanding Data Observability
Data observability is the practice of monitoring, managing, and improving the quality of data within an organization. It involves ensuring that data is accurate, reliable, and consistent across various systems and pipelines. Data observability tools play a crucial role in enabling organizations to proactively identify and resolve issues related to data quality, data integrity, and data completeness.
Implementing data observability requires a comprehensive approach that involves not only technological solutions but also organizational processes and cultural shifts. Organizations need to establish clear data governance policies, define data quality standards, and foster a culture of data literacy among employees. By integrating data observability into the fabric of the organization, companies can ensure that data remains a reliable asset that drives informed decision-making.
The Importance of Data Observability
Data is the lifeblood of modern businesses. It drives strategic decision making, enhances operational efficiency, and enables organizations to gain a competitive edge. However, without proper observability, data can become unreliable and misleading, leading to incorrect insights and decisions. Data observability ensures the trustworthiness of data, providing organizations with the confidence to make data-driven decisions.
Furthermore, data observability is essential for regulatory compliance and risk management. In industries such as finance, healthcare, and e-commerce, where data privacy and security are paramount, maintaining a high level of data observability is crucial. By continuously monitoring data quality and integrity, organizations can mitigate the risks associated with data breaches, compliance violations, and reputational damage.
Key Features of Data Observability Tools
Data observability tools offer a wide range of features to help organizations monitor and improve the quality of their data. These features often include data profiling, anomaly detection, data lineage tracking, data monitoring, and alerting mechanisms. The ability to visualize and analyze data in real-time is also a crucial feature of data observability tools.
Additionally, some advanced data observability tools leverage machine learning and artificial intelligence algorithms to automate the detection of data anomalies and discrepancies. By harnessing the power of AI, organizations can enhance the efficiency and accuracy of their data monitoring processes, enabling them to detect and address issues in real-time. This proactive approach to data observability empowers organizations to maintain a high standard of data quality and integrity, ultimately driving better business outcomes.
An Introduction to Lightup
Lightup is a powerful data observability tool designed to help organizations ensure the quality of their data. It offers a comprehensive set of features that enable users to monitor data pipelines, detect anomalies, track data lineage, and visualize data in real-time. With its intuitive interface and user-friendly design, Lightup makes it easy for data teams to identify and resolve issues related to data quality and reliability.
When it comes to data observability, Lightup stands out as a reliable and efficient solution. By providing a centralized dashboard, Lightup allows users to have a holistic view of their data pipelines. This means that data teams can easily monitor the health of their pipelines, ensuring that data flows smoothly and without any disruptions. With Lightup, organizations can proactively detect and address any issues that may arise, minimizing the impact on data quality and overall business operations.
Overview of Lightup's Functionality
Lightup provides users with a centralized dashboard where they can monitor the health of their data pipelines. It offers advanced anomaly detection algorithms that automatically identify deviations from expected data patterns. This means that data teams can quickly identify any irregularities or discrepancies in their data, allowing them to take immediate action to rectify the situation.
In addition to anomaly detection, Lightup enables users to track data lineage, allowing them to trace the origin and transformation of data throughout the pipeline. This feature is particularly valuable for organizations that deal with complex data workflows, as it provides transparency and visibility into the entire data journey. By understanding the lineage of their data, data teams can confidently make decisions based on accurate and reliable information.
Furthermore, Lightup provides real-time data visualization capabilities, empowering users to gain valuable insights from their data. With its interactive charts, graphs, and visual representations, users can easily analyze and understand their data trends, patterns, and correlations. This not only enhances decision-making processes but also enables organizations to identify opportunities for optimization and improvement.
Pros and Cons of Using Lightup
One of the major advantages of using Lightup is its ease of use. The tool is highly intuitive, allowing users to quickly navigate through its features and functionalities. This means that data teams can easily adapt to Lightup, minimizing the learning curve and maximizing productivity. Additionally, Lightup offers a user-friendly design that enhances the overall user experience, making data observability a seamless and enjoyable process.
Another significant benefit of Lightup is its excellent visualization capabilities. The tool provides a wide range of visualization options, including charts, graphs, and dashboards, which enable users to easily analyze and understand their data. With visually appealing and interactive representations, data teams can identify trends, outliers, and patterns at a glance, facilitating data-driven decision-making processes.
However, it is important to note that Lightup has some limitations, one of which is its limited integration options. While Lightup supports popular data integration platforms, such as Apache Kafka and Amazon S3, it may require additional configuration for less common systems. This means that organizations using niche or custom-built data integration solutions may need to invest additional time and effort to integrate Lightup seamlessly into their existing infrastructure.
In conclusion, Lightup is a powerful data observability tool that offers a comprehensive set of features to ensure the quality and reliability of data. With its intuitive interface, advanced anomaly detection algorithms, data lineage tracking, and real-time data visualization capabilities, Lightup empowers data teams to proactively monitor, analyze, and optimize their data pipelines. While it excels in ease of use and visualization, organizations should consider their integration requirements before implementing Lightup.
An Introduction to Lantern
Lantern is another prominent data observability tool that helps organizations ensure the quality and reliability of their data. It offers a comprehensive suite of features that enable users to monitor data pipelines, detect anomalies, and ensure data integrity. With its robust capabilities, Lantern empowers organizations to gain valuable insights from their data and make informed decisions.
Overview of Lantern's Functionality
Lantern provides users with a wide range of monitoring and alerting mechanisms to ensure the quality of their data. It offers advanced anomaly detection algorithms that can detect and notify users of any unusual data patterns. Lantern also enables users to track data lineage and ensure the integrity of their data. Additionally, Lantern provides powerful data analytics tools that allow users to derive insights from their data.
Pros and Cons of Using Lantern
Lantern offers robust monitoring and alerting capabilities, making it easy for users to identify and resolve issues related to data quality. The tool also provides powerful data analytics tools, enabling users to derive meaningful insights from their data. However, one limitation of Lantern is its relatively steep learning curve. Users may require some time to fully grasp the tool's functionalities and get up to speed with its features.
In-Depth Comparison Between Lightup and Lantern
Now that we have looked at the individual features and functionalities of Lightup and Lantern, let's dive deeper into a head-to-head comparison between these two tools. We will be comparing them in terms of their user interface and experience, data analysis capabilities, and integration and compatibility.
Comparing User Interface and Experience
Both Lightup and Lantern offer user-friendly interfaces that are designed to make it easy for users to navigate through the tools' features and functionalities. Lightup's interface is known for its simplicity and intuitive design, whereas Lantern's interface offers more advanced customization options. The choice between the two will largely depend on the specific needs and preferences of the users.
Comparing Data Analysis Capabilities
When it comes to data analysis, both Lightup and Lantern provide powerful tools that enable users to gain insights from their data. Lightup offers real-time data visualization capabilities, making it easy for users to analyze and understand their data. Lantern, on the other hand, offers advanced data analytics features such as predictive modeling and machine learning algorithms, which can help organizations uncover hidden patterns and trends in their data.
Comparing Integration and Compatibility
Integration and compatibility are crucial factors to consider when choosing a data observability tool. Lightup supports a wide range of data integration platforms and can seamlessly integrate with existing data pipelines. Lantern also offers integration options with popular data platforms, although it may require additional configuration for certain systems. Organizations should carefully evaluate their existing data infrastructure before choosing between Lightup and Lantern.
Pricing Comparison
In addition to functionality and features, pricing is an important factor to consider when selecting a data observability tool. Let's explore the pricing structures of Lightup and Lantern.
Lightup's Pricing Structure
Lightup offers a flexible pricing structure that is based on the number of data sources and the volume of data processed. The tool provides different pricing tiers to accommodate the needs of organizations of all sizes. Lightup also offers a free trial period, allowing users to evaluate the tool's capabilities before making a long-term commitment.
Lantern's Pricing Structure
Lantern's pricing structure is also based on the number of data sources and the volume of data processed. The tool offers different pricing tiers to cater to the needs of organizations with varying data requirements. Lantern provides a free demo, giving users the opportunity to explore the tool and assess its suitability for their data observability needs.
Conclusion
In conclusion, both Lightup and Lantern are powerful data observability tools that offer a wide range of features and functionalities. Lightup is known for its ease of use and excellent visualization capabilities, while Lantern offers robust monitoring and alerting mechanisms. When choosing between these two tools, organizations should carefully consider their specific requirements, such as user interface preferences, data analysis needs, integration options, and pricing structures. Ultimately, the right tool will depend on the unique needs and goals of each organization.
Now you have a comprehensive understanding of the differences between Lightup and Lantern, allowing you to make an informed decision based on your specific data observability needs. Remember to thoroughly evaluate each tool's features, consider the pros and cons, and weigh the pricing structures before making a choice. Implementing the right data observability tool is crucial for ensuring the accuracy, reliability, and completeness of your data, which in turn will drive better decision making and propel your organization forward in the data-driven world.As you consider the best data observability tool for your organization, remember that the journey doesn't end there. With CastorDoc, you can take your data management to the next level by integrating advanced governance, cataloging, and lineage capabilities with the ease of a user-friendly AI assistant. CastorDoc is not just about maintaining data quality; it's about empowering your team to enable self-service analytics, ensuring compliance, and enhancing data accessibility through natural language interactions. Whether you're part of a data team looking for control and visibility or a business user seeking to harness data for strategic decisions, CastorDoc is your partner in navigating the complex data landscape. Ready to revolutionize how your organization leverages data? Check out more tools comparisons here and discover how CastorDoc can elevate your data governance and analytics capabilities.
You might also like
Get in Touch to Learn More
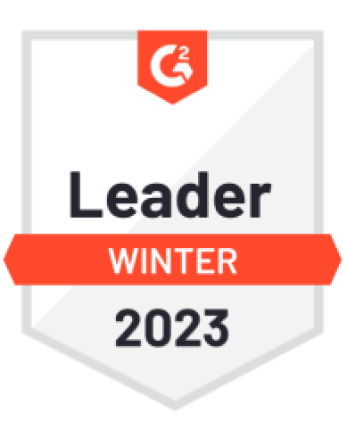
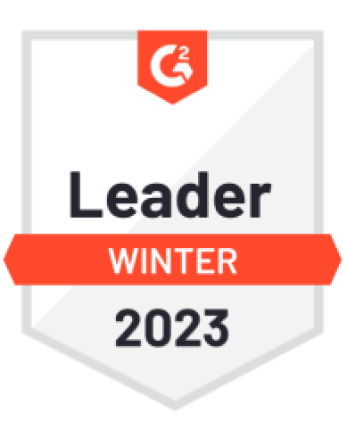

“[I like] The easy to use interface and the speed of finding the relevant assets that you're looking for in your database. I also really enjoy the score given to each table, [which] lets you prioritize the results of your queries by how often certain data is used.” - Michal P., Head of Data