Data Observability Tool Comparison: Monte Carlo vs. Acceldata
In today's data-driven world, the importance of maintaining data quality and reliability cannot be overstated. That's where data observability tools come into play. Among the top contenders in this space are Monte Carlo and Acceldata. In this article, we will delve deep into these two powerful tools, comparing their features, performance, and usability to help you make an informed decision about which one is the best fit for your organization.
Understanding Data Observability
Data observability refers to the practice of monitoring and ensuring the integrity, quality, and reliability of your data. It involves tracking the flow of data, identifying anomalies, and taking proactive measures to remediate any issues that arise. By implementing a robust data observability strategy, organizations can mitigate risks, improve decision-making, and maintain trust in their data-driven processes.
In addition to monitoring data flow and integrity, data observability also involves analyzing data usage patterns and trends. By understanding how data is being utilized within an organization, stakeholders can make informed decisions about resource allocation, system optimization, and future data strategies. This level of insight can lead to increased efficiency and innovation across all business functions.
Defining Data Observability
Data observability encompasses a range of practices and technologies aimed at delivering reliable and trustworthy data. It involves monitoring data pipelines, tracking data lineage, and ensuring data consistency at every stage. The goal is to have complete visibility into the health and integrity of your data, enabling you to identify and resolve any issues efficiently.
Furthermore, data observability plays a crucial role in regulatory compliance and risk management. By maintaining a high level of data observability, organizations can ensure that they are meeting data privacy regulations, industry standards, and internal governance policies. This not only helps in avoiding legal penalties but also builds a strong foundation of trust with customers and stakeholders.
Importance of Data Observability in Business
Data is now the lifeblood of modern businesses, driving critical processes across various industries. However, the sheer volume and complexity of data make it prone to errors, inconsistencies, and inaccuracies. This is where data observability becomes crucial. By proactively monitoring data quality, organizations can prevent costly mistakes, improve operational efficiency, and avoid reputation damage caused by decisions based on faulty data.
Moreover, data observability fosters a culture of data-driven decision-making within organizations. By providing real-time insights and alerts on data quality issues, teams can make quick adjustments and optimize processes on the fly. This agility and responsiveness are essential in today's fast-paced business environment, where adaptability and accuracy are key to staying ahead of the competition.
Introduction to Monte Carlo and Acceldata
Now that we have established the significance of data observability, let's delve deeper into the world of data management with two leading players in this space: Monte Carlo and Acceldata. Understanding the tools and technologies that drive data observability is crucial for organizations looking to maintain data integrity and optimize their data pipelines.
Overview of Monte Carlo
Monte Carlo stands out as a robust data observability tool that goes beyond traditional monitoring approaches. It excels in the proactive detection and prevention of data issues that could potentially disrupt critical workflows. Leveraging a combination of statistical techniques and cutting-edge machine learning algorithms, Monte Carlo is able to pinpoint data anomalies with precision, whether they are missing values, inconsistencies, or unexpected schema changes. This level of automated anomaly detection equips organizations with the ability to uphold a superior standard of data quality and confidently monitor their data pipelines in real-time.
Moreover, Monte Carlo's intuitive interface and customizable alerting mechanisms make it a preferred choice for data teams seeking a streamlined approach to data observability. By offering insights into data health and performance, Monte Carlo empowers organizations to proactively address potential issues and maintain a seamless data flow.
Overview of Acceldata
On the other end of the spectrum, Acceldata emerges as a comprehensive data observability platform designed to provide end-to-end visibility into data pipelines. With a focus on real-time data monitoring and advanced analytics, Acceldata equips organizations with the tools needed to detect anomalies, optimize data quality, and ensure adherence to data governance standards. Its robust integration capabilities enable seamless connectivity with a wide range of data sources, making it a versatile solution for businesses of all sizes.
Acceldata's emphasis on user experience is evident in its user-friendly interface, which simplifies the complexities of data observability for users across different levels of technical expertise. By offering a holistic approach to data observability, Acceldata empowers organizations to make informed decisions based on real-time insights and drive data-driven strategies with confidence.
Key Features of Monte Carlo and Acceldata
Now that we have a general understanding of Monte Carlo and Acceldata, let's dig deeper into their key features to ascertain which tool aligns better with your organization's needs.
When it comes to data management tools like Monte Carlo and Acceldata, understanding their unique features can significantly impact your organization's data quality and performance. Let's explore in more detail the standout features of these two platforms to help you make an informed decision.
Unique Features of Monte Carlo
Monte Carlo's standout feature is its ability to proactively detect data anomalies. By leveraging machine learning algorithms, it analyzes historical patterns and statistical models to identify outliers, schema changes, and other potential data issues. Additionally, Monte Carlo offers data profiling and data lineage tracking, allowing users to track the source and quality of their data at each stage of the data pipeline.
Moreover, Monte Carlo's automated data monitoring and alerting system provide organizations with real-time notifications about any deviations from expected data behavior. This proactive approach to data quality assurance can help organizations prevent costly errors and ensure the reliability of their data-driven decisions.
Unique Features of Acceldata
Acceldata boasts an impressive array of features that cover the entire data observability spectrum. Its real-time data monitoring capabilities provide organizations with immediate visibility into data quality and performance. Acceldata's advanced analytics enable users to gain deeper insights into their data, with features like anomaly detection, data profiling, and data cataloging. Furthermore, its seamless integration with popular data platforms, such as Apache Kafka and Amazon S3, makes it a versatile choice for organizations with complex data infrastructure.
Additionally, Acceldata's collaborative data governance features empower teams to work together efficiently on data-related tasks. With role-based access control and data lineage visualization, organizations can ensure data integrity and compliance with regulatory requirements. By centralizing data management processes, Acceldata streamlines workflows and enhances collaboration across different teams within an organization.
Performance Analysis: Monte Carlo vs. Acceldata
When it comes to data observability, performance is a critical aspect. Let's analyze how Monte Carlo and Acceldata stack up in terms of speed, efficiency, scalability, and flexibility.
Before diving into the comparison, it's essential to understand the unique approaches that Monte Carlo and Acceldata take towards data observability. Monte Carlo focuses on anomaly detection through statistical techniques, providing organizations with a proactive way to identify and address data issues swiftly. On the other hand, Acceldata emphasizes real-time data monitoring, ensuring that anomalies are captured and resolved in near real-time to maintain smooth data operations.
Speed and Efficiency
Monte Carlo prides itself on its lightning-fast anomaly detection capabilities. By leveraging advanced statistical techniques, it quickly identifies data issues, allowing organizations to take proactive measures promptly. Acceldata, on the other hand, offers real-time data monitoring, ensuring that any anomalies are captured and addressed in near real-time, minimizing potential downtime and ensuring smooth data flows.
When it comes to efficiency, Monte Carlo's focus on rapid anomaly detection can help organizations save valuable time in identifying and resolving data issues. Acceldata's real-time monitoring, on the other hand, provides continuous oversight of data operations, allowing for immediate action in response to anomalies, ultimately enhancing operational efficiency.
Scalability and Flexibility
Both Monte Carlo and Acceldata are designed to handle large-scale data environments. Monte Carlo's scalable architecture allows it to seamlessly integrate with various data sources, ensuring observability across the entire data infrastructure. Acceldata, with its flexible deployment options and support for cloud-native environments, provides organizations with the agility to scale their data observability efforts as their business grows.
Scalability is a crucial factor in data observability tools, especially as organizations deal with ever-increasing volumes of data. Monte Carlo's ability to integrate with diverse data sources and its focus on scalability make it a robust choice for organizations looking to maintain observability in dynamic data environments. Acceldata's support for cloud-native environments adds an extra layer of flexibility, allowing organizations to adapt their data observability strategies to changing business needs seamlessly.
Usability and User Experience
Lastly, let's evaluate the usability and user experience of these two tools, as this plays a significant role in the overall effectiveness of a data observability solution.
User Interface Comparison
Monte Carlo offers a clean and intuitive user interface, making it easy for users to navigate and access vital information quickly. Its focus on simplicity and ease of use ensures that users can quickly identify data anomalies without getting bogged down in complex settings or configurations. Acceldata, with its visually appealing and well-organized interface, provides a seamless user experience, allowing users to monitor data pipelines effortlessly and gain valuable insights into their data quality.
Ease of Integration
One of the key strengths of both Monte Carlo and Acceldata lies in their ease of integration with existing data infrastructure. Monte Carlo seamlessly integrates with popular platforms like Snowflake, BigQuery, and Redshift, ensuring a smooth onboarding process. Acceldata's compatibility with a wide range of data platforms, such as Apache Kafka, Apache Spark, and various cloud services, makes it a versatile choice for organizations with diverse data ecosystems.
Conclusion
Data observability is a critical aspect of any data-driven organization. After a detailed analysis of the features, performance, and usability of Monte Carlo and Acceldata, it is evident that both tools bring unique strengths to the table. Monte Carlo excels in proactive anomaly detection, leveraging statistical techniques and machine learning algorithms. Acceldata, on the other hand, offers comprehensive end-to-end visibility, real-time monitoring, and advanced analytics.
Choosing the right data observability tool ultimately depends on your organization's specific requirements and priorities. By carefully assessing these tools in light of your existing data infrastructure and future growth plans, you can make an informed decision and ensure a robust data observability strategy for your business.
While Monte Carlo and Acceldata offer compelling features for data observability, CastorDoc takes data management to the next level by integrating advanced governance, cataloging, and lineage capabilities with a user-friendly AI assistant. Whether you're part of a data team seeking complete control over your data governance lifecycle or a business user aiming to harness data for strategic decision-making, CastorDoc provides a comprehensive and intuitive solution. To explore how CastorDoc compares to other tools and how it can empower your organization to enable self-service analytics, check out more tools comparisons here.
You might also like
Get in Touch to Learn More
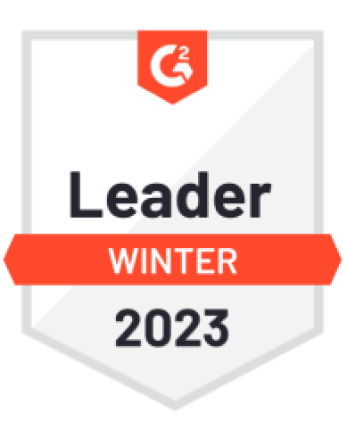
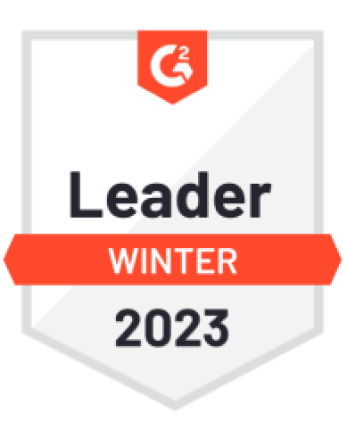

“[I like] The easy to use interface and the speed of finding the relevant assets that you're looking for in your database. I also really enjoy the score given to each table, [which] lets you prioritize the results of your queries by how often certain data is used.” - Michal P., Head of Data