Data Observability Tool Comparison: Monte Carlo vs. Anomalo
In the world of data management, ensuring the quality and reliability of your data is of utmost importance. As data grows more complex and voluminous, organizations rely on data observability tools to monitor, validate, and troubleshoot their data pipelines. Two popular options in this space are Monte Carlo and Anomalo. In this article, we will explore the features, functionality, and value for money offered by these two tools, providing you with the insight you need to make an informed decision for your organization.
Understanding Data Observability
Data observability refers to the ability to gain insights and maintain control over your data by measuring and monitoring its quality, accuracy, and consistency throughout its lifecycle. This practice enables organizations to proactively identify and resolve issues in their data pipelines, preventing downstream problems and ensuring data remains reliable and trustworthy.
The Importance of Data Observability
Data is the lifeblood of modern businesses, driving decision-making, informing strategies, and fueling innovations. However, data is only valuable if it can be trusted. Without proper observability, data quality issues can lead to wrong decisions, inaccurate predictions, and even compliance risks. Therefore, data observability is crucial for maintaining data integrity, delivering actionable insights, and building a foundation of trust in your data-driven initiatives.
Key Features of Data Observability Tools
Data observability tools offer a range of features to help organizations achieve comprehensive visibility into their data. These features typically include:
- Data Quality Monitoring: Tools that enable continuous monitoring of data quality metrics, such as completeness, accuracy, consistency, and timeliness.
- Data Lineage: The ability to track the origin and transformation of data, ensuring transparency and accountability throughout the data pipeline.
- Exception Detection: Tools that automatically identify and flag anomalies, outliers, and inconsistencies within the data, enabling timely corrective actions.
- Alerts and Notifications: Capabilities to send real-time alerts and notifications to stakeholders when data issues occur, minimizing the impact and enabling quick resolution.
- Collaboration: Features that facilitate collaboration and knowledge sharing among data teams, promoting a culture of data-driven decision-making.
Implementing data observability tools not only helps organizations ensure the quality and reliability of their data, but it also brings numerous other benefits. For example, by continuously monitoring data quality metrics, organizations can gain valuable insights into the performance of their data pipelines and identify areas for improvement. This allows them to optimize their data processes, streamline operations, and enhance overall efficiency.
In addition, data observability tools provide organizations with a comprehensive view of their data lineage, allowing them to trace the journey of data from its source to its destination. This visibility not only helps in ensuring data accuracy and consistency but also aids in compliance efforts by providing a clear audit trail.
Furthermore, the exception detection capabilities of data observability tools enable organizations to proactively identify and address data anomalies. By automatically flagging inconsistencies and outliers, these tools empower data teams to take immediate corrective actions, minimizing the impact on downstream processes and ensuring data reliability.
Lastly, the collaboration features offered by data observability tools foster a culture of data-driven decision-making within organizations. By facilitating knowledge sharing and collaboration among data teams, these tools enable cross-functional insights and encourage data-driven discussions. This collaborative approach leads to better decision-making, improved problem-solving, and increased innovation.
Introduction to Monte Carlo
Monte Carlo is a data observability tool designed to provide organizations with a comprehensive view of their data pipelines. By continuously monitoring data quality and detecting anomalies, Monte Carlo helps ensure the reliability and accuracy of data flowing through the system.
At its core, Monte Carlo is all about empowering organizations to have complete confidence in their data. With the increasing volume and complexity of data, it has become crucial for businesses to have a solution that can monitor, track, and detect issues in real-time. This is where Monte Carlo shines, offering a robust set of features that enable organizations to maintain data integrity and make informed decisions.
Overview of Monte Carlo's Functionality
Monte Carlo offers a range of features to support data observability, including:
- Data Monitoring: Monte Carlo continuously checks the quality and consistency of your data, alerting you to any issues or anomalies. It goes beyond simple data validation by monitoring data completeness, accuracy, and timeliness. This ensures that you have a complete picture of your data health at all times.
- Data Lineage Tracking: With Monte Carlo, you can easily trace the journey of your data, allowing you to identify bottlenecks, pinpoint errors, and ensure compliance. By visualizing the data lineage, you gain insights into how data flows through your pipelines, enabling you to optimize performance and troubleshoot issues effectively.
- Data Anomaly Detection: Utilizing advanced algorithms, Monte Carlo automatically detects and flags anomalies in your data, giving you the opportunity to investigate and resolve issues promptly. Whether it's missing values, unexpected spikes, or data inconsistencies, Monte Carlo empowers you to proactively address data quality issues before they impact your business.
- Real-time Alerts and Notifications: Monte Carlo sends real-time alerts and notifications to relevant stakeholders, ensuring that data issues are promptly addressed. By providing instant visibility into data anomalies, Monte Carlo enables teams to collaborate and take immediate action, minimizing the impact on downstream processes.
With these powerful features, Monte Carlo becomes an indispensable tool for organizations striving to maintain data integrity and make data-driven decisions with confidence.
Pros and Cons of Using Monte Carlo
Pros:
- Easy to Use: Monte Carlo offers a user-friendly interface and intuitive design, making it accessible to both technical and non-technical users. You don't need to be a data expert to leverage the power of Monte Carlo and ensure data quality.
- Comprehensive Data Monitoring: With Monte Carlo, you can monitor the quality of your data in real-time, enabling rapid identification and resolution of data issues. This proactive approach saves valuable time and resources, ensuring that your data is always reliable and trustworthy.
- Automatic Anomaly Detection: Monte Carlo's advanced algorithms automatically identify and flag data anomalies, reducing the time and effort required for manual inspection. This frees up your team to focus on higher-value tasks, such as data analysis and decision-making.
Cons:
- Limited Data Processing Capabilities: While Monte Carlo excels in data monitoring and anomaly detection, it may not offer the same level of data processing capabilities as other tools. If your organization requires extensive data transformation or complex data manipulation, you may need to supplement Monte Carlo with additional tools.
- Cost: Monte Carlo's pricing structure may not be suitable for organizations with budget constraints, especially considering the additional costs associated with data storage and processing. It's important to carefully evaluate your organization's needs and budget before committing to Monte Carlo.
Despite these considerations, Monte Carlo remains a powerful solution for organizations seeking to ensure the reliability and accuracy of their data. Its comprehensive data observability features, combined with its user-friendly interface, make it a valuable asset in the modern data landscape.
Introduction to Anomalo
Anomalo is another player in the data observability space, providing organizations with the tools they need to ensure data quality and reliability.
Overview of Anomalo's Functionality
Anomalo offers a range of features to support data observability, including:
- Data Quality Monitoring: Anomalo enables organizations to continuously monitor the quality of their data, ensuring consistency, accuracy, and completeness.
- Data Lineage Tracking: With Anomalo, you can easily trace the origin and transformation of your data, allowing for better error tracking and root cause analysis.
- Anomaly Detection: Anomalo's anomaly detection algorithms automatically identify and flag outliers in your data, providing the opportunity for timely investigation and resolution.
- Alerts and Notifications: Anomalo sends real-time alerts and notifications to stakeholders when data anomalies or issues are detected, allowing for immediate action.
Pros and Cons of Using Anomalo
Pros:
- Robust Data Monitoring: Anomalo provides real-time monitoring of data quality, helping organizations maintain high standards of data integrity.
- User-Friendly Interface: Anomalo offers a simple and intuitive interface, making it easy for users to navigate and leverage the tool's capabilities.
- Anomaly Detection: Anomalo's sophisticated algorithms excel at identifying data anomalies, allowing organizations to swiftly address potential issues.
Cons:
- Limited Data Processing Capabilities: Anomalo's primary focus is on data monitoring and anomaly detection, making it less suitable for complex data processing tasks.
- Pricing Complexity: Anomalo's pricing structure may be complex, and additional costs for storage and processing can make it less cost-effective for some organizations.
Detailed Comparison Between Monte Carlo and Anomalo
Comparing User Interface and Ease of Use
Both Monte Carlo and Anomalo offer user-friendly interfaces, making it easy for users to navigate and leverage the tools' capabilities. However, the specific layout and design may vary, and user preferences may influence which tool resonates best with your organization's needs.
Comparing Data Processing Capabilities
While both Monte Carlo and Anomalo excel at data monitoring and anomaly detection, their data processing capabilities differ. Monte Carlo may be more suitable for organizations that prioritize real-time data quality monitoring, while Anomalo may be a better fit for organizations requiring robust data lineage tracking and root cause analysis.
Comparing Alert and Notification Systems
Both tools offer alert and notification systems to inform stakeholders of data issues or anomalies promptly. The specifics of these systems, such as the level of customization and integrations available, may differ, and organizations should consider their unique requirements when making a decision.
Pricing and Value for Money
Monte Carlo Pricing Structure
Monte Carlo employs a subscription-based pricing model, with costs varying based on factors such as data volume, number of users, and additional features required. Organizations should carefully evaluate their budget and data observability needs to determine if Monte Carlo's pricing aligns with their financial goals.
Anomalo Pricing Structure
Anomalo adopts a similar subscription-based pricing model, with costs dependent on factors such as data volume, number of users, and specific features required. Organizations should consider their data observability requirements and budget constraints when assessing the value for money offered by Anomalo.
In conclusion, both Monte Carlo and Anomalo offer powerful data observability tools that can help organizations maintain the quality and reliability of their data pipelines. Your choice between these tools should be guided by your unique needs, considering factors such as data monitoring capabilities, data processing requirements, user interface preferences, and budget constraints. By understanding the features, functionalities, and pricing structures of these tools, you can make an informed decision that ensures your organization experiences the full value of data observability.While Monte Carlo and Anomalo provide valuable insights into data observability, CastorDoc takes it a step further by integrating advanced governance, cataloging, and lineage capabilities with a user-friendly AI assistant. CastorDoc's powerful platform is designed to enable self-service analytics, making it an ideal choice for businesses looking to enhance their data governance and utilization. Whether you're a data professional seeking comprehensive control or a business user desiring accessible and understandable data, CastorDoc offers the tailored support you need. To explore how CastorDoc compares to other tools and to discover the perfect fit for your organization's data needs, check out more tools comparisons here.
You might also like
Get in Touch to Learn More
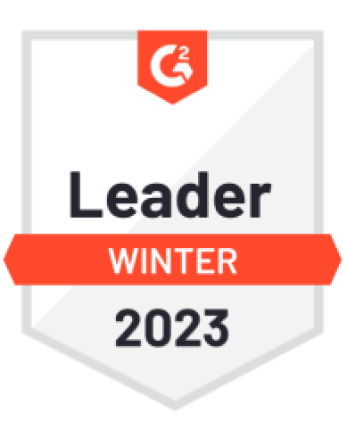
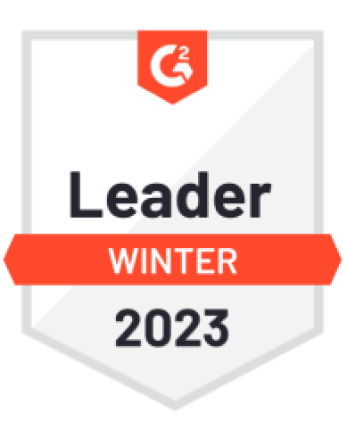

“[I like] The easy to use interface and the speed of finding the relevant assets that you're looking for in your database. I also really enjoy the score given to each table, [which] lets you prioritize the results of your queries by how often certain data is used.” - Michal P., Head of Data