Data Observability Tool Comparison: Monte Carlo vs. Lantern
Data observability is a critical aspect of any data-driven organization. It refers to the ability to understand, monitor, and control the quality and reliability of data, ensuring its accuracy and consistency throughout the data pipeline. In this comparison article, we will explore two prominent data observability tools: Monte Carlo and Lantern. We will delve into their features, capabilities, and pricing structures to help you make an informed decision for your organization's data needs.
Understanding Data Observability
Data observability is an essential practice in modern data infrastructure. As the volume and complexity of data continue to grow, organizations need to ensure that their data is trustworthy, reliable, and compliant. Data observability tools enable businesses to gain valuable insights into the health and quality of their data, making it easier to identify and resolve issues such as data downtime, data drift, and data quality gaps.
The Importance of Data Observability
Data drives decision-making in today's business landscape. However, relying on inaccurate or unreliable data can have severe consequences. Without proper data observability, organizations risk making flawed decisions, compromising customer trust, and facing legal and regulatory issues.
For example, imagine a retail company that relies on data to forecast customer demand. If their data observability is lacking, they may end up overstocking certain products, leading to unnecessary costs and wasted resources. On the other hand, if they underestimate demand due to poor data quality, they may face stockouts and lose potential sales, damaging their reputation and customer satisfaction.
By implementing a robust data observability strategy and leveraging tools such as Monte Carlo and Lantern, organizations can gain confidence in their data and minimize the risks associated with poor data quality.
Key Features of Data Observability Tools
Data observability tools provide a range of essential features to ensure data reliability and quality. Some key features to consider when evaluating data observability tools include:
- Data Monitoring: Real-time monitoring of data pipelines to detect anomalies, inconsistencies, and data downtime.
- Data Validation: Automated validation of data quality, including schema validation, data type validation, and business rule validation.
- Data Lineage: Tracking and documentation of data lineage to understand the origin, transformation, and movement of data within an organization.
- Alerting and Notifications: Proactive alerts and notifications to inform data users and stakeholders about any data issues or anomalies.
- Data Profiling: Analyzing the content and structure of data to identify patterns, anomalies, and potential issues.
These features work together to provide organizations with a comprehensive view of their data, ensuring its accuracy, completeness, and timeliness. Data monitoring allows businesses to detect any abnormalities in their data pipelines, enabling them to take immediate action to resolve issues and minimize data downtime.
Data validation plays a crucial role in ensuring the quality of data. By automating the validation process, organizations can quickly identify and rectify any data inconsistencies or errors, preventing them from propagating throughout the system.
Data lineage provides transparency into the journey of data, allowing organizations to understand how data is transformed and where it comes from. This knowledge is vital for compliance purposes and for troubleshooting any data-related issues that may arise.
Alerting and notifications keep data users and stakeholders informed about any data issues or anomalies in real-time. This proactive approach enables organizations to address problems promptly, minimizing the impact on decision-making processes.
Data profiling, on the other hand, helps organizations gain a deeper understanding of their data. By analyzing the content and structure of data, businesses can identify patterns, anomalies, and potential issues that may affect data quality and reliability.
In conclusion, data observability is a critical practice for organizations seeking to ensure the trustworthiness and reliability of their data. By implementing robust data observability strategies and leveraging the features offered by data observability tools, businesses can make informed decisions, mitigate risks, and drive success in today's data-driven world.
Introduction to Monte Carlo
Monte Carlo is a powerful data observability tool that helps organizations ensure the accuracy, quality, and trustworthiness of their data. With its comprehensive suite of features, Monte Carlo enables data teams to gain visibility into data issues, prevent data incidents, and streamline their data operations.
Organizations across various industries rely on Monte Carlo to maintain data integrity and drive informed decision-making processes. By leveraging cutting-edge technology and advanced algorithms, Monte Carlo empowers data professionals to proactively manage data quality and consistency, ultimately enhancing the reliability of their data-driven initiatives.
Overview of Monte Carlo
Monte Carlo uses advanced statistical methods to detect data anomalies, identify data discrepancies, and predict potential data incidents. By continuously monitoring data sources and pipelines, Monte Carlo provides data teams with real-time insights into the health and quality of their data, helping them take proactive measures to prevent data issues.
Furthermore, Monte Carlo's intuitive dashboard and customizable alerts enable data teams to prioritize their efforts and focus on resolving critical data issues promptly. This proactive approach not only minimizes the risk of data errors but also optimizes data workflows, ensuring seamless data delivery and analysis.
Unique Features of Monte Carlo
Monte Carlo offers several unique features that set it apart as a leading data observability tool:
- Data Monitoring at Scale: Monte Carlo can handle large-scale data operations, making it suitable for organizations dealing with vast amounts of data.
- Data Quality Profiles: Monte Carlo automatically creates data quality profiles, allowing data teams to understand the overall quality of their data at a glance.
- Collaborative Workflow: Monte Carlo provides a collaborative environment for data teams, enabling them to collaborate, share insights, and resolve data issues efficiently.
Moreover, Monte Carlo's machine learning capabilities continuously adapt to evolving data landscapes, ensuring that data teams stay ahead of potential challenges and maintain data accuracy over time. By harnessing the power of automation and predictive analytics, Monte Carlo empowers organizations to proactively address data quality issues and drive data-driven innovation with confidence.
Introduction to Lantern
Lantern is another robust data observability tool that focuses on ensuring reliable and high-quality data. With its intuitive interface and powerful capabilities, Lantern empowers data teams to gain complete visibility into their data health, resolve data issues faster, and optimize their data operations effectively.
Overview of Lantern
Lantern excels at providing real-time insights into data quality and data anomalies. By integrating with existing data infrastructure, Lantern helps data teams validate data quality, visualize data pipelines, and streamline their data operations.
Unique Features of Lantern
Lantern stands out from other data observability tools due to its unique features:
- Data Pipeline Visualization: Lantern visually represents data pipelines, making it easier for data teams to understand the flow of data and identify potential bottlenecks or issues.
- Data Profiling and Alerting: Lantern offers extensive data profiling capabilities, allowing data teams to identify data anomalies and receive real-time alerts for any critical data issues.
- Intuitive User Interface: Lantern's user interface is user-friendly and intuitive, making it accessible for both technical and non-technical users.
Comparing Monte Carlo and Lantern
Ease of Use: Monte Carlo vs. Lantern
Both Monte Carlo and Lantern provide user-friendly interfaces that make it easy for data teams to navigate and use their respective tools. However, Monte Carlo's collaborative workflow and intuitive design give it an edge in terms of ease of use, enabling efficient collaboration among data team members.
Lantern, on the other hand, focuses on simplicity and accessibility, catering to both technical and non-technical users, making it an excellent choice for organizations with diverse skill sets.
Data Accuracy: Monte Carlo vs. Lantern
Ensuring data accuracy is paramount for any data observability tool. Both Monte Carlo and Lantern excel in detecting data inaccuracies and anomalies.
Monte Carlo's statistical modeling capabilities and data quality profiles provide a comprehensive understanding of data accuracy. Lantern's data profiling and alerting features also play a crucial role in identifying and resolving data accuracy issues.
Ultimately, the choice between Monte Carlo and Lantern depends on the specific data accuracy requirements and the complexity of your data operations.
Scalability: Monte Carlo vs. Lantern
When it comes to scalability, Monte Carlo has a clear advantage. Its ability to handle large-scale data operations makes it ideal for organizations dealing with massive data volumes.
While Lantern is scalable and can cater to the needs of most organizations, it may not be as suitable for highly complex data environments.
Consider your organization's data volume and complexity to determine the scalability requirements that align with your business needs.
Pricing Comparison
Monte Carlo Pricing Structure
Monte Carlo offers various pricing plans tailored to the specific needs of organizations. Its pricing structure typically includes a monthly or annual subscription based on factors such as data volume, number of users, and additional features required.
For more detailed information on pricing, we recommend reaching out to Monte Carlo's sales team to discuss your specific requirements.
Lantern Pricing Structure
Lantern follows a similar pricing model to Monte Carlo, with cost depending on factors such as data volume, number of users, and additional features.
For accurate and up-to-date pricing information, we recommend contacting Lantern's sales team to discuss your organization's needs and obtain a customized pricing quote.
In conclusion, both Monte Carlo and Lantern offer powerful data observability tools with unique features and capabilities. The choice between them depends on your organization's specific requirements in terms of ease of use, data accuracy, scalability, and pricing. Evaluating these factors and conducting thorough research will help you make an informed decision that aligns with your data observability goals.
Remember, investing in a reliable data observability tool is crucial for maintaining the integrity and trustworthiness of your data, ultimately enabling you to make data-driven decisions with confidence.
As you consider the right data observability tool for your organization, remember that the journey towards robust data governance doesn't end here. CastorDoc offers a unique blend of advanced governance, cataloging, and lineage capabilities, complemented by a user-friendly AI assistant to enable self-service analytics. With CastorDoc, you can take control of your data governance lifecycle, ensure compliance, and empower your business users to leverage data with confidence. To explore how CastorDoc compares to other tools in the modern data stack and to find the perfect fit for your data needs, check out more tools comparisons here.
You might also like
Get in Touch to Learn More
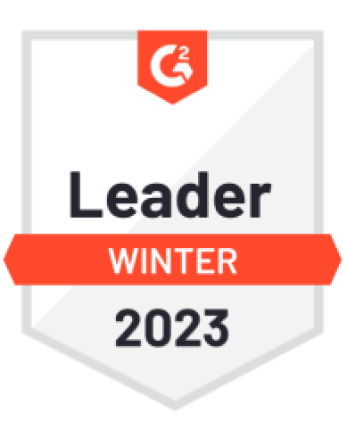
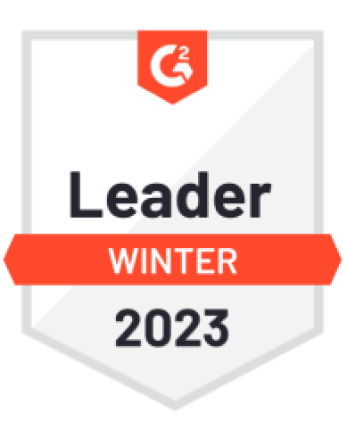

“[I like] The easy to use interface and the speed of finding the relevant assets that you're looking for in your database. I also really enjoy the score given to each table, [which] lets you prioritize the results of your queries by how often certain data is used.” - Michal P., Head of Data