Data Observability Tool Comparison: Monte Carlo vs. Lightup
Data observability tools are crucial for ensuring the integrity and reliability of data in today's data-driven world. In this article, we will compare two prominent data observability tools: Monte Carlo and Lightup. By understanding the importance of data observability and exploring the key features of these tools, we can make an informed decision about which one suits our specific needs.
Understanding Data Observability
Data observability refers to the ability to gain insight into the health, quality, and accuracy of data across an organization's systems and processes. It allows businesses to detect and resolve data issues promptly, ensuring data reliability and generating trustworthy insights.
Imagine a scenario where a company is making critical decisions based on inaccurate or incomplete data. The consequences could be disastrous. Data observability acts as a safeguard, providing organizations with the necessary tools to ensure the integrity of their data. By monitoring data pipelines in real-time, businesses can proactively identify and address any issues that may arise, preventing potential pitfalls and enabling data-driven decision-making.
The Importance of Data Observability
Data lies at the heart of decision-making for organizations in various industries. Without reliable and accurate data, businesses risk making flawed decisions that can have severe consequences. Data observability allows organizations to mitigate these risks by providing visibility into the quality of data, preventing potential pitfalls and enabling data-driven decision-making.
Moreover, data observability plays a vital role in ensuring regulatory compliance, especially in industries that deal with sensitive customer data, such as healthcare and finance. By monitoring data pipelines, organizations can identify any anomalies or discrepancies, ensuring compliance with data protection laws and regulations.
Consider a healthcare organization that handles patient records. Data observability becomes crucial in this context, as any inaccuracies or breaches in data security could compromise patient privacy and violate regulatory requirements. By implementing data observability tools, healthcare organizations can proactively monitor their data pipelines, detect any potential issues, and take immediate action to rectify them, ensuring compliance and maintaining the trust of their patients.
Key Features of Data Observability Tools
Data observability tools offer a range of features aimed at achieving comprehensive visibility into data pipelines. These features typically include:
- Real-time data monitoring: Tools provide real-time monitoring capabilities, allowing organizations to detect and address issues as they arise.
- Data anomaly detection: Software uses machine learning algorithms to identify abnormal patterns and outliers, enabling the early detection of data issues.
- Alerting and notification: Tools notify users through alerts and notifications when data anomalies or issues are detected.
- Data lineage and impact analysis: These features allow users to trace data from its source, track changes, and understand the impact of any modifications made throughout the data pipeline.
Real-time data monitoring is particularly valuable in fast-paced industries where decisions need to be made quickly. By having instant visibility into the health of their data, organizations can proactively address any issues, ensuring that accurate and reliable information is available when needed.
Data anomaly detection goes beyond traditional error-checking methods by leveraging machine learning algorithms. These algorithms can identify patterns and outliers that may indicate data issues, even in complex and large datasets. By detecting anomalies early on, organizations can prevent the propagation of inaccurate or faulty data throughout their systems.
Alerting and notification features provide organizations with timely information about data anomalies or issues. This allows teams to respond promptly, minimizing the potential impact on operations and decision-making. By receiving alerts, stakeholders can take immediate action, ensuring that data quality is maintained and potential risks are mitigated.
Data lineage and impact analysis features enable organizations to trace the journey of data from its source to its destination. This capability is particularly valuable when investigating data issues or understanding the impact of changes made to the data pipeline. By having a clear understanding of the data's lineage and the modifications it undergoes, organizations can identify potential bottlenecks or areas for improvement, enhancing the overall data quality and reliability.
An Introduction to Monte Carlo
Monte Carlo is a leading data observability tool that helps organizations gain deep visibility into their data pipelines. It focuses on ensuring data reliability and driving data-informed decision-making.
With the exponential growth of data in today's digital landscape, the need for robust data observability tools like Monte Carlo has become increasingly crucial. By providing organizations with a comprehensive view of their data pipelines, Monte Carlo empowers data teams to proactively identify and address issues, ultimately enhancing the overall data quality and integrity.
Overview of Monte Carlo's Functionality
Monte Carlo offers a range of functionality to address various aspects of data observability:
- Data monitoring: Monte Carlo monitors data quality metrics in real-time, providing visibility into the health of data pipelines. It provides pre-built detectors for common data issues such as NULL values, schema changes, and data drift.
- Anomaly detection: Leveraging advanced statistical models, Monte Carlo detects anomalies in data patterns, enabling early detection of data issues and preventing downstream impacts.
- Data profiling: Monte Carlo allows users to perform comprehensive data profiling, enabling a deep understanding of data quality, distribution, and patterns.
Furthermore, Monte Carlo's anomaly detection capabilities go beyond traditional rule-based systems, utilizing machine learning algorithms to adapt to evolving data patterns and proactively identify abnormalities that may go unnoticed by manual monitoring processes. This proactive approach not only saves time but also minimizes the risk of data-related incidents that could impact critical business operations.
Pros and Cons of Monte Carlo
Pros:
- Easy to use interface with intuitive dashboards.
- Comprehensive monitoring and anomaly detection capabilities.
- Robust data profiling and exploration features.
Cons:
- Limited integrations with certain data sources and platforms.
- Advanced features may require technical expertise to leverage fully.
An Introduction to Lightup
Lightup is another notable data observability tool that focuses on ensuring data reliability and visibility. With its advanced features and user-friendly interface, Lightup is designed to provide organizations with the tools they need to monitor and maintain high-quality data across their pipelines.
By leveraging Lightup, data teams can gain valuable insights into the health of their data, enabling them to make informed decisions and take proactive measures to address any issues that may arise.
Overview of Lightup's Functionality
Lightup provides a range of features to enhance data observability:
- Real-time data monitoring: Lightup offers real-time monitoring of data quality across an organization's data pipelines, ensuring prompt detection and resolution of data issues.
- Customizable data checks: Users can define their custom data checks using SQL queries or Python scripts, giving them the flexibility to monitor specific data quality aspects.
- Collaborative data issue resolution: Lightup facilitates collaboration among teams to resolve data issues efficiently, ensuring data is reliable and accurate.
With its real-time monitoring capabilities, Lightup empowers organizations to proactively identify and address data quality issues before they impact business operations. The customizable data checks feature allows users to tailor their monitoring efforts to focus on the most critical aspects of their data, ensuring that they can maintain high standards of data quality.
Pros and Cons of Lightup
Pros:
- User-friendly interface with easy-to-navigate dashboards.
- Customizable data checks offer flexibility in monitoring specific data quality aspects.
- Efficient collaboration features for issue resolution.
Cons:
- Limited advanced anomaly detection capabilities compared to other tools.
- Integration with certain data sources may require additional configuration.
Despite its limitations in advanced anomaly detection, Lightup's focus on real-time monitoring and customizable data checks makes it a valuable tool for organizations looking to maintain data reliability and visibility.
Detailed Comparison Between Monte Carlo and Lightup
Comparing User Interface and Ease of Use
Both Monte Carlo and Lightup offer user-friendly interfaces with intuitive dashboards. However, Monte Carlo's interface provides more comprehensive visualizations, enabling users to quickly gain insights into data issues. Lightup's interface is simpler and easier to navigate, making it suitable for users who prefer a more streamlined experience.
Comparing Data Monitoring Capabilities
Monte Carlo and Lightup both offer real-time data monitoring capabilities. Monte Carlo, however, provides a broader range of pre-built detectors for common data issues, offering more comprehensive visibility into data pipelines. Lightup, on the other hand, allows users to define custom data checks, providing flexibility in monitoring specific aspects of data quality.
Comparing Alerting and Reporting Features
Both tools offer alerting and notification features to inform users of data issues. Monte Carlo provides more advanced alerting capabilities, allowing users to set thresholds and specify notification channels. Lightup's alerting features are more basic in comparison. When it comes to reporting, both tools offer comprehensive reporting capabilities, enabling users to generate custom reports based on specific data quality metrics.
Pricing Structure: Monte Carlo vs. Lightup
Understanding Monte Carlo's Pricing
Monte Carlo's pricing is based on the volume of data processed and the number of data sources connected. Pricing tiers are available to accommodate organizations of different sizes, with custom enterprise plans available for larger enterprises.
Understanding Lightup's Pricing
Lightup's pricing structure is also based on the volume of data processed and the number of data sources connected. Similar to Monte Carlo, Lightup offers pricing tiers tailored to organizations of different sizes.
In conclusion, choosing the right data observability tool is essential for maintaining data integrity and reliability. Both Monte Carlo and Lightup offer powerful features to enhance data observability, but their strengths lie in different areas. While Monte Carlo provides a comprehensive solution with advanced anomaly detection and monitoring capabilities, Lightup offers a user-friendly interface with customizable data checks and efficient collaboration features. Assessing your organization's specific needs and comparing these tools' functionality and pricing structure will help you make an informed decision and take your data observability to the next level.While Monte Carlo and Lightup offer valuable insights into data observability, CastorDoc takes data management to the next level by integrating advanced governance, cataloging, and lineage capabilities with a user-friendly AI assistant. CastorDoc's powerful platform enables self-service analytics, allowing both data teams and business users to navigate the complexities of data governance with ease. Whether you're looking to manage data catalogs, ensure compliance, maintain data quality, or empower business users to find and utilize data effectively, CastorDoc is your go-to solution. To explore how CastorDoc compares to other tools and to discover how it can revolutionize your data governance and analytics, check out more tools comparisons here.
You might also like
Get in Touch to Learn More
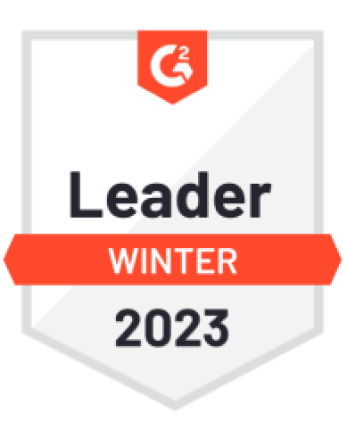
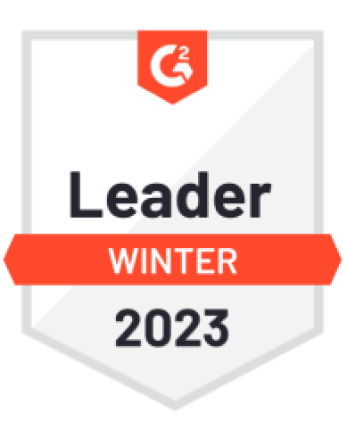

“[I like] The easy to use interface and the speed of finding the relevant assets that you're looking for in your database. I also really enjoy the score given to each table, [which] lets you prioritize the results of your queries by how often certain data is used.” - Michal P., Head of Data