Data Observability Tool Comparison: Monte Carlo vs. Sifflet
Data observability has become increasingly critical in today's data-driven world. As organizations rely more and more on data analytics to drive their decision-making processes, it has become essential to ensure the quality, reliability, and accuracy of the data being used. In this article, we will compare two popular data observability tools: Monte Carlo and Sifflet. By understanding their key features, pros and cons, as well as conducting a detailed comparison between the two, we aim to help you make an informed decision on which tool is best suited for your organization's needs.
Understanding Data Observability
Data observability is the ability to measure and monitor the quality, consistency, and availability of data. It involves identifying and resolving any issues or anomalies that may impact the reliability and trustworthiness of data. By ensuring data observability, organizations can have confidence in the accuracy and validity of their data, leading to more reliable insights and informed decision-making.
The Importance of Data Observability
Data observability plays a crucial role in maintaining data integrity and ensuring the success of data-driven initiatives. When inconsistencies or inaccuracies go undetected in the data, organizations risk making incorrect conclusions and poor decisions. This can have severe consequences, such as financial losses, reputational damage, and compliance violations. By implementing robust data observability practices and utilizing appropriate tools, organizations can prevent data-related issues before they lead to costly mistakes.
Key Features of Data Observability Tools
Effective data observability tools offer a range of features designed to enable organizations to monitor and manage their data efficiently. These features may include:
- Data Quality Monitoring: Tools that provide insights into the quality and accuracy of data, highlighting any anomalies or inconsistencies.
- Data Lineage Tracking: The ability to trace the origins and transformations of data, allowing organizations to understand how data flows and changes over time.
- Data Anomaly Detection: Algorithms that identify unusual patterns or outliers in data, flagging potential issues that may require investigation.
- Data Profiling: Tools that analyze the structure, content, and relationships within datasets for a comprehensive understanding of data characteristics.
Additionally, data observability tools often offer advanced visualization capabilities, allowing users to gain a deeper understanding of their data through interactive charts, graphs, and dashboards. These visualizations can help identify trends, patterns, and correlations that may not be immediately apparent in raw data. By presenting data in a visually engaging manner, organizations can enhance their data exploration and analysis processes, leading to more meaningful insights.
Furthermore, data observability tools may provide automated alerting and notification systems. These systems can be configured to send alerts to designated individuals or teams when specific data anomalies or issues are detected. This proactive approach to monitoring allows organizations to address potential problems swiftly, minimizing the impact on data integrity and decision-making processes.
Another important aspect of data observability tools is their ability to integrate with various data sources and platforms. Whether an organization's data resides in on-premises databases, cloud-based storage, or a combination of both, these tools can connect to and gather data from multiple sources. This flexibility enables organizations to achieve a holistic view of their data ecosystem, ensuring comprehensive observability across all data assets.
Introduction to Monte Carlo
Monte Carlo is a leading data observability tool that helps organizations monitor the quality and reliability of their data pipelines. With its intuitive interface and powerful features, Monte Carlo has gained popularity among data professionals seeking to ensure data accuracy and trust.
But what exactly is data observability, and why is it crucial for organizations? Data observability refers to the ability to monitor, understand, and act upon the health and reliability of data in real-time. In today's data-driven world, where decisions are increasingly based on data insights, organizations cannot afford to overlook the importance of data quality. That's where Monte Carlo comes in.
Overview of Monte Carlo
Monte Carlo allows organizations to track data quality in real-time and receive alerts for potential issues. By monitoring data health metrics, such as freshness, volume, and schema changes, Monte Carlo provides valuable insights into the overall reliability of data. With its automated monitoring capabilities, organizations can catch data issues early and take proactive steps to rectify them.
Imagine a scenario where a critical business decision is about to be made based on data insights. Without data observability, there is a risk of relying on inaccurate or outdated data, leading to flawed decision-making. Monte Carlo helps mitigate this risk by providing continuous monitoring and alerting, ensuring that organizations have a clear understanding of the quality and reliability of their data.
Key Features of Monte Carlo
Monte Carlo offers several key features that make it a powerful data observability tool:
- Data Monitoring: Monte Carlo continuously monitors critical data health metrics, ensuring that any changes or anomalies are promptly identified.
- Data Quality Alerts: Organizations can set up customized alerts based on predetermined thresholds, enabling them to take immediate action when data quality drops below acceptable levels.
- Data Lineage Tracking: Monte Carlo provides insights into the lineage of data, giving organizations full visibility into where data originates and how it is transformed throughout the pipeline.
- Collaboration and Reporting: Monte Carlo facilitates collaboration among data teams and stakeholders by providing real-time dashboards, reports, and visualizations.
Let's dive deeper into the data lineage tracking feature. Understanding the lineage of data is crucial for ensuring data accuracy and trust. With Monte Carlo, organizations can trace the journey of data from its source to its destination, identifying any transformations or modifications along the way. This visibility not only helps in troubleshooting data issues but also enables organizations to maintain compliance with data governance regulations.
Furthermore, the collaboration and reporting capabilities of Monte Carlo foster a culture of data-driven decision-making. Data teams can easily share insights, collaborate on resolving issues, and provide stakeholders with real-time access to data health metrics. This transparency and accessibility empower organizations to make informed decisions based on reliable data.
Pros and Cons of Monte Carlo
Monte Carlo offers several advantages, such as comprehensive data monitoring and alerting capabilities, real-time data lineage tracking, and user-friendly interfaces. However, it is essential to consider potential limitations, such as the need for proper integration with existing data infrastructure and the associated costs of implementation and maintenance.
Despite these considerations, the benefits of Monte Carlo outweigh the challenges for organizations that prioritize data quality and reliability. By leveraging the power of Monte Carlo, organizations can gain a competitive edge by making data-driven decisions with confidence.
Introduction to Sifflet
Sifflet is another notable player in the data observability space, offering organizations a range of features to monitor and ensure data quality throughout their pipelines.
Overview of Sifflet
Sifflet enables organizations to detect and resolve data issues early on, saving time and resources that would otherwise be spent on manual troubleshooting. By continuously monitoring data health metrics and providing actionable insights, Sifflet empowers data teams to maintain high-quality data and drive reliable analytics.
Key Features of Sifflet
Sifflet offers the following key features to enhance data observability:
- Data Monitoring and Alerts: Sifflet tracks data quality metrics and sends notifications when predefined thresholds are exceeded, ensuring timely resolution of potential issues.
- Data Validation: Sifflet validates data integrity and accuracy by performing checks against predefined rules and constraints.
- Data Profiling: With its data profiling capabilities, Sifflet provides a comprehensive understanding of data characteristics, helping organizations identify and address any data-related issues.
- Integration Flexibility: Sifflet seamlessly integrates with various data platforms, making it a flexible choice for organizations with diverse data ecosystems.
Pros and Cons of Sifflet
Sifflet offers organizations numerous benefits, including comprehensive data monitoring and validation capabilities, flexible integration options, and robust data profiling features. However, organizations should consider factors such as the learning curve associated with implementation and customization requirements to ensure a smooth adoption process.
Detailed Comparison Between Monte Carlo and Sifflet
Now that we have explored the key features and capabilities of both Monte Carlo and Sifflet, let us conduct a detailed comparison to help you make an informed decision:
User Interface Comparison
Both Monte Carlo and Sifflet offer user-friendly interfaces that enable users to monitor data health metrics, set up alerts, and gain insights with ease. However, Monte Carlo's intuitive dashboard design and visualization capabilities provide a slight edge in terms of overall user experience.
Performance and Speed Comparison
Monte Carlo and Sifflet are both designed to deliver real-time insights and alerts, ensuring timely resolution of data issues. While both tools offer impressive performance, Monte Carlo's optimized algorithms and streamlined processes provide quicker and more efficient data observability, making it a preferred choice for organizations with large datasets and strict performance requirements.
Scalability Comparison
When it comes to scalability, Monte Carlo's cloud-native architecture offers seamless scalability and flexibility to accommodate growing data volumes and complex data pipelines. Sifflet, while scalable as well, may require additional configuration and resources to handle large-scale data environments.
Pricing Comparison
Monte Carlo and Sifflet offer flexible pricing models tailored to meet the needs of different organizations. Monte Carlo's pricing is primarily based on the volume of data being monitored, while Sifflet's pricing structure is influenced by factors such as the number of data connectors and customizations required. It is important to evaluate and compare pricing plans based on your organization's specific requirements and budget.
In conclusion, both Monte Carlo and Sifflet are robust data observability tools that can help organizations ensure the quality and reliability of their data pipelines. Each tool offers unique features and advantages, and the choice between the two ultimately depends on your organization's specific needs, budget, and preferences. By carefully considering the key features, pros and cons, and detailed comparison provided in this article, you can make an informed decision that aligns with your data observability goals and objectives.
While Monte Carlo and Sifflet offer compelling solutions for data observability, CastorDoc takes a holistic approach to data management, combining advanced governance, cataloging, and lineage capabilities with an AI-driven user experience. Whether you're a data professional seeking comprehensive control over your data governance lifecycle or a business user in need of intuitive data accessibility, CastorDoc is designed to meet your needs. To explore how CastorDoc stands out in the landscape of data tools and to find more insightful comparisons like this one, check out more tools comparisons here.
You might also like
Get in Touch to Learn More
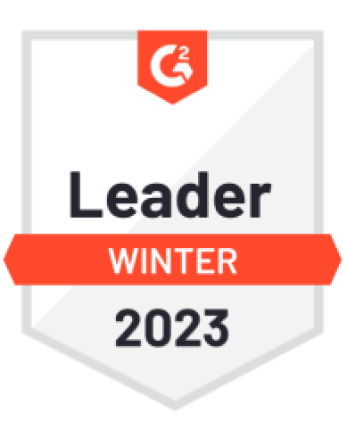
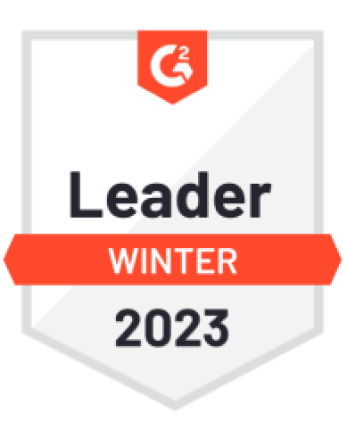

“[I like] The easy to use interface and the speed of finding the relevant assets that you're looking for in your database. I also really enjoy the score given to each table, [which] lets you prioritize the results of your queries by how often certain data is used.” - Michal P., Head of Data