Data Observability Tool Comparison: Soda vs. Monte Carlo
In this article, we will dive into a detailed comparison between two popular data observability tools: Soda and Monte Carlo. Data observability is a critical aspect of any data-driven organization, ensuring that data is accurate, reliable, and meets the intended quality standards. With the increasing volume and complexity of data, it is crucial to have effective tools that can monitor, validate, and alert on data issues. Soda and Monte Carlo are both powerful solutions in this space, each with its own unique set of features and capabilities. Let's explore them further.
Understanding Data Observability
Data observability refers to the ability to gain insights into the quality and health of your data. It involves continuously monitoring data pipelines, validating data for accuracy, and proactively identifying and resolving any issues that may arise. By ensuring data observability, organizations can make informed decisions based on reliable and trustworthy data, minimizing the risk of making faulty assumptions or incorrect conclusions.
The Importance of Data Observability
Data observability is crucial for maintaining data integrity and ensuring the overall health of your data infrastructure. Without proper observability, organizations may encounter data quality issues, such as missing or inaccurate data, which can lead to flawed analyses and incorrect business decisions. By implementing a robust data observability strategy, organizations can save significant time and resources that would otherwise be wasted on detecting and remedying data issues manually.
Key Features of Data Observability Tools
Effective data observability tools offer a wide range of features and functionalities to address the specific needs of data-driven organizations. These features typically include:
- Data Monitoring: Real-time monitoring of data pipelines to detect anomalies or issues.
- Data Validation: Automatic validation of data against predefined rules or expectations.
- Data Profiling: Analyzing and profiling data to gain insights into its quality and characteristics.
- Data Governance: Enforcing data governance policies and ensuring compliance with regulations.
- Alerting and Reporting: Notifying users when data anomalies are detected and generating detailed reports for analysis.
Data monitoring is an essential aspect of data observability. It allows organizations to keep a close eye on their data pipelines, ensuring that data flows smoothly and without any hiccups. Real-time monitoring enables the detection of anomalies or issues as they occur, allowing for immediate action to be taken. This proactive approach helps organizations maintain the integrity of their data and prevent any potential disruptions in their data-driven processes.
Data validation plays a critical role in ensuring the accuracy and reliability of data. By automatically validating data against predefined rules or expectations, organizations can identify any inconsistencies or errors in their data. This not only helps in maintaining data quality but also saves valuable time that would otherwise be spent manually checking and verifying data. With data validation, organizations can have confidence in the accuracy of their data, enabling them to make informed decisions based on reliable information.
An Introduction to Soda
Soda is a leading data observability tool that helps organizations ensure data accuracy and reliability. With its user-friendly interface and powerful features, Soda simplifies the process of monitoring and validating data in real-time.
Overview of Soda's Functionality
Soda offers a comprehensive suite of features that enable organizations to monitor, validate, and govern their data effectively:
- Real-time Data Monitoring: Soda provides real-time monitoring capabilities to detect any deviations or anomalies in data pipelines.
- Data Validation Rules: With Soda's flexible rule engine, users can define custom validation rules to check data for accuracy and completeness.
- Data Profiling and Analysis: Soda enables in-depth data profiling and analysis, allowing users to gain insights into data quality and identify patterns or trends.
- Data Governance and Compliance: Soda integrates with existing data governance frameworks to ensure data compliance and adherence to organizational policies.
- Alerting and Reporting: Soda sends real-time alerts and generates detailed reports when data anomalies are detected, enabling quick resolution of issues.
Pros and Cons of Using Soda
Soda offers several advantages as a data observability tool, including:
- User-Friendly Interface: Soda's intuitive interface makes it easy for users to navigate and leverage the tool's functionalities without extensive technical knowledge.
- Flexible Rule Engine: The flexible rule engine allows users to define custom validation rules, adapting to the specific requirements of their data pipelines.
- Comprehensive Monitoring: Soda provides real-time monitoring capabilities, ensuring that data issues are detected as soon as they occur.
However, it's important to note that Soda may have some limitations, such as:
- Scalability: While Soda is suitable for small to medium-sized organizations, it may face scalability challenges when dealing with large-scale data infrastructures.
- Complex Configurations: Setting up and configuring Soda's rules and monitoring may require some technical expertise and initial investment of time.
An Introduction to Monte Carlo
Monte Carlo is another popular data observability tool that helps organizations gain confidence in their data by providing proactive monitoring and validation capabilities.
Overview of Monte Carlo's Functionality
Monte Carlo offers a range of powerful features tailored for data observability:
- Data Monitoring and Error Detection: Monte Carlo continuously monitors data for inconsistencies or errors, enabling early detection of potential issues.
- Data Observability Metrics: Monte Carlo provides insightful metrics and KPIs to track data health and identify areas that require attention.
- Data Validation Workflows: Monte Carlo allows users to define validation workflows and notifications for timely resolution of data issues.
- Data Dependency Mapping: Monte Carlo helps identify data dependencies and understand the impact of changes on downstream processes.
- Collaboration and Workflow Management: Monte Carlo facilitates collaboration among data teams and streamlines workflow management.
Pros and Cons of Using Monte Carlo
Monte Carlo offers several benefits as a data observability tool:
- Proactive Error Detection: Monte Carlo's proactive monitoring capabilities help identify data issues before they impact critical processes or analyses.
- Comprehensive Metrics and KPIs: Monte Carlo provides meaningful metrics and KPIs, allowing teams to track data health and make data-driven decisions.
- Workflow Collaboration: Monte Carlo promotes collaboration among data teams, enabling streamlined workflows and faster issue resolution.
However, it's important to consider a few limitations of Monte Carlo:
- Learning Curve: Monte Carlo may have a slightly steeper learning curve compared to other data observability tools, requiring some initial investment of time and training.
- Auto-Remediation: Unlike some other tools, Monte Carlo does not offer automated remediation of data issues, and resolution still requires manual intervention.
Detailed Comparison Between Soda and Monte Carlo
Comparing User Interface and Ease of Use
Soda and Monte Carlo differ in terms of their user interface and ease of use:
Soda offers a user-friendly interface, making it accessible to users with varying technical backgrounds. It provides a visually appealing experience with intuitive navigation and easy-to-understand visualizations. This simplicity allows users to quickly familiarize themselves with the tool and leverage its functionalities effectively.
On the other hand, Monte Carlo's interface may have a slightly steeper learning curve for users who are new to the tool. However, once users become familiar with its features, Monte Carlo offers a comprehensive and customizable experience. It provides users with a wide range of metrics and visualizations to monitor and understand their data effectively.
Comparing Data Monitoring Capabilities
Both Soda and Monte Carlo excel in data monitoring, but they offer different approaches:
Soda provides real-time monitoring capabilities, detecting and alerting users to data issues as they occur. It continuously scans data pipelines for anomalies, ensuring that potential problems are identified and addressed promptly. Soda's monitoring features empower users to take proactive action to maintain data accuracy.
Monte Carlo takes a proactive approach to data monitoring. By establishing baseline expectations for data quality, Monte Carlo detects anomalies and trends that deviate from these expectations, giving users early visibility into potential issues. Monte Carlo's comprehensive monitoring capabilities enable data teams to mitigate issues before they impact downstream processes and analyses.
Comparing Alerting and Reporting Features
Both Soda and Monte Carlo offer alerting and reporting features, but with some differences:
Soda provides real-time alerts and notifications when data anomalies are detected. Its flexible alerting system allows users to customize the thresholds and criteria for alerts based on their specific requirements. Additionally, Soda generates detailed reports that provide insights into the root causes of data issues, facilitating quick resolution and preventing future occurrences.
Monte Carlo also offers alerting capabilities, notifying users when data quality thresholds are breached. While its alerting system may not provide as much flexibility as Soda's, Monte Carlo compensates with informative alerts that empower users to identify and resolve data issues promptly. Monte Carlo's reporting capabilities allow users to monitor key metrics and trends, providing a comprehensive view of data health and quality over time.
Pricing Comparison
Soda Pricing Structure
Soda's pricing structure is based on the specific needs and scale of organizations. It typically offers tiered pricing models that align with the volume of data processed or the number of users. Pricing details and specific plans can be obtained by contacting Soda's sales team directly.
Monte Carlo Pricing Structure
Similar to Soda, Monte Carlo's pricing is tailored to the requirements of each organization. It offers flexible plans that consider factors such as data volume, number of users, and additional desired features. To get detailed pricing information, it is recommended to contact Monte Carlo's sales team.
In conclusion, both Soda and Monte Carlo offer powerful solutions for data observability, enabling organizations to ensure the quality and accuracy of their data. Your choice between these two tools depends on factors such as your organization's specific needs, technical proficiency, and budget. By considering the strengths, weaknesses, and unique features of each tool, you can make an informed decision that aligns with your data observability goals. Remember to evaluate your requirements carefully and leverage trial versions or demos whenever possible to assess the suitability of each tool for your organization.While Soda and Monte Carlo offer robust solutions for data observability, the journey towards comprehensive data management doesn't end there. CastorDoc takes it a step further by integrating advanced governance, cataloging, and lineage capabilities with a user-friendly AI assistant. This powerful combination enables businesses to engage in self-service analytics and empowers users to navigate the complexities of data with ease. Whether you're looking to streamline your data governance lifecycle or make data more accessible to business users, CastorDoc is designed to revolutionize your data strategy. To discover how CastorDoc complements tools like Soda and Monte Carlo, and to explore more tool comparisons, check out more tools comparisons here.
You might also like
Get in Touch to Learn More
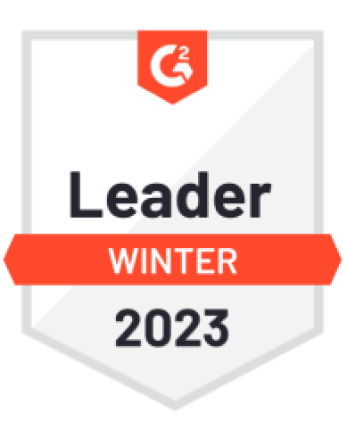
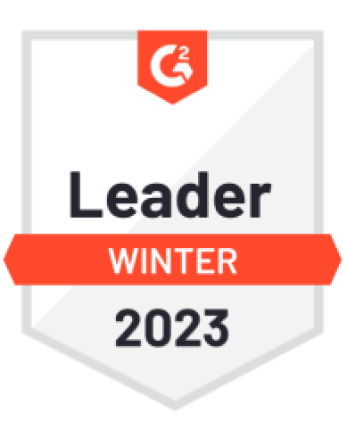

“[I like] The easy to use interface and the speed of finding the relevant assets that you're looking for in your database. I also really enjoy the score given to each table, [which] lets you prioritize the results of your queries by how often certain data is used.” - Michal P., Head of Data