Data Observability Tool Comparison: Datafold vs. Acceldata
In today's data-driven world, the need for robust data observability tools has become paramount. Organizations must ensure the accuracy, reliability, and integrity of their data to make informed business decisions. Two popular tools that have emerged in this space are Datafold and Acceldata. In this article, we will provide a thorough comparison of these two tools, exploring their features, pros and cons, and ultimately help you in making the right choice for your business.
Understanding Data Observability
Data observability refers to the ability to measure, monitor, and ensure the quality and reliability of data in real-time. It involves continuous data validation, monitoring, and anomaly detection to identify and rectify issues before they impact critical business processes. Data observability tools play a crucial role in enabling organizations to gain insights and make data-driven decisions with confidence.
Implementing data observability practices involves setting up automated processes that continuously monitor data quality metrics, such as completeness, accuracy, and timeliness. By leveraging advanced algorithms and machine learning models, organizations can proactively detect anomalies and deviations from expected patterns, allowing them to take corrective actions promptly. This proactive approach not only enhances data reliability but also boosts operational efficiency by reducing the time and effort required to troubleshoot data issues.
The Importance of Data Observability
Data observability is vital for organizations operating in dynamic environments where data volumes are growing rapidly. By ensuring data accuracy and reliability, businesses can mitigate risks, improve operational efficiency, enhance data product development, and increase customer satisfaction. Data observability helps maintain data integrity while enabling teams to identify, address, and resolve issues quickly.
Furthermore, data observability plays a critical role in regulatory compliance, particularly in industries such as finance, healthcare, and telecommunications, where data privacy and security regulations are stringent. By implementing robust data observability practices, organizations can ensure that their data processing activities comply with relevant laws and standards, minimizing the risk of non-compliance penalties and reputational damage.
Key Features of Data Observability Tools
Data observability tools offer a range of features to help organizations maintain a high level of data quality. These features typically include data monitoring, data validation, anomaly detection, data lineage tracking, and data quality profiling. By providing comprehensive visibility into the data lifecycle, observability tools empower organizations to identify issues, track changes, and ensure data integrity.
In addition to the core features, advanced data observability tools may also incorporate predictive analytics capabilities, allowing organizations to forecast potential data quality issues and take preemptive measures to prevent them. By leveraging predictive analytics, businesses can anticipate data anomalies based on historical patterns and trends, enabling them to proactively address underlying issues before they escalate and impact business operations.
An Introduction to Datafold
Datafold is a leading data observability platform that aims to simplify the process of data validation and monitoring. It offers a comprehensive suite of features designed to help organizations maintain high-quality data. Let's take a closer look at what Datafold has to offer.
Datafold understands the importance of collaboration in the world of data engineering and analysis. That's why it provides a user-friendly interface that allows data engineers and data analysts to collaborate effectively. With Datafold, teams can easily work together, share insights, and make data-driven decisions. This collaborative environment fosters innovation and efficiency, enabling organizations to stay ahead in the fast-paced world of data.
Overview of Datafold's Features
Datafold provides automated data validation, making it easy to catch data anomalies and ensure data quality. This feature saves valuable time and effort for data teams, as they no longer have to manually check for errors or inconsistencies in their datasets. With Datafold, data validation becomes a seamless and automated process, giving teams the confidence that their data is accurate and reliable.
One of the standout features of Datafold is its ability to track data lineage. This means that users can easily understand where data comes from and how it is transformed as it moves through the pipeline. Data lineage provides valuable insights into the data's journey, allowing teams to trace any issues or discrepancies back to their source. This level of transparency and visibility is crucial for maintaining data integrity and ensuring the reliability of analysis and decision-making.
Additionally, Datafold integrates seamlessly with popular data platforms, making it a versatile tool for data observability. Whether you're using Apache Kafka, Amazon Redshift, or Google BigQuery, Datafold can easily connect to your existing data infrastructure. This compatibility ensures that you can leverage the power of Datafold without disrupting your current workflows or processes.
Pros and Cons of Using Datafold
Like any tool, Datafold has its strengths and weaknesses. One of the main advantages of using Datafold is its intuitive user interface, which simplifies the process of setting up data validation rules and monitoring workflows. The user-friendly design allows even non-technical users to navigate the platform with ease, empowering them to take control of their data quality.
The ability to track data lineage also proves valuable in understanding complex data pipelines. By visualizing the flow of data and identifying any transformations or dependencies, teams can gain a deeper understanding of their data ecosystem. This knowledge helps them identify potential bottlenecks, optimize their processes, and make informed decisions based on a solid foundation of data insights.
However, it's worth noting that some users have reported that Datafold's advanced features can be overwhelming for beginners, requiring a steep learning curve. While the platform is designed to be user-friendly, it does offer powerful capabilities that may take some time to master. However, with the right training and support, teams can unlock the full potential of Datafold and harness its advanced features to drive data excellence.
Additionally, while Datafold integrates well with popular data platforms, compatibility with niche tools may be limited. If your organization relies heavily on specialized data tools, it's important to ensure that Datafold supports those integrations before making a decision. However, for most organizations, Datafold's wide range of integrations covers the majority of their data infrastructure, making it a reliable and versatile choice for data observability.
An Introduction to Acceldata
Acceldata is another prominent data observability tool that emphasizes data quality and observability. With a comprehensive range of features, Acceldata aims to enable organizations to uncover insights, drive innovation, and ensure data reliability.
Overview of Acceldata's Features
Acceldata provides end-to-end data monitoring and validation capabilities that empower users to track data changes, detect anomalies, and validate data quality. It offers a centralized dashboard that provides real-time insights into data performance and reliability. Acceldata's robust alerting system enables timely notifications and proactive issue resolution. The tool also offers advanced data governance features, allowing organizations to define and enforce data quality standards across the board.
Pros and Cons of Using Acceldata
Acceldata offers several advantages that make it an attractive choice for organizations seeking data observability. The tool's intuitive interface ensures ease of use, allowing users to set up monitoring and validation workflows efficiently. Its powerful alerting system and data governance capabilities provide organizations with the means to maintain data quality and enforce compliance. However, some users have reported that Acceldata's customization options can be limited, and additional integration efforts may be required to leverage its full potential.
Detailed Comparison Between Datafold and Acceldata
Now that we have explored the features and capabilities of Datafold and Acceldata individually, it's time to dive deeper into their comparison. This section will evaluate specific aspects of each tool, enabling you to make an informed decision based on your requirements.
Comparing User Interface and Ease of Use
When it comes to user interface and ease of use, both Datafold and Acceldata offer intuitive platforms that facilitate efficient data validation and monitoring. However, users have reported that Datafold's interface is more approachable, especially for beginners. On the other hand, Acceldata's interface may require some familiarity with data observability concepts and customization options.
Comparing Data Monitoring Capabilities
Data monitoring is a critical aspect of data observability tools, and both Datafold and Acceldata excel in this area. Datafold's ability to track data lineage provides valuable insights into how data transforms throughout the pipeline. Acceldata, on the other hand, offers a centralized dashboard that presents real-time data performance and reliability metrics. Ultimately, the choice between the two depends on the specific needs and preferences of your organization.
Comparing Data Validation Features
Data validation is essential to ensure data accuracy and reliability. Datafold offers automated data validation capabilities, simplifying the process of catching data anomalies. Acceldata, on the other hand, provides end-to-end data validation, enabling users to track data changes and validate data quality comprehensively. Deciding which tool suits your requirements depends on the complexity and depth of data validation you need.
Comparing Pricing Models
When it comes to pricing, Datafold offers a subscription-based model, allowing organizations to scale their usage based on their needs. Acceldata, on the other hand, adopts a customized pricing approach based on specific requirements and usage. It is recommended to evaluate the pricing models of both tools in conjunction with your organization's budgetary guidelines.
Making the Right Choice for Your Business
Now that we have compared Datafold and Acceldata extensively, it's time to make the right choice for your business. To ensure you select the ideal tool for your organization, consider the following factors:
Assessing Your Business Needs
Every organization has unique requirements when it comes to data observability. Assess your business needs, data volumes, and specific use cases to understand which tool aligns better with your objectives. Consider factors such as team size, data complexity, and existing data infrastructure.
Evaluating Tool Performance Against Your Requirements
Test both Datafold and Acceldata extensively by running them against your data pipelines. Evaluate their performance in terms of data monitoring, validation, and ease of use. Determine which tool integrates seamlessly with your existing data ecosystem and provides the required level of observability.
Considering Future Scalability and Support
As your organization grows, your data observability needs are likely to evolve. Consider the scalability of both tools and their ability to grow with your organization. Additionally, evaluate the support provided by each vendor, including documentation, customer support, and community engagement.
By carefully considering these factors, you will be empowered to make an informed decision and select the right data observability tool for your business.
Conclusion
In this article, we explored the realm of data observability tools, focusing on the comparison between Datafold and Acceldata. Both tools offer robust features designed to ensure data accuracy, reliability, and integrity. While Datafold excels in terms of its user-friendly interface and data lineage tracking, Acceldata stands out with its comprehensive monitoring and validation capabilities. By assessing your organization's specific requirements and evaluating each tool's performance, you can make an informed decision that aligns with your business objectives. Remember, data observability is essential for gaining actionable insights and making informed decisions, so choose your tool wisely.
While Datafold and Acceldata offer compelling solutions for data observability, CastorDoc takes a holistic approach to data management, combining advanced governance, cataloging, and lineage capabilities with an AI-driven user experience. Whether you're part of a data team looking to streamline governance and compliance or a business user seeking to harness data for strategic insights, CastorDoc's intuitive AI assistant and conversational interface make it a powerful ally in enabling self-service analytics. To discover how CastorDoc compares to other tools and how it can elevate your organization's data capabilities, check out more tools comparisons here.
You might also like
Get in Touch to Learn More
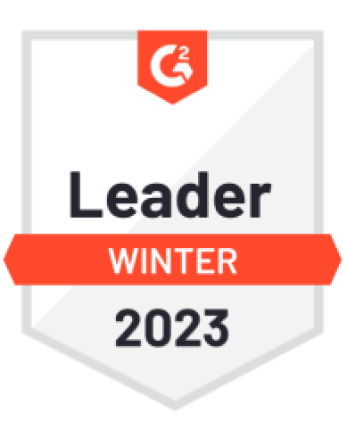
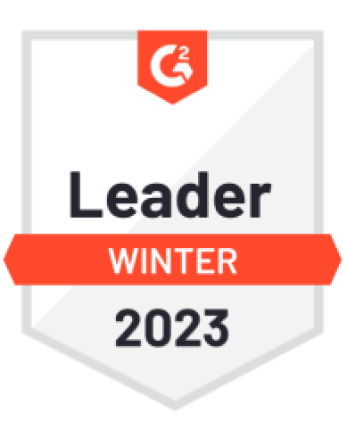

“[I like] The easy to use interface and the speed of finding the relevant assets that you're looking for in your database. I also really enjoy the score given to each table, [which] lets you prioritize the results of your queries by how often certain data is used.” - Michal P., Head of Data