Data Observability Tool Comparison: great expectations vs. Lantern
In the realm of data management, the need for observability has become paramount. Without proper data observability, organizations risk making critical decisions based on flawed or incomplete information. To address this challenge, a variety of data observability tools have emerged to help analysts and data engineers ensure the quality and reliability of their data. Two such tools are Great Expectations and Lantern. In this article, we will compare and contrast these two tools, examining their key features, advantages, and limitations, to help you make an informed decision about which tool best suits your organization's needs.
Understanding Data Observability
Data observability is the practice of monitoring, measuring, and ensuring the quality and accuracy of data throughout its lifecycle. It involves proactively detecting and addressing issues such as data drift, missing values, schema inconsistencies, and other anomalies that could compromise data reliability and integrity. Data observability empowers organizations to have confidence in their data, enabling them to make informed decisions and prevent downstream errors.
One key aspect of data observability is the concept of data lineage. Data lineage refers to the ability to trace the origin and transformation of data as it moves through various systems and processes. By establishing a clear data lineage, organizations can understand how data is generated, manipulated, and consumed, which is essential for ensuring data quality and compliance.
The Importance of Data Observability
Effective data observability is crucial for any data-driven organization. With the exponential growth of data sources and the increasing complexity of data pipelines, ensuring the quality and trustworthiness of data has become a formidable challenge. Without proper data observability, organizations risk making decisions based on inaccurate or misleading data, leading to costly mistakes, missed opportunities, and damaged reputation.
Furthermore, data observability plays a vital role in regulatory compliance and risk management. In industries such as finance, healthcare, and e-commerce, where data privacy and security are paramount, having visibility into data quality and integrity is essential for meeting compliance requirements and mitigating potential risks.
Key Components of Data Observability
Data observability comprises several key components that together provide a comprehensive view of data quality and reliability. These components include data validation, anomaly detection, data monitoring, and proactive alerting. By implementing robust data observability practices, organizations can identify and resolve data issues before they propagate throughout the system, ensuring the accuracy and consistency of their data.
Another critical component of data observability is metadata management. Metadata, which provides information about the structure, content, and context of data, is essential for understanding data assets and ensuring their proper usage. By effectively managing metadata, organizations can improve data discoverability, lineage tracking, and governance, ultimately enhancing the overall observability of their data ecosystem.
Introduction to Great Expectations
Great Expectations is a powerful open-source framework designed to help data teams validate, document, and monitor data pipelines. It allows users to define expectations, which are assertions about the data, and automatically validate those expectations against incoming data, generating detailed reports and alerts when discrepancies are detected. This enables data teams to gain insights into data quality issues, identify root causes, and proactively address them.
Features of Great Expectations
Great Expectations offers a wide range of features to support robust data observability:
- Data Validation: Great Expectations provides a flexible and extensible way to define and validate expectations for data quality, including schema verification, column value range checks, and uniqueness constraints.
- Data Documentation: With Great Expectations, data documentation becomes an integral part of the data pipeline. It enables users to document expectations, assumptions, and data profiles, ensuring better understanding and collaboration among data stakeholders.
- Data Monitoring: Great Expectations allows for continuous monitoring of data quality, generating reports and notifications whenever expectations are violated. This provides early detection of issues and helps maintain data integrity over time.
Pros and Cons of Great Expectations
Like any tool, Great Expectations has its pros and cons:
- Pros:
- Open-source and widely adopted, with an active and supportive community.
- Flexible and customizable, allowing users to define and validate expectations based on their specific requirements.
- Offers powerful data documentation capabilities, facilitating collaboration and knowledge sharing among data stakeholders.
- Cons:
- Steep learning curve, especially for users new to the framework.
- Limited native integrations with certain data storage platforms.
- Requires coding skills to configure and customize expectations.
Introduction to Lantern
Lantern is a data observability platform that provides end-to-end visibility and control over data quality. It offers a range of features designed to help organizations ensure the accuracy and reliability of their data throughout the entire data lifecycle. With Lantern, data teams can easily monitor data quality, detect anomalies, and optimize data pipelines to deliver high-quality data to downstream applications and users.
Features of Lantern
Lantern offers several notable features to support data observability:
- Data Quality Monitoring: Lantern provides real-time monitoring of critical data quality metrics, allowing users to track data health and identify issues promptly. It offers customizable dashboards and customizable alerts to ensure timely notification and action.
- Anomaly Detection: With Lantern's advanced anomaly detection algorithms, users can proactively detect data anomalies and outliers. This helps organizations identify issues early and prevent them from propagating through the system.
- Data Pipeline Optimization: Lantern enables users to analyze and optimize data pipelines, identifying bottlenecks and inefficiencies that could impact data quality. By fine-tuning pipelines, organizations can ensure a smooth flow of high-quality data.
Pros and Cons of Lantern
Let's examine the pros and cons of using Lantern for data observability:
- Pros:
- Comprehensive and user-friendly interface, making it easy to set up and manage data observability processes.
- Advanced anomaly detection capabilities, allowing for early identification of data issues.
- Supports integrations with various data storage platforms, enabling seamless data connectivity.
- Cons:
- Relatively new to the market, with a smaller user base compared to Great Expectations.
- Less customization options compared to Great Expectations, limiting flexibility for specific use cases.
- Requires a subscription and may incur additional costs based on usage and data volume.
In-depth Comparison
Ease of Use: Great Expectations vs. Lantern
When it comes to ease of use, both Great Expectations and Lantern offer user-friendly interfaces that simplify data observability tasks. Great Expectations offers a command-line interface (CLI) and a Python API, allowing users to define expectations using a familiar programming language. Lantern, on the other hand, provides a web-based interface that requires minimal coding, enabling users to perform data observability tasks without extensive technical knowledge.
While both tools aim to make data observability accessible to users of varying skill levels, Great Expectations may have a steeper learning curve, particularly for those new to the framework. Its flexibility and extensibility come at the cost of additional configuration and setup. In contrast, Lantern's streamlined interface and intuitive workflows make it easier for users to get started quickly, without sacrificing essential features.
Performance: Great Expectations vs. Lantern
In terms of performance, both Great Expectations and Lantern offer robust capabilities for data observability. Great Expectations excels in its ability to validate large volumes of data efficiently, thanks to its optimized execution engine. It leverages parallelization and distributed computing to process expectations in parallel, enabling high-speed validation across multiple data sources.
Lantern, on the other hand, focuses not only on data validation but also on real-time monitoring and anomaly detection. Its advanced algorithms analyze data streams in real-time, allowing for timely alerts and proactive issue resolution. While Lantern may have slightly lower performance compared to Great Expectations in terms of processing large volumes of data, its emphasis on real-time monitoring and anomaly detection offers valuable insights into data quality.
Scalability: Great Expectations vs. Lantern
Scalability is a crucial consideration when choosing a data observability tool, especially for organizations dealing with massive amounts of data. Great Expectations is built to handle scalability challenges, allowing users to scale horizontally by distributing expectations across multiple nodes. This enables efficient processing of large-scale data pipelines and accommodates growing data volumes.
Lantern is designed with scalability in mind, providing users with the ability to adapt to evolving data requirements. Its cloud-based architecture allows for seamless scaling by leveraging underlying cloud infrastructure. With Lantern, organizations can easily expand their data observability processes as their data volumes and complexity increase.
Pricing Analysis
Cost of Great Expectations
Great Expectations is an open-source framework, meaning that the software itself is free to use without any licensing fees. However, like any tool, there are associated costs, such as the investment in time and resources for implementation, configuration, and maintenance. Additionally, organizations may need to allocate resources to train their data teams on how to effectively use Great Expectations to maximize its benefits.
Cost of Lantern
Lantern operates under a subscription-based pricing model. The cost of Lantern varies depending on factors such as data volume, usage, and additional features required. Organizations interested in using Lantern for data observability should consult with the Lantern team to determine the specific pricing details and packages that best suit their needs.
It's important to consider the total cost of ownership, including not only the upfront costs but also ongoing maintenance, support, and potential integration expenses when evaluating the affordability of these tools.
Conclusion
Both Great Expectations and Lantern offer robust solutions for data observability, empowering organizations to detect and address data quality issues effectively. Great Expectations excels in its flexibility, extensibility, and integration with existing data pipelines. On the other hand, Lantern's user-friendly interface, advanced anomaly detection, and focus on real-time monitoring make it an excellent choice for organizations seeking a comprehensive and scalable data observability platform.
Ultimately, the choice between Great Expectations and Lantern depends on your organization's specific needs, technical expertise, and long-term data observability goals. By carefully considering the features, pros and cons, and pricing aspects of each tool, you can confidently select the one that aligns best with your data management strategy, ensuring the quality and reliability of your data throughout its lifecycle.
As you consider the best data observability tools for your organization, remember that the journey doesn't end there. CastorDoc offers a seamless extension to your data management capabilities, integrating advanced governance, cataloging, and lineage features with a user-friendly AI assistant. It's the perfect companion for businesses looking to enable self-service analytics and maintain data quality across the board. CastorDoc empowers your data teams with complete control and visibility, while also providing business users with the intuitive tools they need to find and utilize data effectively. Ready to explore how CastorDoc can complement tools like Great Expectations and Lantern in your modern data stack? Check out more tools comparisons here and discover the transformative power of CastorDoc for your enterprise.
You might also like
Get in Touch to Learn More
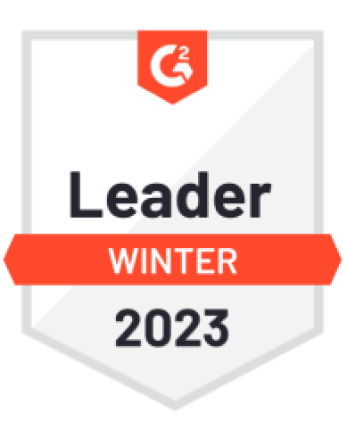
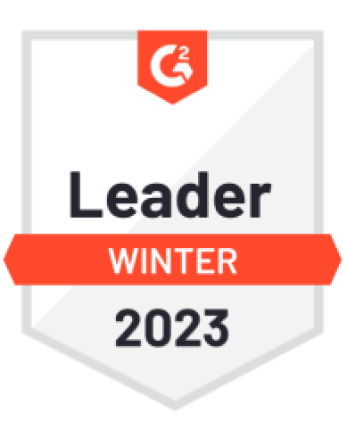

“[I like] The easy to use interface and the speed of finding the relevant assets that you're looking for in your database. I also really enjoy the score given to each table, [which] lets you prioritize the results of your queries by how often certain data is used.” - Michal P., Head of Data