Data Observability Tool Comparison: great expectations vs. Anomalo
In the world of data analysis and management, ensuring the quality and reliability of your data is of paramount importance. This is where Data Observability comes into play. Data Observability is the practice of monitoring and maintaining data quality to ensure that it meets specific standards and remains consistent over time. To support this practice, there are several tools available in the market, and two of the most popular ones are Great Expectations and Anomalo. In this article, we will compare these two tools in detail to help you make an informed decision for your business.
Understanding Data Observability
Data Observability is a relatively new concept, but it has gained significant attention in recent years. It refers to the ability to understand, monitor, and validate data in order to make informed decisions based on reliable information. This practice involves implementing various techniques and tools to ensure the completeness, accuracy, and consistency of data throughout its lifecycle.
The Importance of Data Observability
Data Observability is important for several reasons. First and foremost, it ensures that the data used for decision making is accurate and reliable. Inaccurate data can lead to incorrect conclusions and potentially disastrous outcomes. By implementing Data Observability practices and tools, organizations can mitigate the risks associated with data quality issues.
Additionally, Data Observability helps organizations proactively identify and resolve data issues before they impact business operations. It enables data teams to monitor the health of their data pipelines, identify anomalies, and intervene when necessary. This proactive approach saves time and resources, as data issues can be addressed and resolved quickly, minimizing their impact on downstream processes.
Key Components of Data Observability
Data Observability comprises various components. These include data validation, data profiling, data lineage, and data monitoring. Let's briefly discuss each of these components:
- Data Validation: This component involves checking the integrity and quality of data by applying a set of predefined rules or expectations. It helps ensure that the data meets specific criteria and requirements.
- Data Profiling: Data profiling refers to the analysis of data to understand its characteristics, such as data types, distributions, and patterns. It helps in identifying anomalies and outliers, which can indicate data quality issues.
- Data Lineage: Data lineage provides a detailed record of the origins, transformations, and movements of data within a system or across multiple systems. It helps in understanding the data's journey and enables traceability.
- Data Monitoring: This component involves continuously monitoring data flows, processes, and systems to identify potential issues or anomalies. It allows organizations to take proactive measures to ensure data quality and reliability.
Introduction to Great Expectations
Great Expectations is an open-source platform that specializes in Data Observability. It provides a comprehensive set of tools and functionalities to help organizations ensure the quality and reliability of their data. Let's explore some of its key features:
Features of Great Expectations
Great Expectations offers a wide range of features that make it a powerful tool for data teams. Some of its notable features include:
- Data Validation: Great Expectations allows you to define and enforce data validation rules on your datasets, ensuring that the data meets specific expectations. It provides a flexible and customizable framework for expressing these rules.
- Data Profiling: With Great Expectations, you can easily generate data profiles that summarize the properties of your datasets. It helps you gain insights into the data's characteristics and identify any potential quality issues.
- Data Documentation: Great Expectations enables you to document your data pipelines and expectations, making it easier for data teams to collaborate and share knowledge. It provides a centralized repository for all your data documentation needs.
- Data Testing: Great Expectations allows you to write unit tests for your data, similar to how you would write tests for your code. These tests validate the expectations you have defined for your datasets, ensuring data correctness.
Pros and Cons of Great Expectations
Like any tool, Great Expectations has its pros and cons. Let's take a look at some of them:
Pros:
- Open-source and free to use, reducing the barrier to entry for organizations of all sizes.
- Extensive documentation and a supportive community, making it easy to get started and seek help when needed.
- Flexible and customizable, allowing data teams to define their own rules and expectations.
- Integration with popular data technologies such as Apache Spark and Pandas.
Cons:
- Steep learning curve for beginners, especially if they are not familiar with the command-line interface.
- Limited support for certain data storage systems, which may require additional customization.
- Less mature compared to some commercial data observability tools in terms of features and user experience.
Introduction to Anomalo
Anomalo is another data observability tool that aims to simplify the process of ensuring data quality and reliability. Let's explore its key features:
Features of Anomalo
Anomalo offers a unique set of features that make it a strong contender in the data observability space. Here are some of its noteworthy features:
- Data Anomaly Detection: Anomalo uses advanced machine learning algorithms to automatically detect anomalies in your data. It alerts you when it identifies any deviations from the expected patterns or distributions.
- Data Quality Metrics: Anomalo provides pre-defined data quality metrics that enable you to assess the quality of your data easily. It helps you understand the health of your datasets at a glance.
- Data Drift Analysis: With Anomalo, you can track the changes in your data over time and analyze the impact of those changes on your business operations. It helps in identifying potential data drift and its consequences.
- Collaboration and Communication: Anomalo offers features that facilitate collaboration and communication among data teams. It provides a centralized platform where team members can discuss and address data-related issues.
Pros and Cons of Anomalo
Let's take a closer look at the pros and cons of Anomalo:
Pros:
- Advanced anomaly detection capabilities powered by machine learning algorithms.
- User-friendly interface and intuitive workflows, making it accessible to both technical and non-technical users.
- Strong focus on collaboration and communication, fostering teamwork and knowledge sharing.
- Integration with popular data storage systems such as Amazon S3 and Google BigQuery.
Cons:
- Relatively higher pricing compared to some other data observability tools in the market.
- Potential limitations in terms of customization and flexibility, which may hinder specific use cases.
- Less support and community compared to more established open-source tools like Great Expectations.
Detailed Comparison Between Great Expectations and Anomalo
Comparison of Features
Both Great Expectations and Anomalo offer robust features for data observability, but they have some differences in terms of their focus and capabilities. Here's a detailed comparison of their features:
Data Validation: Great Expectations provides a flexible framework for defining and enforcing data validation rules, while Anomalo focuses more on automated anomaly detection.
Data Profiling: Great Expectations enables data profiling and offers various data insights, while Anomalo provides pre-defined data quality metrics.
Data Documentation: Great Expectations offers comprehensive data documentation capabilities, while Anomalo focuses on collaboration and communication among data teams.
Data Testing: Great Expectations allows you to write data tests, while Anomalo focuses more on detecting anomalies.
Comparison of User Experience
In terms of user experience, both tools offer intuitive interfaces and workflows. Great Expectations requires some level of technical expertise, especially when using the command-line interface. Anomalo, on the other hand, caters to both technical and non-technical users, offering a more user-friendly experience.
Comparison of Pricing
When it comes to pricing, Great Expectations stands out as an open-source tool that is free to use. Anomalo, however, follows a subscription-based model, which may involve higher costs depending on your requirements. It's essential to consider your budget and specific needs when comparing the pricing of these tools.
Making the Right Choice for your Business
Factors to Consider When Choosing a Data Observability Tool
When selecting a data observability tool for your business, several factors come into play. Here are some key considerations:
- Requirements: Assess your specific requirements, including the volume, variety, and velocity of your data. Consider the features and capabilities that align with your needs.
- Integration: Evaluate how well the tool integrates with your existing data infrastructure and workflows. Look for compatibility with your preferred data storage systems and technologies.
- Ease of Use: Consider the technical expertise required to use the tool effectively. Ensure that the tool's interface and workflows align with your team's skills and resources.
- Scalability: Evaluate the tool's scalability and performance capabilities. Assess its ability to handle increasing data volumes and growing business needs.
- Budget: Consider the cost implications of the tool, including both initial setup costs and ongoing subscription fees. Ensure that the tool provides value for money and aligns with your budget constraints.
Assessing Your Business Needs
Before making a decision, it's crucial to assess your business needs and priorities. Take into account your data management goals, compliance requirements, and the unique challenges you face. Consider factors such as data quality issues, compliance regulations, and the impact of data inaccuracies on your business operations. By thoroughly evaluating your business needs, you can make an informed choice that aligns with your objectives.
In conclusion, both Great Expectations and Anomalo offer strong capabilities for Data Observability. Great Expectations excels in providing flexibility and customization, while Anomalo focuses on automated anomaly detection and collaboration. By considering factors such as features, user experience, pricing, and your business needs, you can make the right choice for your organization. Remember, implementing a robust Data Observability tool is an investment that ensures the quality, reliability, and trustworthiness of your data, enabling you to make informed decisions and drive business success.
As you consider the right Data Observability tool for your organization, remember that the journey doesn't end with anomaly detection and data validation. CastorDoc offers a holistic approach to data management, integrating advanced governance, cataloging, and lineage capabilities with a user-friendly AI assistant to enable self-service analytics. Whether you're looking to enhance your data team's efficiency or empower your business users with intuitive data accessibility, CastorDoc provides a comprehensive solution that caters to all aspects of the data governance lifecycle. To explore how CastorDoc compares with other tools and how it can elevate your data strategy, check out more tools comparisons here.
You might also like
Get in Touch to Learn More
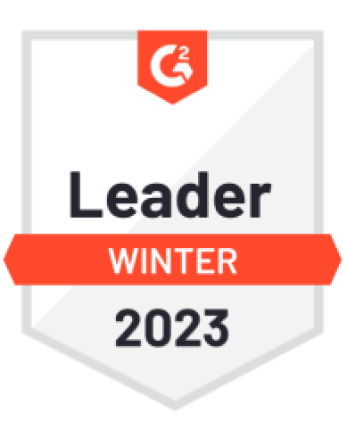
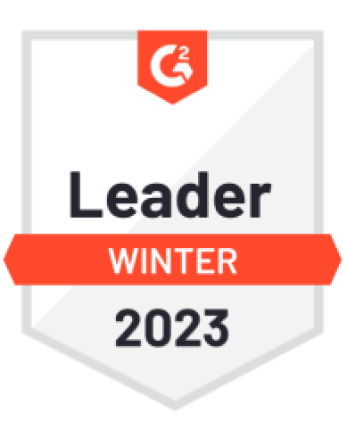

“[I like] The easy to use interface and the speed of finding the relevant assets that you're looking for in your database. I also really enjoy the score given to each table, [which] lets you prioritize the results of your queries by how often certain data is used.” - Michal P., Head of Data