Data Observability Tool Comparison: great expectations vs. Datafold
In the world of data analysis and management, data observability has become a crucial aspect for ensuring accurate and reliable insights. Two popular tools that enable data observability are Great Expectations and Datafold. In this article, we will delve into the features, pros and cons of these tools and provide a detailed comparison to help you choose the right one for your needs.
Understanding Data Observability
Data observability is the practice of ensuring data quality, reliability, and integrity throughout the entire data lifecycle. It involves monitoring, validating, and maintaining trust in data pipelines and workflows. With the increasing complexity of data systems and the growing need for data-driven decision-making, data observability has become a fundamental requirement for organizations.
The Importance of Data Observability
Data observability is essential for several reasons. Firstly, it helps identify and address data quality issues before they impact business operations. By detecting anomalies, inconsistencies, and errors, organizations can proactively take corrective measures and minimize the impact on decision-making processes.
Secondly, data observability increases confidence in the data being used for analysis. It enables data professionals to establish trust and transparency, ensuring that the insights derived from the data are accurate and reliable.
Lastly, data observability promotes collaboration and knowledge sharing among teams. With a unified understanding of data quality expectations and metrics, teams can work together more effectively and make informed decisions based on data.
Key Components of Data Observability
Several components contribute to a robust data observability framework:
- Data Monitoring: Continuous monitoring of data pipelines and workflows to detect and alert on anomalies, data drift, schema changes, and other issues.
- Data Validation: Validation of data against predefined expectations, rules, or constraints to ensure its compliance with the expected quality standards.
- Data Profiling: Profiling and analyzing data to gain insights into its structure, distribution, and statistical properties. This helps in understanding data anomalies and identifying data quality issues.
- Data Documentation: Documentation of data schema, lineage, and metadata to facilitate understanding and collaboration among data teams.
Data observability goes beyond these key components. It also involves data lineage tracking, which enables organizations to trace the origin and transformation of data throughout its lifecycle. This information is crucial for regulatory compliance, data governance, and ensuring data accuracy.
Another important aspect of data observability is data anomaly detection. By leveraging advanced algorithms and machine learning techniques, organizations can automatically identify unusual patterns or outliers in the data. This helps in detecting potential data quality issues or anomalies that may require further investigation.
Furthermore, data observability encompasses data governance practices. It involves defining data quality standards, establishing data ownership, and implementing data access controls. These measures ensure that data is managed and used in a secure and compliant manner.
In conclusion, data observability is a critical practice for organizations that rely on data for decision-making. By implementing a comprehensive data observability framework, organizations can ensure the quality, reliability, and integrity of their data, enabling them to make informed decisions and drive business success.
Introduction to Great Expectations
Great Expectations is an open-source framework designed to help data teams implement data observability practices. It provides a suite of tools and libraries to define, document, and validate data expectations across various data sources and data workflows.
Features of Great Expectations
Great Expectations offers several features that make it a powerful data observability tool:
- Data Expectation Management: Great Expectations allows you to define and document expectations about your data, such as data types, value ranges, and uniqueness constraints.
- Data Profiling: You can use Great Expectations to profile your data, understand its statistical properties, and identify anomalies or data quality issues.
- Data Validation: Great Expectations provides built-in capabilities for validating data against defined expectations. It alerts you when the data does not meet the expected criteria.
- Data Documentation: With Great Expectations, you can generate documentation about your data expectations, making it easier for teams to collaborate and understand the data.
Pros and Cons of Great Expectations
Like any tool, Great Expectations has its advantages and disadvantages:
On the positive side:
- Open-Source: As an open-source tool, Great Expectations is accessible and customizable to meet various data observability needs.
- Extensive Documentation: Great Expectations provides comprehensive documentation and guides that make it easier to get started and utilize its features effectively.
- Community Support: With a growing community of contributors, Great Expectations benefits from continuous improvement and support from the user community.
However, there are a few drawbacks worth mentioning:
- Learning Curve: While Great Expectations offers extensive documentation, getting up to speed and fully utilizing its capabilities may require some time and effort.
- Complex Configurations: Configuring and fine-tuning Great Expectations for specific data workflows can be challenging, especially for users who are new to the tool.
Introduction to Datafold
Datafold is a cloud-native data observability platform that aims to help data teams monitor, validate, and understand their data pipelines effortlessly. It provides real-time insights into data quality and performance, empowering organizations to make data-driven decisions confidently.
Features of Datafold
Datafold offers a range of features to enhance data observability:
- Data Monitoring: Datafold continuously monitors data pipelines, alerting users to any anomalies or deviations from expected data quality metrics.
- Data Validation: With built-in validation rules and metrics, Datafold helps validate data against predefined expectations and business rules.
- Data Anomaly Detection: Datafold uses statistical analysis and machine learning to detect anomalies and outliers in data, enabling early detection of data quality issues.
- Data Lineage: Datafold tracks and documents the lineage of data, allowing users to understand how data is transformed and derived across different stages of the pipeline.
Pros and Cons of Datafold
Let's look at the advantages and disadvantages of Datafold:
Here are the advantages:
- User-Friendly Interface: Datafold offers a user-friendly interface that makes it easy to set up, configure, and monitor data pipelines.
- Automated Insights: The platform provides automated insights into data quality, making it easier to identify issues and take corrective measures.
- Advanced Anomaly Detection: Datafold leverages advanced techniques, such as statistical modeling and machine learning, for accurate anomaly detection.
However, there are a few drawbacks:
- Pricing: Datafold is a commercial product with tiered pricing plans, which may be a constraint for organizations with limited budgets.
- Customization Limitations: While Datafold offers a range of features, its customization options may not be as extensive as open-source tools like Great Expectations.
Detailed Comparison between Great Expectations and Datafold
Comparison of Features
While both Great Expectations and Datafold offer data observability capabilities, there are notable differences in their feature sets:
- Data Expectations: Great Expectations provides a comprehensive framework to define and validate complex data expectations, while Datafold focuses more on monitoring and automated insights.
- Data Documentation: Great Expectations excels in generating comprehensive data documentation, making it easier for teams to collaborate. Datafold, on the other hand, emphasizes real-time monitoring and anomaly detection.
- Data Lineage: Datafold offers advanced data lineage capabilities, allowing users to track and understand how data is transformed across pipelines. Great Expectations provides limited support for data lineage.
Ultimately, the choice between the two tools depends on the specific needs and priorities of your organization.
Comparison of User Experience
In terms of user experience, both tools aim to provide user-friendly interfaces and intuitive workflows. Great Expectations' extensive documentation and community support ensure that users can effectively leverage its features, although it may require a learning curve for beginners. On the other hand, Datafold offers a more streamlined and guided experience, simplifying the setup and configuration process.
Comparison of Performance
In terms of performance, both tools deliver reliable data observability capabilities. Great Expectations, being an open-source tool, can be fine-tuned to suit specific performance requirements and infrastructure setups. Datafold's cloud-native architecture provides scalability, real-time monitoring, and automated insights, ensuring efficient performance for monitoring data pipelines.
Choosing the Right Data Observability Tool
Factors to Consider
When selecting a data observability tool, consider the following factors:
- Business Needs: Evaluate your specific data observability requirements, such as the need for complex data expectations, extensive data documentation, or advanced anomaly detection.
- Team Expertise: Assess the skill set and familiarity of your data team with the tools under consideration. Consider the learning curve and ease of adoption for each option.
- Scalability: If your data pipelines are expected to scale significantly, consider the scalability and performance aspects of the tools.
- Cost: Evaluate the pricing models and licensing options for the tools, ensuring that they align with your budget and overall cost considerations.
Making an Informed Decision
Ultimately, the choice between Great Expectations and Datafold depends on your specific needs and priorities. Consider conducting a proof of concept (POC) or pilot project using both tools to evaluate their functionality, integration capabilities, and usability within your organization. Engage with the respective communities, seek user reviews, and gather feedback from stakeholders to make an informed decision.
Remember that data observability is an ongoing process, and regularly reassessing your tooling choices based on evolving requirements and developments in the field is recommended.
In conclusion, both Great Expectations and Datafold provide valuable capabilities for data observability. Assess your organization's needs, evaluate the features, pros, and cons of each tool, and choose the one that aligns best with your data management and analytics goals. By leveraging the power of data observability, you can ensure the reliability, accuracy, and trustworthiness of your data, enabling robust decision-making and driving business success.
As you consider the best data observability tools for your organization, remember that the landscape is rich with options that cater to various needs. If you're looking for a solution that not only offers advanced governance, cataloging, and lineage capabilities but also enhances user experience with a friendly AI assistant, CastorDoc might be the perfect fit. CastorDoc is designed to facilitate self-service analytics, allowing data teams to maintain control over governance while enabling business users to access and understand data with ease. To explore how CastorDoc compares to other tools in the modern data stack and to find the right balance of features for your business, check out more tools comparisons here.
You might also like
Get in Touch to Learn More
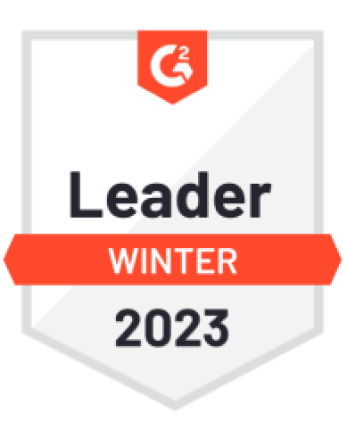
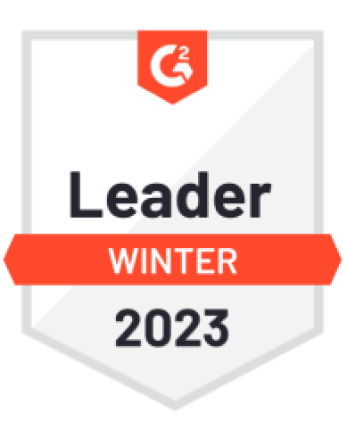

“[I like] The easy to use interface and the speed of finding the relevant assets that you're looking for in your database. I also really enjoy the score given to each table, [which] lets you prioritize the results of your queries by how often certain data is used.” - Michal P., Head of Data