Data Observability Tool Comparison: great expectations vs. Marquez
In the realm of data management and analysis, ensuring the quality and reliability of your data is paramount. To achieve this, data observability tools have emerged as an essential component of any data-driven organization's toolkit. In this article, we will explore two of the leading data observability tools: Great Expectations and Marquez. We'll delve into their features, functionalities, and compare them head-to-head to help you make an informed decision for your data needs.
Understanding Data Observability Tools
The Importance of Data Observability
Data observability is the practice of monitoring and ensuring the quality and reliability of data throughout its lifecycle. As data becomes increasingly diverse and voluminous, organizations face significant challenges in maintaining data integrity. Data observability tools provide a solution by enabling proactive data monitoring, identifying anomalies, and alerting users to potential issues. This not only helps data teams maintain trust in their data but also empowers stakeholders to make informed decisions based on reliable insights.
Ensuring data observability is crucial in today's data-driven world where decisions are heavily reliant on the accuracy and timeliness of data. Without proper monitoring and observability tools in place, organizations risk making critical decisions based on incomplete or inaccurate information. Data observability tools act as a safeguard, ensuring that data remains trustworthy and consistent, ultimately leading to more successful outcomes and informed strategies.
Key Features of Data Observability Tools
Data observability tools offer a range of features designed to tackle the complex task of ensuring data quality. These features typically include data profiling, data validation, data documentation, data lineage tracking, and anomaly detection. By leveraging these capabilities, organizations can gain deeper insights into their data and drive data-driven decision-making.
Data profiling within observability tools involves analyzing the structure and content of data to identify patterns, inconsistencies, and outliers. This process is essential for understanding the quality of data and uncovering potential issues that may impact analysis or decision-making. Additionally, data lineage tracking allows organizations to trace the origins and transformations of data, providing transparency and accountability in data processes. By having a clear understanding of how data moves through systems, organizations can ensure data accuracy and compliance with regulations.
Introduction to Great Expectations
Overview of Great Expectations
Great Expectations is an open-source data observability tool that provides a framework for defining, documenting, and validating data expectations. It enables users to create automated tests against data, ensuring that it meets predefined expectations. Great Expectations adopts a code-driven approach, making it highly flexible and scalable for diverse data environments. With its comprehensive suite of functionalities, Great Expectations has gained popularity among data engineers and scientists for its ability to promote collaborative data development.
One of the key strengths of Great Expectations lies in its ability to facilitate seamless integration with various data platforms and tools commonly used in the industry. Whether working with traditional relational databases, cloud-based data warehouses, or big data frameworks, Great Expectations offers compatibility and adaptability to meet the unique needs of different data ecosystems. This versatility allows organizations to leverage their existing infrastructure while enhancing data quality and reliability through automated testing and validation processes.
Core Functionalities of Great Expectations
At the heart of Great Expectations are the core functionalities that define its effectiveness as a data observability tool. These functionalities include data profiling to assess data characteristics, automated data validation to ensure data meets defined standards, data documentation to provide transparency and context, and data lineage tracking to trace data transformations and dependencies. Together, these functionalities empower users to establish and maintain data quality expectations with ease.
Moreover, Great Expectations offers extensibility through custom plugins and integrations, allowing users to tailor the tool to their specific requirements and workflows. By enabling the creation of custom validation rules, data connectors, and reporting mechanisms, Great Expectations empowers organizations to address unique data quality challenges effectively. This flexibility not only enhances the tool's adaptability but also encourages innovation and experimentation in data quality management practices.
Introduction to Marquez
Overview of Marquez
Marquez is an open-source metadata service that provides a unified view of data pipelines, making it a powerful tool for data discovery and observability. Marquez focuses on capturing and documenting metadata about the flow of data within an organization, enabling users to track data lineage and dependencies across various systems. By offering a clear understanding of data provenance, Marquez empowers data teams to effectively manage and validate their data infrastructure.
Understanding the importance of data lineage is crucial in today's data-driven world. Data lineage not only helps in tracing the origins of data but also assists in identifying potential bottlenecks or issues within the data pipeline. Marquez excels in providing a visual representation of data lineage, allowing users to gain insights into how data flows through their systems, from source to destination.
Core Functionalities of Marquez
Marquez offers a set of core functionalities that are instrumental in achieving data observability. It enables the capture of metadata related to data sources, processing jobs, and datasets, providing a comprehensive overview of data lineage. Marquez also supports versioning of datasets, making it easier to track changes and ensure reproducibility. Furthermore, Marquez integrates with existing data tools and platforms, facilitating seamless adoption within data ecosystems.
One of the key features of Marquez is its ability to automate metadata management tasks, reducing the manual effort required to maintain metadata records. This automation not only saves time but also ensures consistency and accuracy in metadata documentation. Additionally, Marquez's metadata repository serves as a centralized hub for all metadata information, making it easily accessible for data engineers, analysts, and other stakeholders involved in the data pipeline.
Comparing the Tools: Great Expectations vs. Marquez
Ease of Use: Great Expectations vs. Marquez
When it comes to ease of use, Great Expectations offers a more user-friendly experience due to its simple and intuitive interface. Its code-driven approach allows users to easily define and update data expectations using familiar programming languages. This means that data engineers and analysts can quickly get up to speed and start using Great Expectations without a steep learning curve.
On the other hand, Marquez may require a steeper learning curve, particularly for users not familiar with metadata services. However, once mastered, Marquez provides a powerful and unified view of data lineage and metadata. This can be immensely valuable for organizations that need a comprehensive understanding of their data and its origins.
Scalability: Great Expectations vs. Marquez
Both Great Expectations and Marquez offer scalability, but their approaches are fundamentally different. Great Expectations excels in scalability by integrating seamlessly into existing data pipelines, enabling users to manage large-scale data processing effortlessly. This means that as your data needs grow, Great Expectations can scale with you, ensuring that your data expectations are consistently met.
On the other hand, Marquez focuses on scalability in terms of the number of data sources and jobs it can handle. With its ability to handle complex data ecosystems, Marquez is a robust choice for organizations dealing with diverse data sources. Whether you have multiple data pipelines or a variety of data platforms, Marquez can handle the scale and complexity of your data ecosystem.
Integration Capabilities: Great Expectations vs. Marquez
The integration capabilities of both tools are crucial for their successful adoption within data ecosystems. Great Expectations boasts a wide range of integrations with popular data platforms and frameworks, making it highly versatile and adaptable. This means that you can seamlessly incorporate Great Expectations into your existing data infrastructure without any major disruptions.
On the other hand, Marquez integrates seamlessly with other tools in the data ecosystem and offers REST APIs for easy integration. This enables users to leverage existing infrastructure investments while enhancing their data observability capabilities. By integrating Marquez with your existing tools and platforms, you can gain a holistic view of your data lineage and metadata, making it easier to track and understand the flow of data throughout your organization.
Pros and Cons of Great Expectations
Advantages of Great Expectations
Great Expectations offers numerous advantages that contribute to its popularity among data professionals. One significant advantage is its code-driven approach, which provides a familiar and flexible environment for defining and managing data expectations. Additionally, Great Expectations promotes collaboration by allowing multiple stakeholders to contribute to the data quality assurance process. Moreover, its active community and extensive documentation make it easier for users to troubleshoot issues and seek help.
Disadvantages of Great Expectations
Despite its many advantages, Great Expectations does have a few limitations. One limitation is the learning curve associated with understanding and utilizing the tool's extensive features and functionalities effectively. Additionally, as an open-source tool, the responsibility for maintaining and updating the tool falls on the users and the community. However, the vibrant Great Expectations community helps mitigate this concern by providing ongoing support and frequent updates.
In conclusion, both Great Expectations and Marquez offer powerful data observability capabilities, but their approaches and functionalities differ. Great Expectations excels in flexibility, ease of use, and seamless integration, making it an attractive choice for organizations seeking a code-centric solution. On the other hand, Marquez stands out in its ability to provide a unified view of data lineage and enable metadata management across diverse data ecosystems. Ultimately, the choice between these tools depends on your specific needs and requirements. By understanding the features, functionalities, and trade-offs of Great Expectations and Marquez, you can make an informed decision to ensure the observability and reliability of your data.As you consider the strengths and trade-offs between Great Expectations and Marquez for your data observability needs, it's worth exploring how CastorDoc can further enhance your data management strategy. CastorDoc integrates advanced governance, cataloging, and lineage capabilities with a user-friendly AI assistant, offering a powerful tool for businesses to enable self-service analytics. With its robust data catalog and conversational AI, CastorDoc empowers data teams and business users alike to navigate the complexities of data governance with ease, ensuring data quality and compliance throughout the data lifecycle. Whether you're looking to streamline your data operations or democratize data access across your organization, check out more tools comparisons here and discover how CastorDoc can revolutionize your approach to data observability and governance.
You might also like
Get in Touch to Learn More
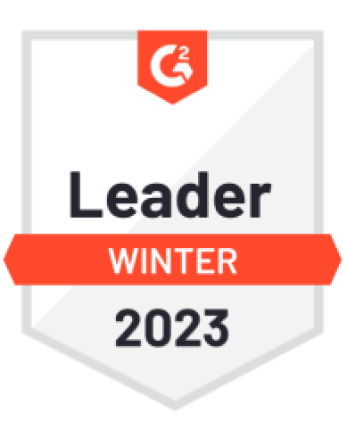
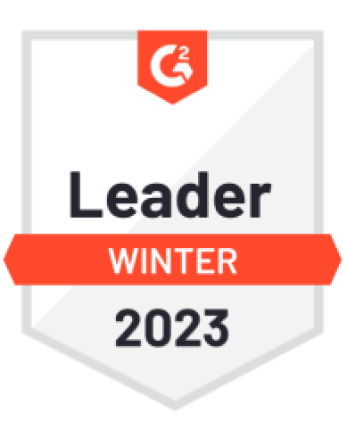

“[I like] The easy to use interface and the speed of finding the relevant assets that you're looking for in your database. I also really enjoy the score given to each table, [which] lets you prioritize the results of your queries by how often certain data is used.” - Michal P., Head of Data